Sufficient Statistic Memory AMP.
CoRR(2021)
摘要
Approximate message passing (AMP) type algorithms have been widely used in the signal reconstruction of certain large random linear systems. A key feature of the AMP-type algorithms is that their dynamics can be correctly described by state evolution. While state evolution is a useful analytic tool, its convergence is not guaranteed. To solve the convergence problem of the state evolution of AMP-type algorithms in principle, this paper proposes a sufficient-statistic memory AMP (SS-MAMP) algorithm framework under the conditions of right-unitarily invariant sensing matrices, Lipschitz-continuous local processors and the sufficient-statistic constraint (i.e., the current message of each local processor is a sufficient statistic of the signal vector given the current and all preceding messages). We show that the covariance matrices of SS-MAMP are L-banded and convergent, which is an optimal framework (from the local MMSE/LMMSE perspective) for AMP-type algorithms given the Lipschitz-continuous local processors. Given an arbitrary MAMP, we can construct an SS-MAMP by damping, which not only ensures the convergence of the state evolution, but also preserves the orthogonality, i.e., its dynamics can be correctly described by state evolution. As a byproduct, we prove that the Bayes-optimal orthogonal/vector AMP (BO-OAMP/VAMP) is an SS-MAMP. As an example, we construct a sufficient-statistic Bayes-optimal MAMP (SS-BO-MAMP) whose state evolution converges to the minimum (i.e., Bayes-optimal) mean square error (MSE) predicted by replica methods when it has a unique fixed point. In addition, the MSE of SS-BO-MAMP is not worse than the original BO-MAMP. Finally, simulations are provided to support the theoretical results.
更多查看译文
关键词
memory,amp,sufficient-statistic
AI 理解论文
溯源树
样例
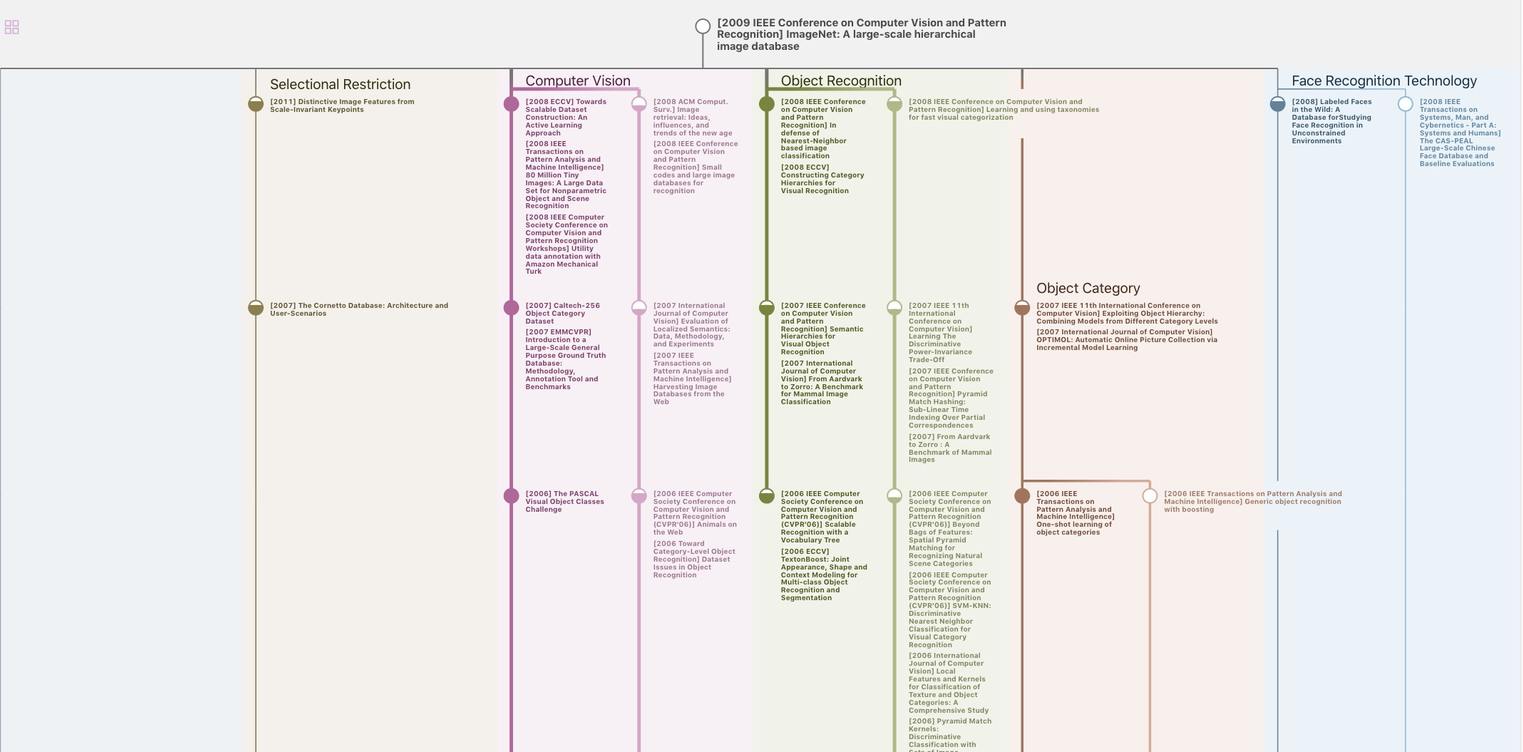
生成溯源树,研究论文发展脉络
Chat Paper
正在生成论文摘要