Self-Supervised Learning for Organs At Risk and Tumor Segmentation with Uncertainty Quantification
CoRR(2023)
摘要
In this study, our goal is to show the impact of self-supervised pre-training of transformers for organ at risk (OAR) and tumor segmentation as compared to costly fully-supervised learning. The proposed algorithm is called Monte Carlo Transformer based U-Net (MC-Swin-U). Unlike many other available models, our approach presents uncertainty quantification with Monte Carlo dropout strategy while generating its voxel-wise prediction. We test and validate the proposed model on both public and one private datasets and evaluate the gross tumor volume (GTV) as well as nearby risky organs' boundaries. We show that self-supervised pre-training approach improves the segmentation scores significantly while providing additional benefits for avoiding large-scale annotation costs.
更多查看译文
关键词
tumor segmentation,learning,uncertainty quantification,organs,self-supervised
AI 理解论文
溯源树
样例
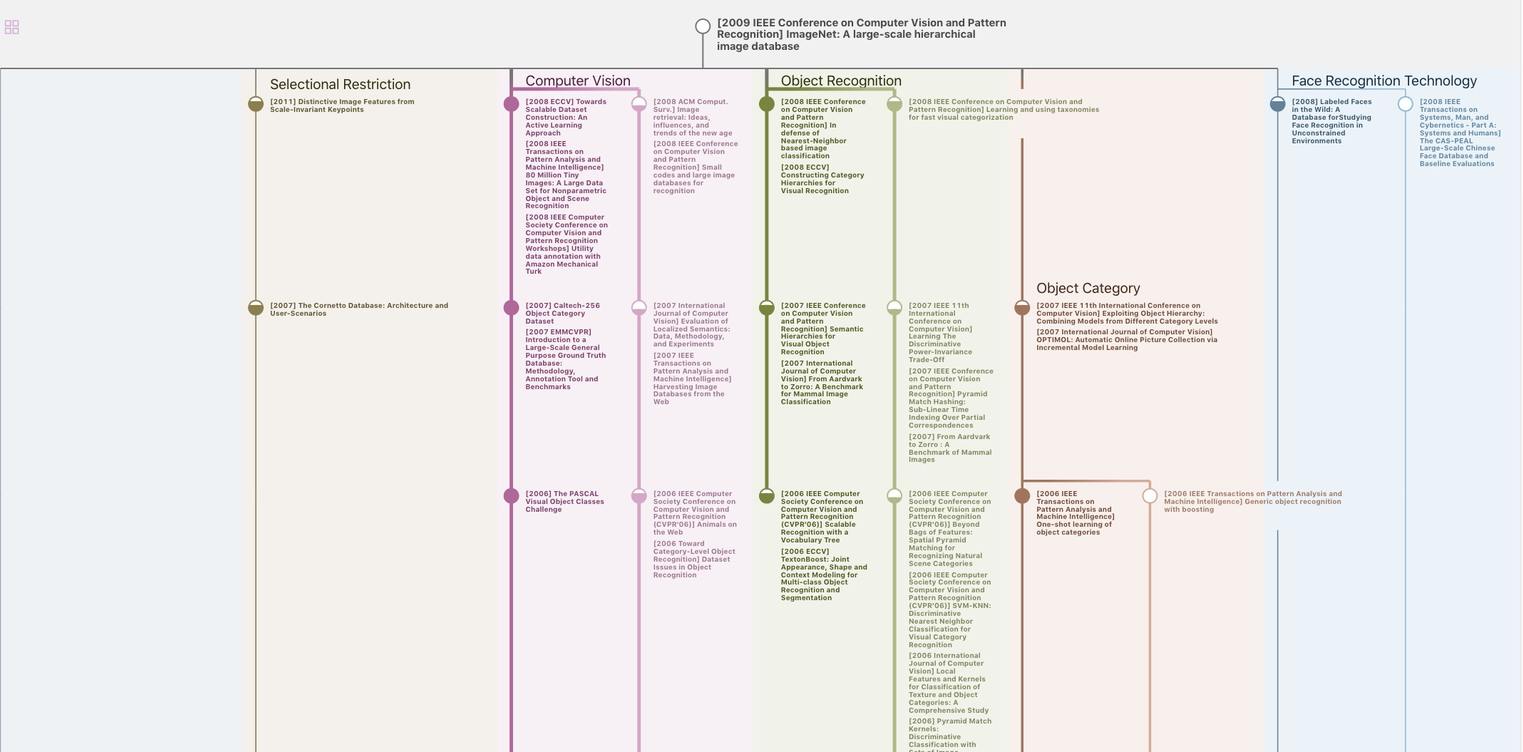
生成溯源树,研究论文发展脉络
Chat Paper
正在生成论文摘要