A semi-supervised short text sentiment classification method based on improved Bert model from unlabelled data
J. Big Data(2023)
摘要
Short text information has considerable commercial value and immeasurable social value. Natural language processing and short text sentiment analysis technology can organize and analyze short text information on the Internet. Natural language processing tasks such as sentiment classification have achieved satisfactory performance under a supervised learning framework. However, traditional supervised learning relies on large-scale and high-quality manual labels and obtaining high-quality label data costs a lot. Therefore, the strong dependence on label data hinders the application of the deep learning model to a large extent, which is the bottleneck of supervised learning. At the same time, short text datasets such as product reviews have an imbalance in the distribution of data samples. To solve the above problems, this paper proposes a method to predict label data according to semi-supervised learning mode and implements the MixMatchNL data enhancement method. Meanwhile, the Bert pre-training model is updated. The cross-entropy loss function in the model is improved to the Focal Loss function to alleviate the data imbalance in short text datasets. Experimental results based on public datasets indicate the proposed model has improved the accuracy of short text sentiment recognition compared with the previous update and other state-of-the-art models.
更多查看译文
关键词
Sentiment analysis,Semi-supervised learning,Data enhancement,Data imbalance,Language models
AI 理解论文
溯源树
样例
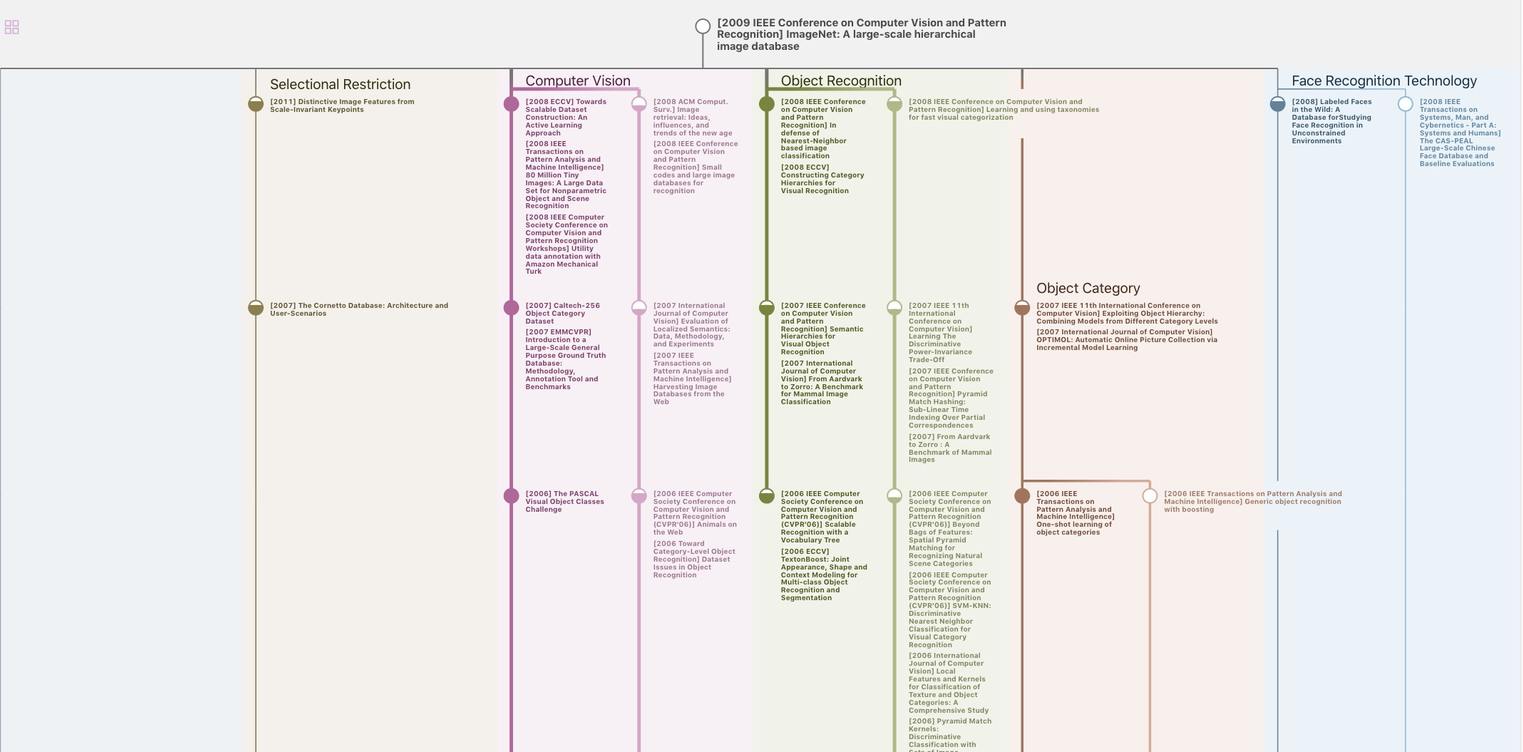
生成溯源树,研究论文发展脉络
Chat Paper
正在生成论文摘要