Energy-Efficient Forecasting of Temperature Data in Sensor Cloud System Using a Hybrid SVM-ANN Method
Wirel. Pers. Commun.(2023)
摘要
In a sensor cloud system, the sensors collect data and send it to the cloud system so that the end-users can access the data. When the frequency of data transmission is high, more power is consumed which reduces the finite battery lifetime of sensors. To reduce the data transmission between sensor and cloud without compromising the frequency of availability of data to end-users, an efficient hybrid forecasting method is developed to forecast the sensor data within the cloud and the end-users get the forecasted data at the same frequency with minimal error. In the proposed hybrid method, the sensor data is first modeled using the support vector machine (SVM) with Gaussian kernel function (SVMG) and SVM with linear kernel function (SVML). The forecasted values of SVMG and SVML are modeled using the artificial neural network to get the true forecasts. To assess the effectiveness of the proposed method, hourly temperature sensor data of Delhi is considered, and the obtained results are compared with the state-of-the-art deep learning, machine learning, ensemble, naïve and hybrid models. In the proposed approach, the sensor communicates with the cloud either every two hours (in case of one-step-ahead forecasting) or six hours (in case of five-step-ahead forecasting), but the user gets the forecasted hourly data from the cloud. Using the proposed approach, the energy consumption is reduced by 50% and 83.56% in comparison to the traditional approach in 1-step-ahead and 5-step-ahead forecasting without compromising much in accuracy.
更多查看译文
关键词
Sensor cloud,Data forecasting,Artificial neural network (ANN),Hybrid model,Support vector machine (SVM)
AI 理解论文
溯源树
样例
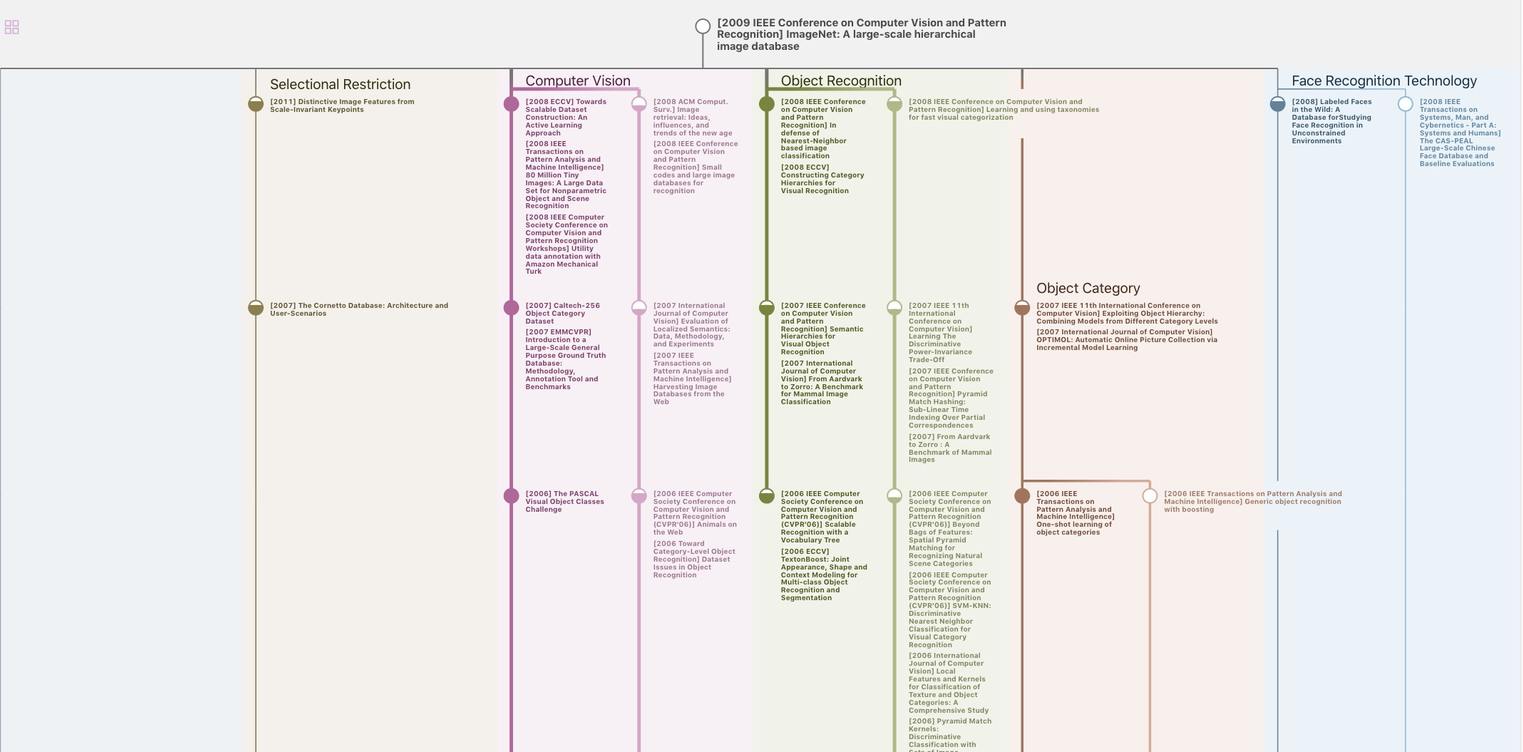
生成溯源树,研究论文发展脉络
Chat Paper
正在生成论文摘要