Distributed synchronization based on model-free reinforcement learning in wireless ad hoc networks.
Comput. Networks(2023)
摘要
Time synchronization is a key issue in wireless ad hoc networks. Due to the dynamic characteristics of such networks, distributed synchronization (DS) is preferred for its reliability and validity. However, one major drawback of this synchronization mechanism is that nodes exchange time synchronization messages with their neighbors, which can be very time-consuming. In order to reduce network synchronization overhead while maintaining synchronization quality, this paper presents a model-free reinforcement learning distributed synchronization (RLDS) by evaluating the current network state and node synchronization level, adaptively deciding that the current node interacts with a certain portion of its neighbors instead of all of them for synchronization information. The simulation results indicated that during the initial network synchronization, RLDS achieves the same synchronization accuracy as the traditional DS, while reducing the total communi-cation overhead by 15%. The superiority of RLDS is more evident in the long-term maintenance of network synchronization, reducing the communication overhead by 48% during 500 rounds of synchronization. This is because the number of node neighbors in communication can be appropriately reduced, thus achieving an adaptive trade-off between ensuring time synchronization and saving communication overhead. This study shows the latent capacity of reinforcement learning in improving the performance of traditional ad hoc networking technologies.
更多查看译文
关键词
synchronization,networks,model-free
AI 理解论文
溯源树
样例
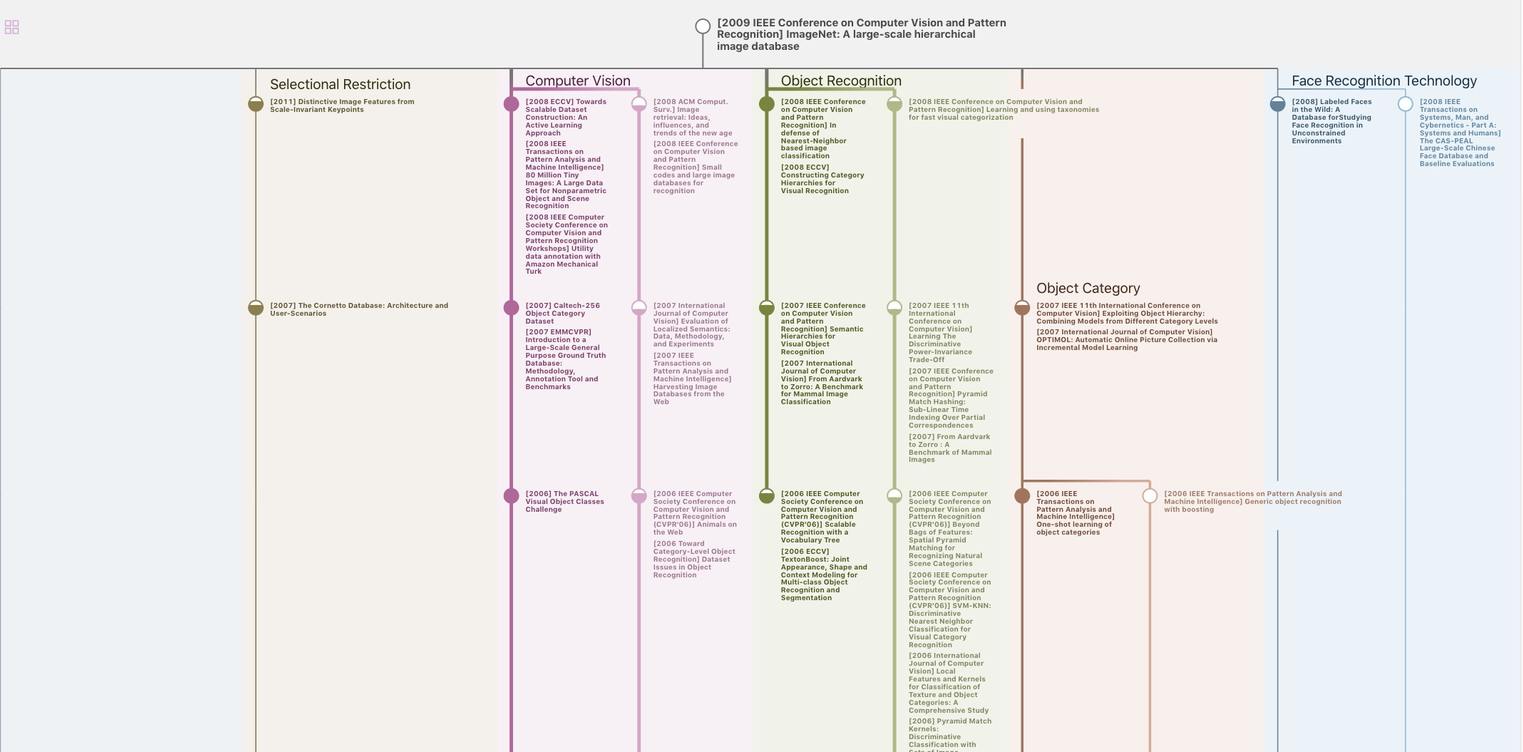
生成溯源树,研究论文发展脉络
Chat Paper
正在生成论文摘要