Cell-attribute aware community detection improves differential abundance testing from single-cell RNA-Seq data
Nature Communications(2023)
摘要
Variations of cell-type proportions within tissues could be informative of biological aging and disease risk. Single-cell RNA-sequencing offers the opportunity to detect such differential abundance patterns, yet this task can be statistically challenging due to the noise in single-cell data, inter-sample variability and because such patterns are often of small effect size. Here we present a differential abundance testing paradigm called ELVAR that uses cell attribute aware clustering when inferring differentially enriched communities within the single-cell manifold. Using simulated and real single-cell and single-nucleus RNA-Seq datasets, we benchmark ELVAR against an analogous algorithm that uses Louvain for clustering, as well as local neighborhood-based methods, demonstrating that ELVAR improves the sensitivity to detect cell-type composition shifts in relation to aging, precancerous states and Covid-19 phenotypes. In effect, leveraging cell attribute information when inferring cell communities can denoise single-cell data, avoid the need for batch correction and help retrieve more robust cell states for subsequent differential abundance testing. ELVAR is available as an open-source R-package.
更多查看译文
关键词
differential abundance testing,aware community detection,cell-attribute,single-cell,rna-seq
AI 理解论文
溯源树
样例
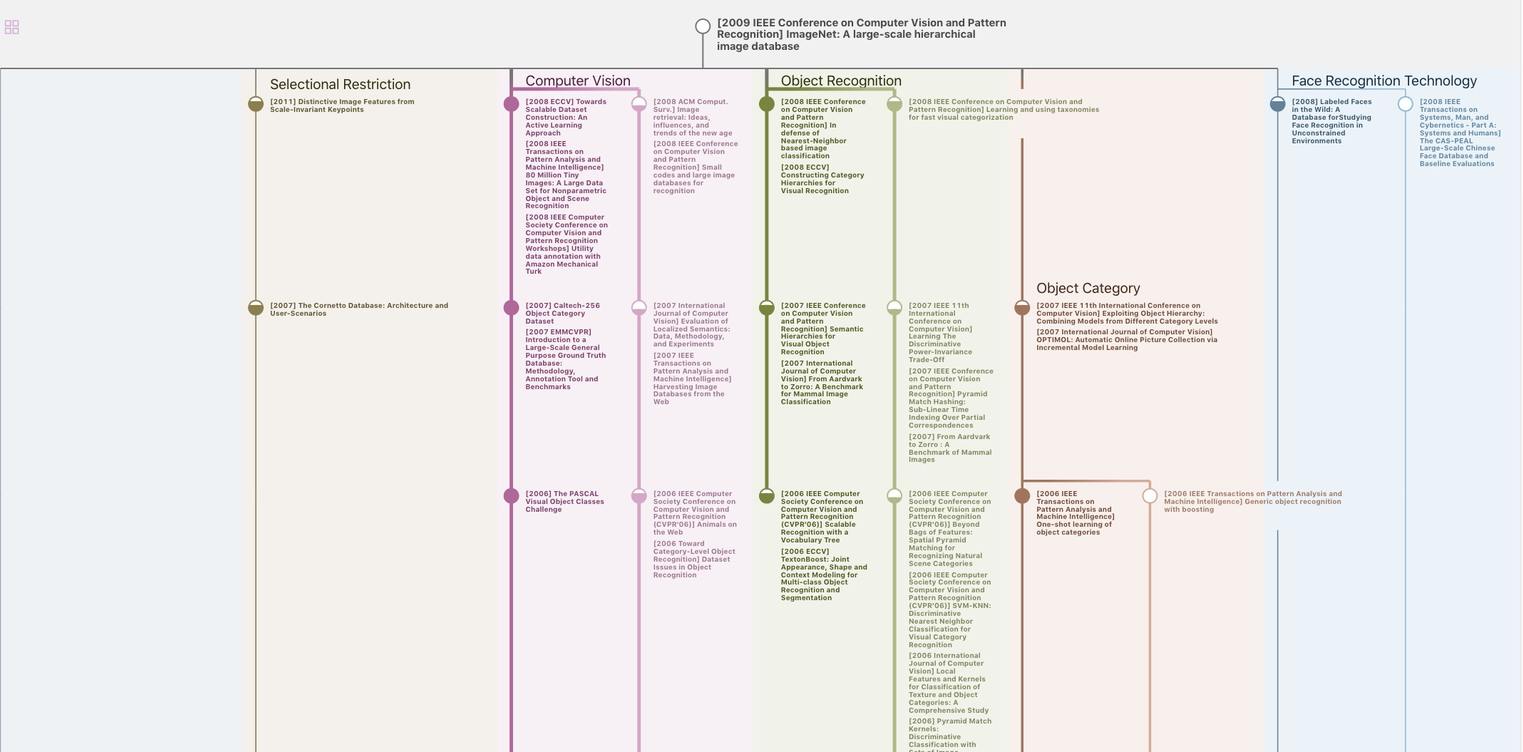
生成溯源树,研究论文发展脉络
Chat Paper
正在生成论文摘要