Classification of Tumor in Brain MR Images Using Deep Convolutional Neural Network and Global Average Pooling
PROCESSES(2023)
摘要
Brain tumors can cause serious health complications and lead to death if not detected accurately. Therefore, early-stage detection of brain tumors and accurate classification of types of brain tumors play a major role in diagnosis. Recently, deep convolutional neural network (DCNN) based approaches using brain magnetic resonance imaging (MRI) images have shown excellent performance in detection and classification tasks. However, the accuracy of DCNN architectures depends on the training of data samples since it requires more precise data for better output. Thus, we propose a transfer learning-based DCNN framework to classify brain tumors for example meningioma tumors, glioma tumors, and pituitary tumors. We use a pre-trained DCNN architecture VGGNet which is previously trained on huge datasets and used to transfer its learning parameters to the target dataset. Also, we employ transfer learning aspects such as fine-tune the convolutional network and freeze the layers of the convolutional network for better performance. Further, this proposed approach uses a Global Average Pooling (GAP) layer at the output to avoid overfitting issues and vanishing gradient problems. The proposed architecture is assessed and compared with competing deep learning based brain tumor classification approaches on the Figshare dataset. Our proposed approach produces 98.93% testing accuracy and outperforms the contemporary learning-based approaches.
更多查看译文
关键词
medical imaging,magnetic resonance imaging,deep learning,transfer learning,tumor detection,global average pooling
AI 理解论文
溯源树
样例
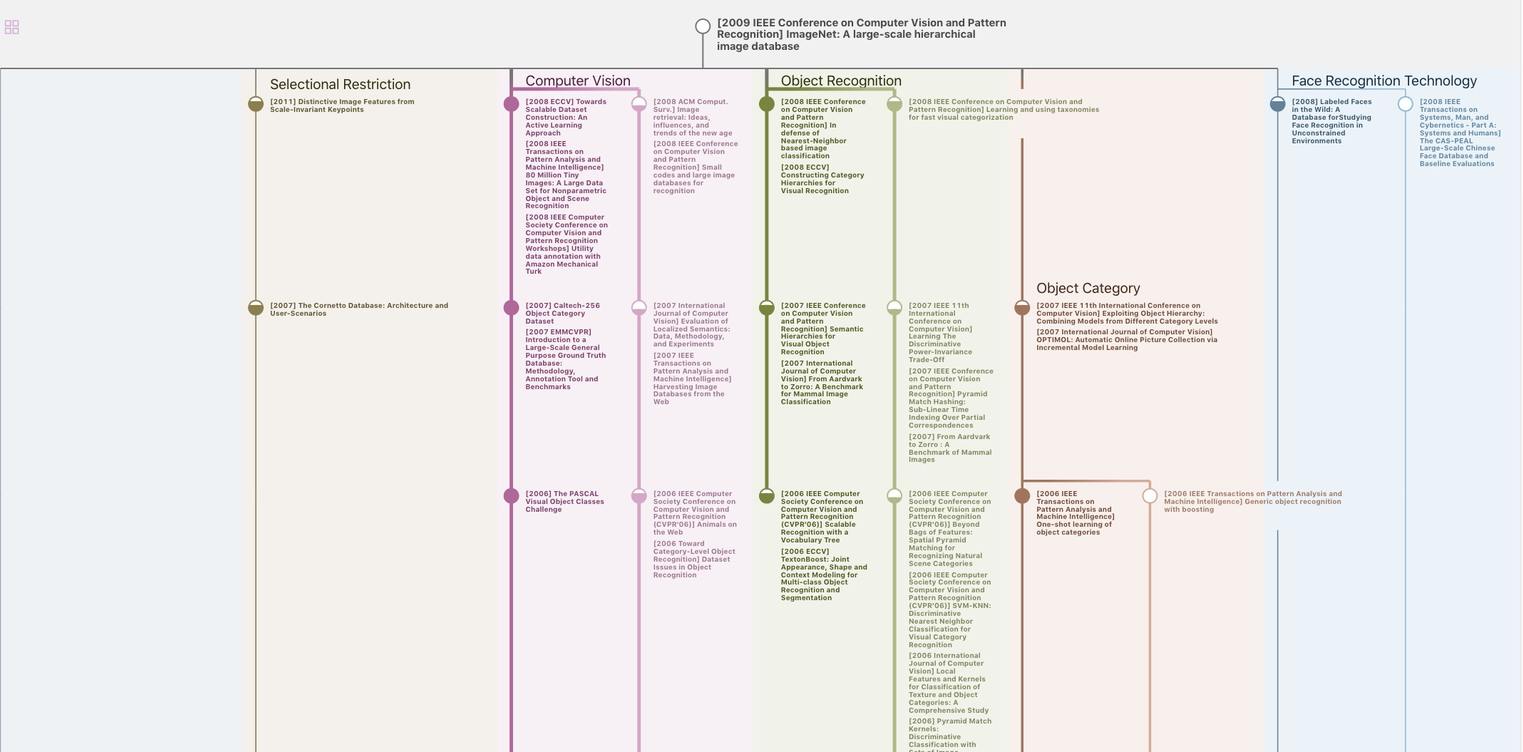
生成溯源树,研究论文发展脉络
Chat Paper
正在生成论文摘要