CWIWD-IPS: A Crowdsensing/Walk-Surveying Inertial/Wi-Fi Data-Driven Indoor Positioning System
IEEE Internet of Things Journal(2023)
摘要
Indoor positioning system plays a key role in location-based services since the widely used global navigation satellite system (GNSS) is denied in indoor scenarios. Crowdsensing or walking-surveying-based indoor positioning is proposed aiming at providing low-cost and high-efficient 3-D location. This article proposes a crowdsensing/walking-surveying 3-D indoor positioning system by fusing the crowdsensed inertial data and Wi-Fi fingerprinting samples using deep learning frameworks. A sine-wave-based step detector is used for pedestrian dead-reckoning (PDR) to generate original dense-trajectories. An enhanced optimization-based algorithm (Opt) and a smoothing-based algorithm (Smo) are proposed and evaluated to correct the original dense-trajectories into near-true dense-trajectories which are used to construct the inertial database and Wi-Fi radio map. A ResNet-based inertial neural network and a BiLSTM-based Wi-Fi fingerprinting neural network are trained on the constructed navigation database and combined by a Kalman filter to provide accurate and robust 3-D localization performance. The realistic experimental results among complex indoor environments demonstrate that the proposed algorithms are proved to achieve a precise 3-D indoor localization performance which is superior to several existing relative methods.
更多查看译文
关键词
Crowdsensing/walking-surveying,data driven,deep learning,neural network,pedestrian dead-reckoning (PDR),Wi-Fi fingerprinting
AI 理解论文
溯源树
样例
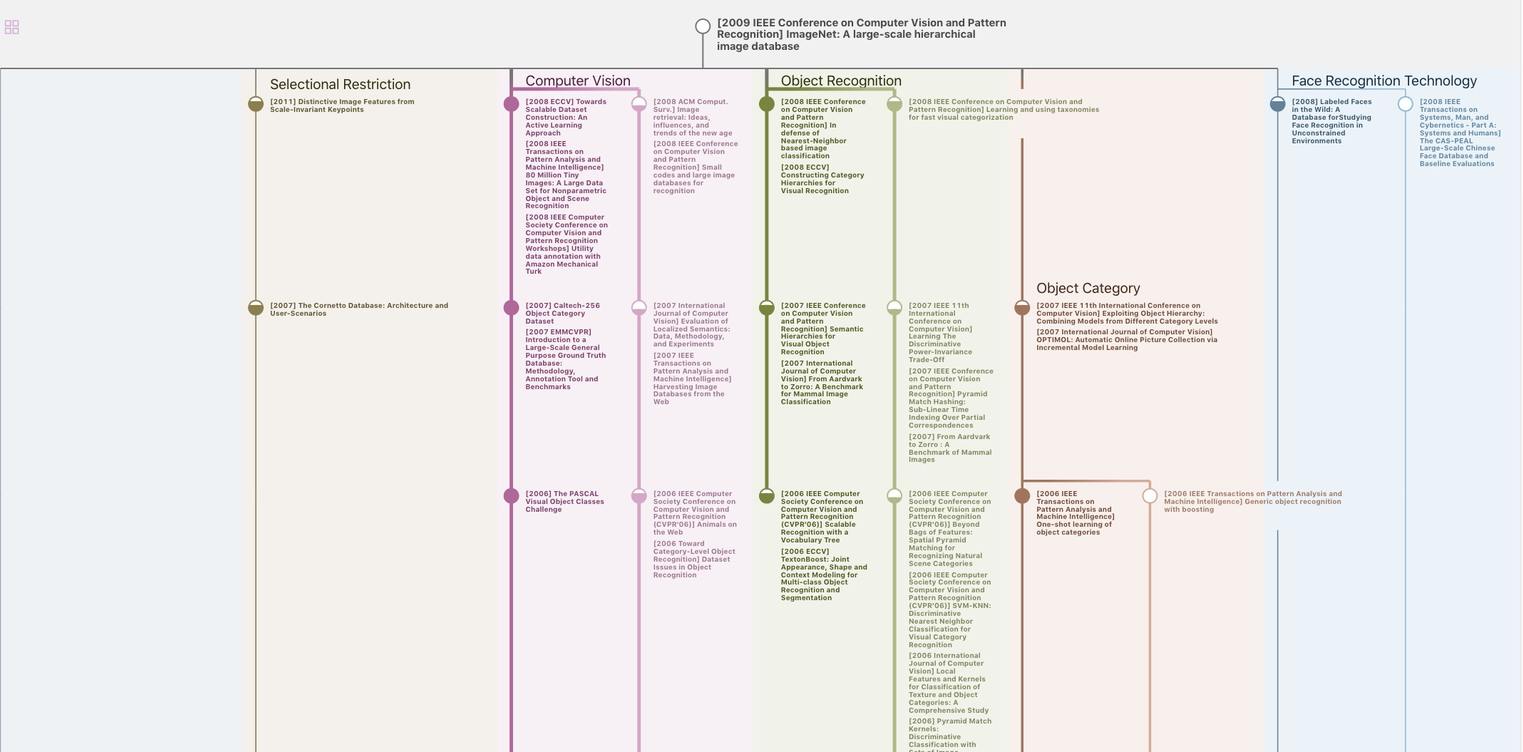
生成溯源树,研究论文发展脉络
Chat Paper
正在生成论文摘要