Quantum average neighborhood margin maximization for feature extraction
Quantum Inf. Process.(2023)
摘要
verage neighborhood margin maximization (ANMM) is a local supervised metric learning approach, which aims to find projection directions where the local class discriminability is maximized. Furthermore, it has no assumption of class distribution and works well on small sample size. In this work, we address this problem in the quantum setting and present a quantum ANMM algorithm for linear feature extraction. More specifically, a quantum algorithm is designed to construct scatterness and compactness matrices in the quantum state form. Then a quantum algorithm is presented to obtain the features of the testing sample set in the quantum state form. The time complexity analysis of the quantum ANMM algorithm shows that our algorithm may achieve an exponential speedup on the dimension of the sample points D , and a quadratic speedup on the numbers of training samples N and testing samples M compared to the classical counterpart under certain conditions.
更多查看译文
关键词
Average neighborhood margin maximization, Quantum average neighborhood margin maximization, Linear feature extraction
AI 理解论文
溯源树
样例
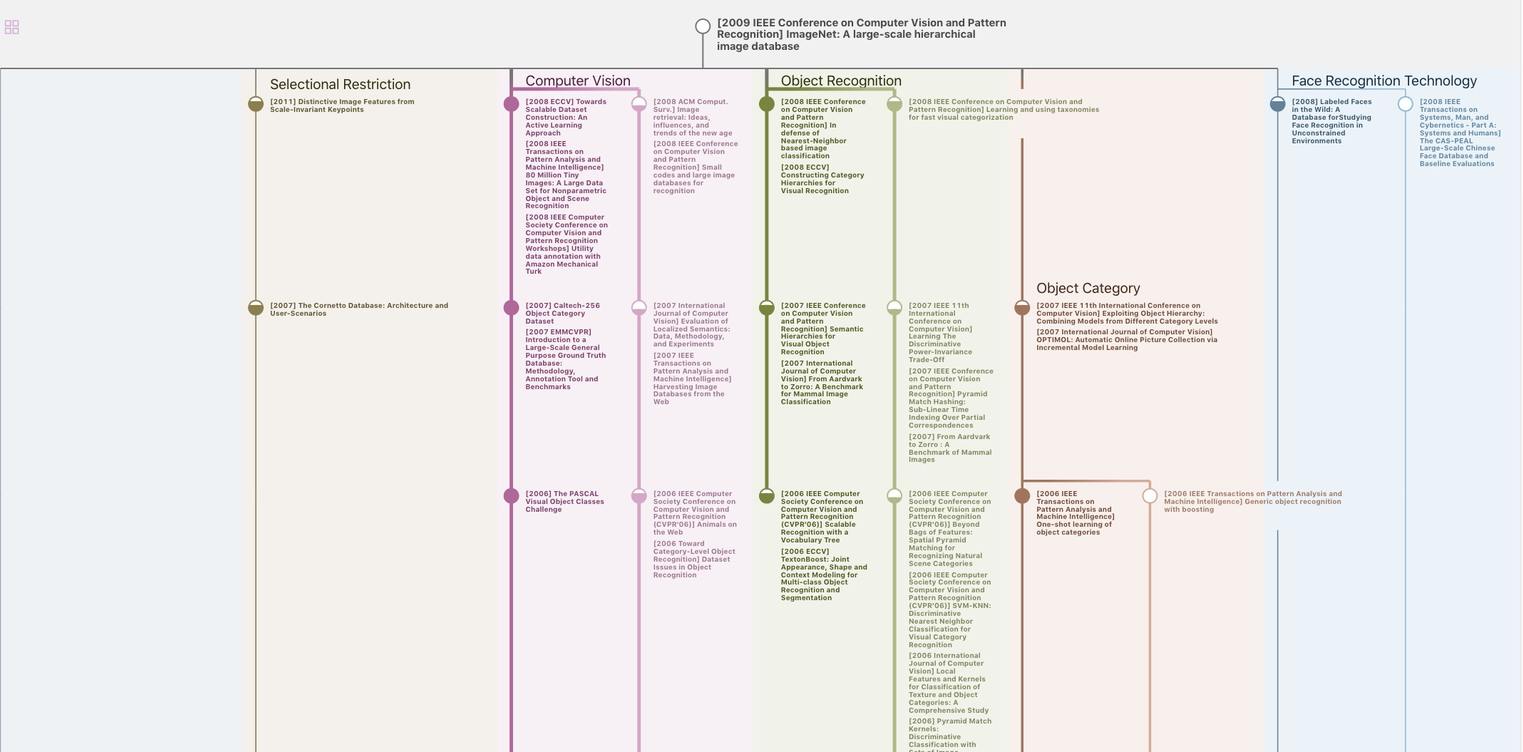
生成溯源树,研究论文发展脉络
Chat Paper
正在生成论文摘要