Detection of Arterial Hypertension Through Electrocardiograms.
CinC(2022)
摘要
Comorbidities' awareness is relevant to evaluate patients' conditions. However, very often, the main source of such information is patient self-report, which lacks reliability. Arterial Hypertension (AH) is a very relevant comorbidity that may change prognosis for several diseases. Recent studies use electrocardiogram (ECG) to diagnose and even identify relevant patient information, such as gender and age. There is also evidence that it is possible to extract patient comorbidities using the raw ECG signal. Our goal in this work is to identify AH using the 12 lead ECG signal. We use a deep neural network model tailored for learning latent features from ECG signal raw data (i. e., end-to-end approach). Our model achieved 0.89 sensibility (Recall), 0.61 positive predictive value (Precision), with an overall F1-score of 0.72. These results are significant in practice, in particular the high sensibility, considering the low prevalence of AH on patients. Despite its relatively low precision, its results are better than those associated with patient self-report, making it a potentially useful resource for physicians, mainly in telehealth practice.
更多查看译文
关键词
0.61 positive predictive value,12 lead ECG signal,AH,arterial Hypertension,Arterial Hypertension,deep neural network model,ECG signal raw data,electrocardiograms,end-to-end approach,gender,latent features,particular the high sensibility,patient comorbidities,patient self-report,patients,raw ECG signal,relatively low precision,relevant comorbidity,relevant patient information
AI 理解论文
溯源树
样例
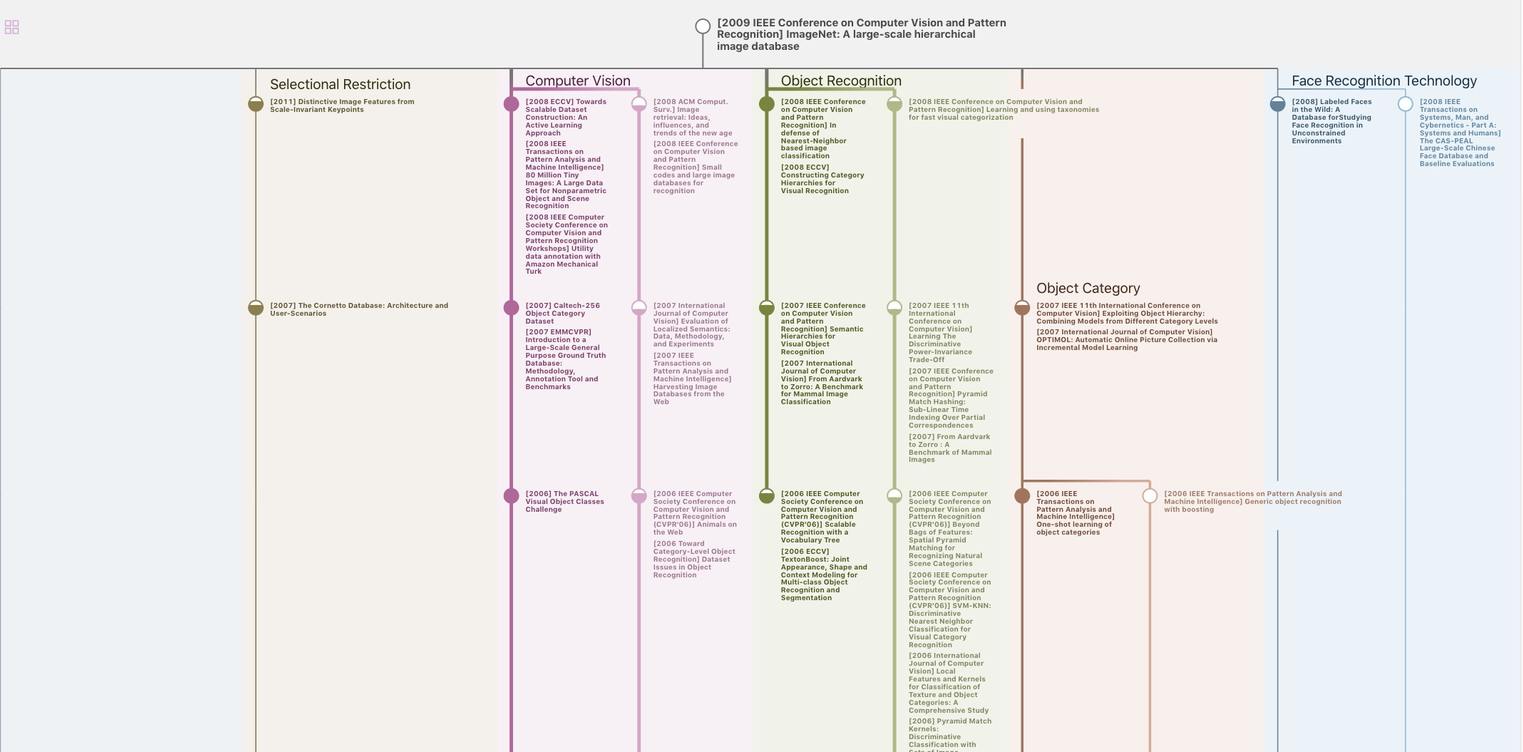
生成溯源树,研究论文发展脉络
Chat Paper
正在生成论文摘要