Anonymous Edge Representation for Inductive Anomaly Detection in Dynamic Bipartite Graphs.
Proc. VLDB Endow.(2023)
摘要
The activities in many real-world applications, such as e-commerce and online education, are usually modeled as a dynamic bipartite graph that evolves over time. It is a critical task to detect anomalies inductively in a dynamic bipartite graph. Previous approaches either focus on detecting pre-defined types of anomalies or cannot handle nodes that are unseen during the training stage. To address this challenge, we propose an effective method to learn anonymous edge representation (AER) that captures the characteristics of an edge without using identity information. We further propose a model named AER-AD to utilize AER to detect anomalies in dynamic bipartite graphs in an inductive setting. Extensive experiments on both real-life and synthetic datasets are conducted to illustrate that AER-AD outperforms state-of-the-art baselines. In terms of AUC and F1, AER-AD is able to achieve 8.38% and 14.98% higher results than the best inductive representation baselines, and 6.99% and 19.59% than the best anomaly detection baselines.
更多查看译文
关键词
inductive anomaly detection,dynamic bipartite graph,edge
AI 理解论文
溯源树
样例
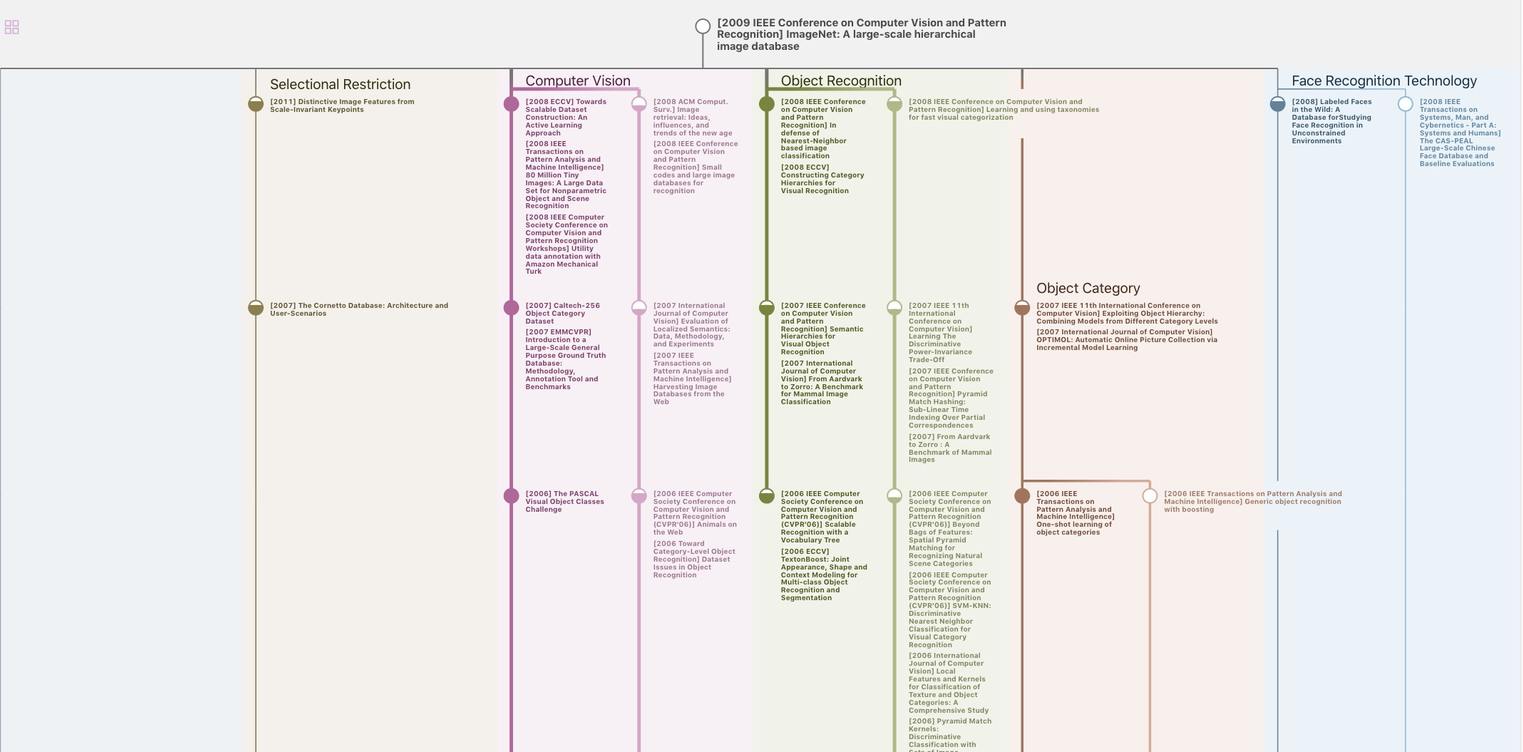
生成溯源树,研究论文发展脉络
Chat Paper
正在生成论文摘要