Improving Image-Based Precision Medicine with Uncertainty-Aware Causal Models
CoRR(2023)
摘要
Image-based precision medicine aims to personalize treatment decisions based on an individual's unique imaging features so as to improve their clinical outcome. Machine learning frameworks that integrate uncertainty estimation as part of their treatment recommendations would be safer and more reliable. However, little work has been done in adapting uncertainty estimation techniques and validation metrics for precision medicine. In this paper, we use Bayesian deep learning for estimating the posterior distribution over factual and counterfactual outcomes on several treatments. This allows for estimating the uncertainty for each treatment option and for the individual treatment effects (ITE) between any two treatments. We train and evaluate this model to predict future new and enlarging T2 lesion counts on a large, multi-center dataset of MR brain images of patients with multiple sclerosis, exposed to several treatments during randomized controlled trials. We evaluate the correlation of the uncertainty estimate with the factual error, and, given the lack of ground truth counterfactual outcomes, demonstrate how uncertainty for the ITE prediction relates to bounds on the ITE error. Lastly, we demonstrate how knowledge of uncertainty could modify clinical decision-making to improve individual patient and clinical trial outcomes.
更多查看译文
关键词
precision medicine,causal
AI 理解论文
溯源树
样例
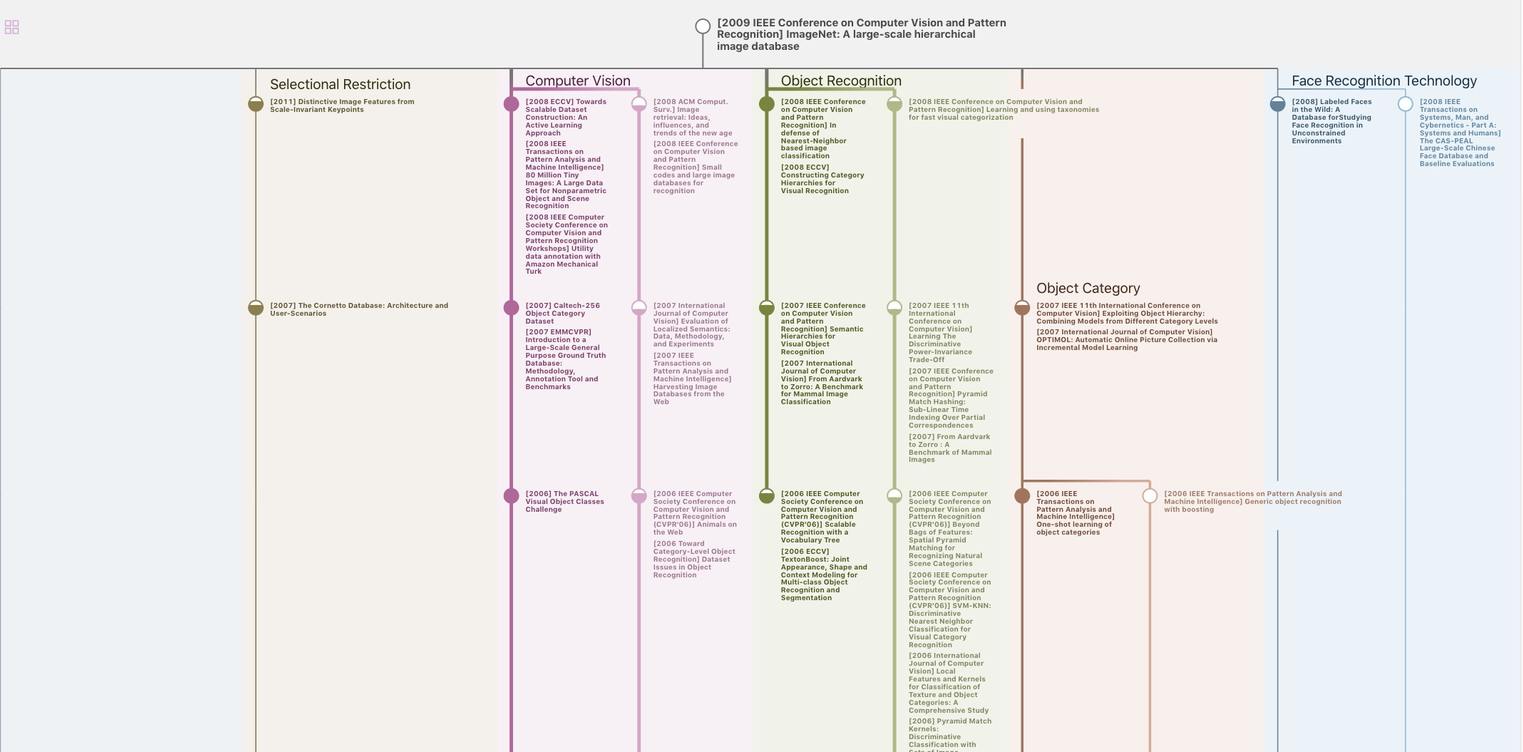
生成溯源树,研究论文发展脉络
Chat Paper
正在生成论文摘要