Zelda: Video Analytics using Vision-Language Models
CoRR(2023)
摘要
Advances in ML have motivated the design of video analytics systems that allow for structured queries over video datasets. However, existing systems limit query expressivity, require users to specify an ML model per predicate, rely on complex optimizations that trade off accuracy for performance, and return large amounts of redundant and low-quality results. This paper focuses on the recently developed Vision-Language Models (VLMs) that allow users to query images using natural language like "cars during daytime at traffic intersections." Through an in-depth analysis, we show VLMs address three limitations of current video analytics systems: general expressivity, a single general purpose model to query many predicates, and are both simple and fast. However, VLMs still return large numbers of redundant and low-quality results, which can overwhelm and burden users. We present Zelda: a video analytics system that uses VLMs to return both relevant and semantically diverse results for top-K queries on large video datasets. Zelda prompts the VLM with the user's query in natural language and additional terms to improve accuracy and identify low-quality frames. Zelda improves result diversity by leveraging the rich semantic information encoded in VLM embeddings. We evaluate Zelda across five datasets and 19 queries and quantitatively show it achieves higher mean average precision (up to 1.15$\times$) and improves average pairwise similarity (up to 1.16$\times$) compared to using VLMs out-of-the-box. We also compare Zelda to a state-of-the-art video analytics engine and show that Zelda retrieves results 7.5$\times$ (up to 10.4$\times$) faster for the same accuracy and frame diversity.
更多查看译文
关键词
video analytics,vision-language
AI 理解论文
溯源树
样例
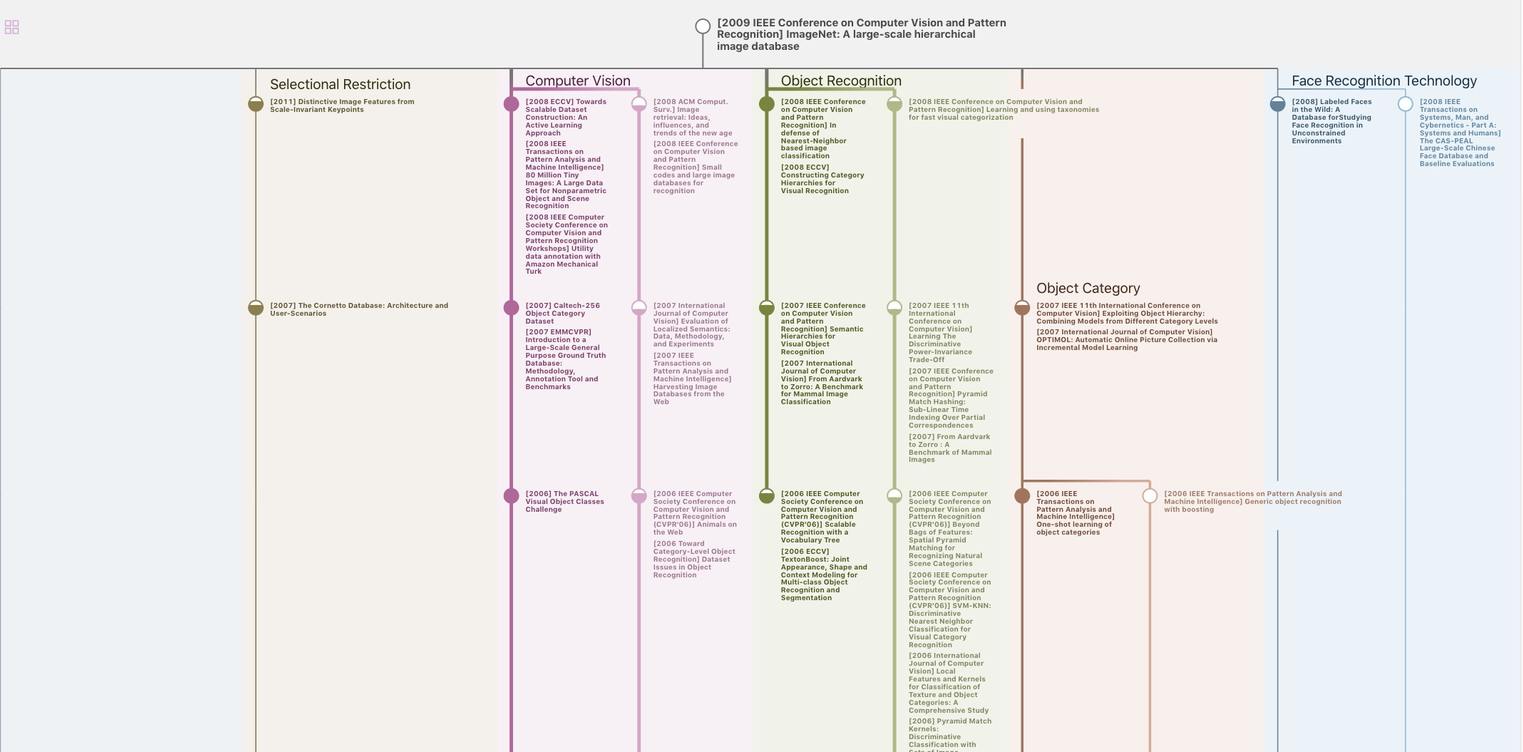
生成溯源树,研究论文发展脉络
Chat Paper
正在生成论文摘要