DebiasedDTA: Model Debiasing to Boost Drug-Target Affinity Prediction.
CoRR(2021)
摘要
Motivation: Computational models that accurately identify high-affinity protein-compound pairs can accelerate drug discovery pipelines. These models aim to learn binding mechanics through drug-target interaction datasets and use the learned knowledge for predicting the affinity of an input protein-compound pair. However, the datasets they rely on bear misleading patterns that bias models towards memorizing dataset-specific biomolecule properties, instead of learning binding mechanics. This results in models that struggle while predicting drug-target affinities (DTA), especially between de novo biomolecules. Here we present DebiasedDTA, the first DTA model debiasing approach that avoids dataset biases in order to boost affinity prediction for novel biomolecules. DebiasedDTA uses ensemble learning and sample weight adaptation for bias identification and avoidance and is applicable to almost all existing DTA prediction models. Results: The results show that DebiasedDTA can boost models while predicting the interactions between novel biomolecules. Known biomolecules also benefit from the performance improvement, especially when the test biomolecules are dissimilar to the training set. The experiments also show that DebiasedDTA can augment DTA prediction models of different input and model structures and is able to avoid biases of different sources. Availability and Implementation: The source code, the models, and the datasets are freely available for download at this https URL, implementation in Python3, and supported for Linux, MacOS and MS Windows. Contact: arzucan.ozgur@boun.this http URL, elif.ozkirimli@roche.com
更多查看译文
关键词
prediction,drug-target
AI 理解论文
溯源树
样例
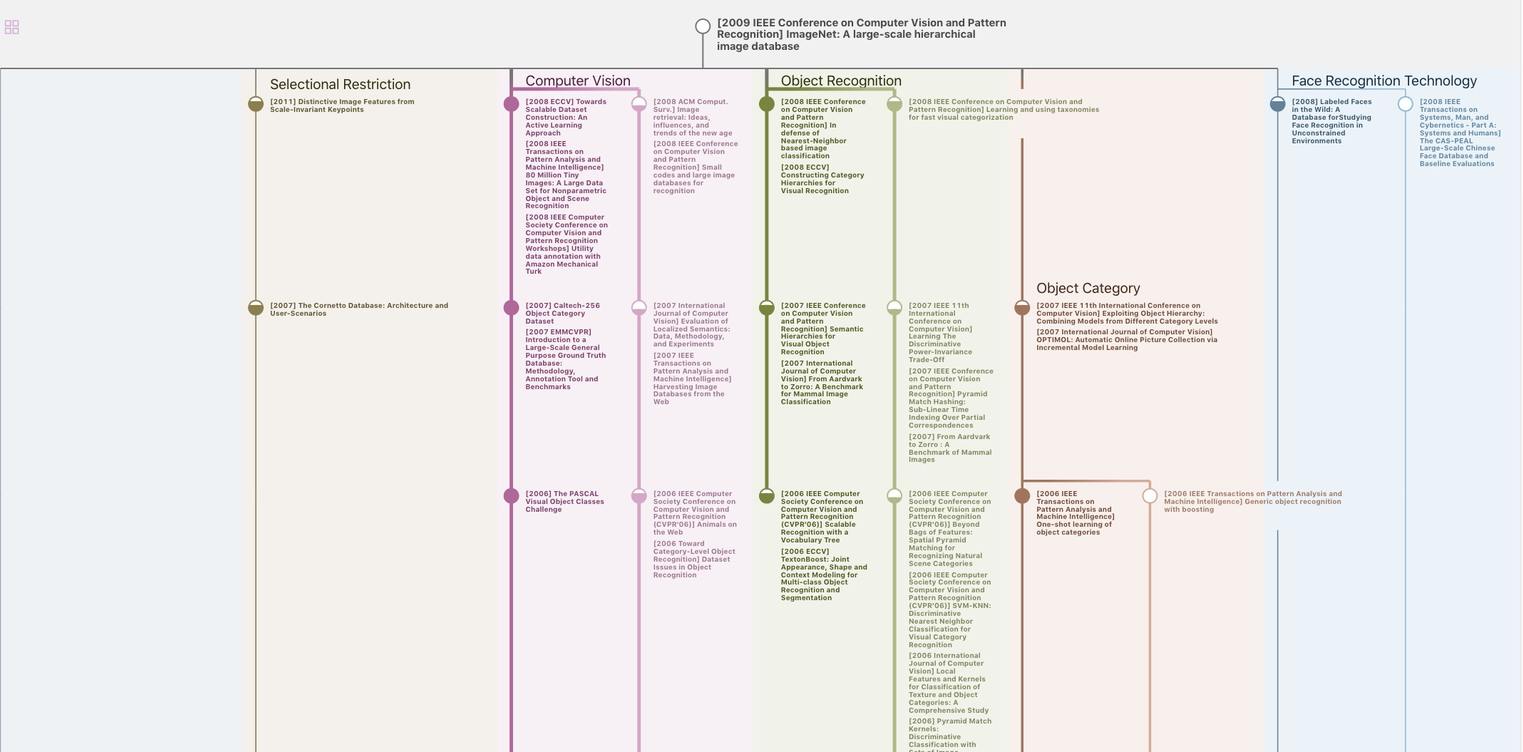
生成溯源树,研究论文发展脉络
Chat Paper
正在生成论文摘要