Modeling Viral Information Spreading via Directed Acyclic Graph Diffusion
GLOBECOM 2023 - 2023 IEEE Global Communications Conference(2023)
摘要
Viral information like rumors or fake news is spread over a communication network like a virus infection in a unidirectional manner: entity $i$ conveys information to a neighbor $j$, resulting in two equally informed (infected) parties. Existing graph diffusion works focus only on bidirectional diffusion on an undirected graph. Instead, we propose a new directed acyclic graph (DAG) diffusion model to estimate the probability $x_i(t)$ of node $i$'s infection at time $t$ given a source node $s$, where $x_i(\infty)~=~1$. Specifically, given an undirected positive graph modeling node-to-node communication, we first compute its graph embedding: a latent coordinate for each node in an assumed low-dimensional manifold space from extreme eigenvectors via LOBPCG. Next, we construct a DAG based on Euclidean distances between latent coordinates. Spectrally, we prove that the asymmetric DAG Laplacian matrix contains real non-negative eigenvalues, and that the DAG diffusion converges to the all-infection vector $\x(\infty) = \1$ as $t \rightarrow \infty$. Simulation experiments show that our proposed DAG diffusion accurately models viral information spreading over a variety of graph structures at different time instants.
更多查看译文
关键词
Graph diffusion,graph signal processing,graph embedding
AI 理解论文
溯源树
样例
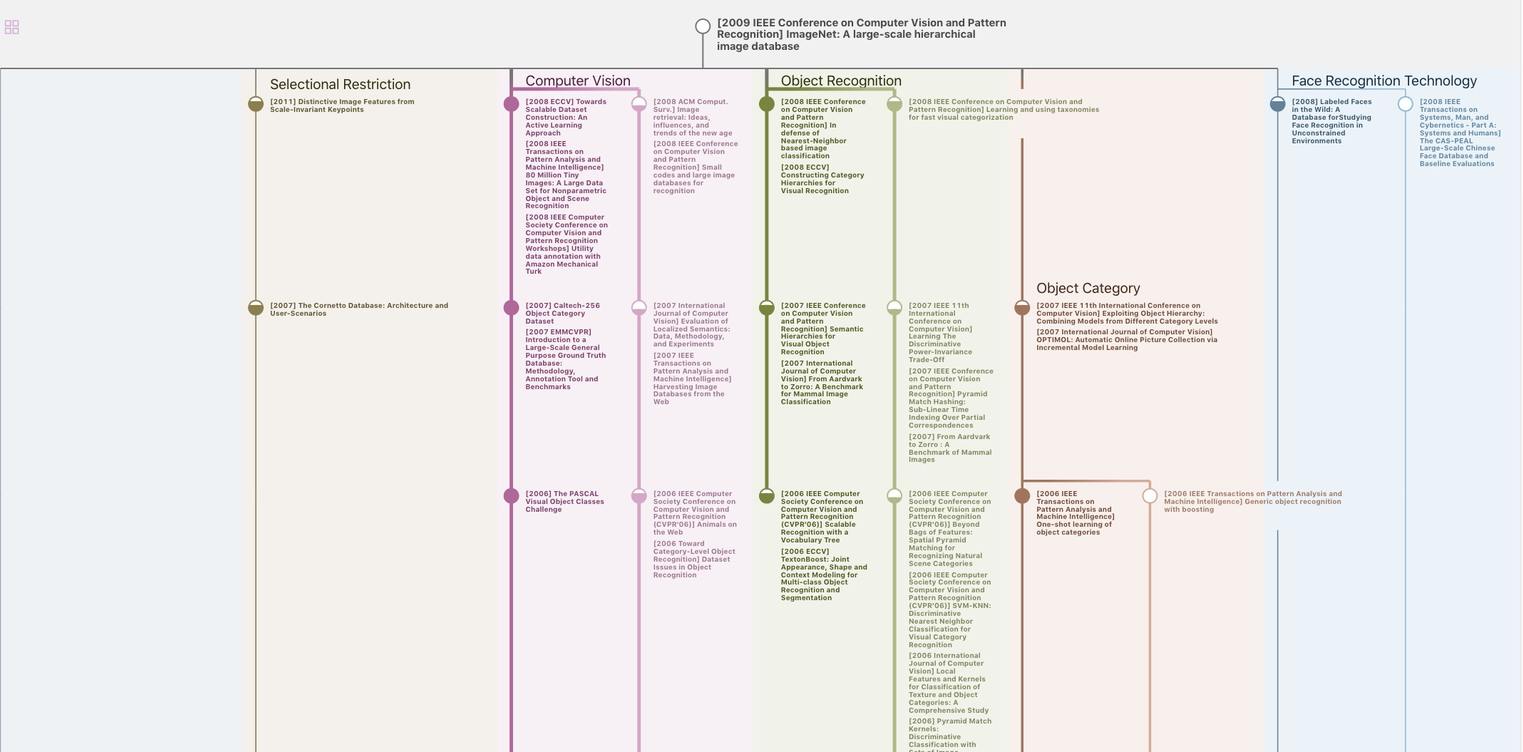
生成溯源树,研究论文发展脉络
Chat Paper
正在生成论文摘要