Pay More Attention to Relation Exploration for Knowledge Base Question Answering
conf_acl(2023)
摘要
Knowledge base question answering (KBQA) is a challenging task that aims to retrieve correct answers from large-scale knowledge bases. Existing attempts primarily focus on entity representation and final answer reasoning, which results in limited supervision for this task. Moreover, the relations, which empirically determine the reasoning path selection, are not fully considered in recent advancements. In this study, we propose a novel framework, RE-KBQA, that utilizes relations in the knowledge base to enhance entity representation and introduce additional supervision. We explore guidance from relations in three aspects, including (1) distinguishing similar entities by employing a variational graph auto-encoder to learn relation importance; (2) exploring extra supervision by predicting relation distributions as soft labels with a multi-task scheme; (3) designing a relation-guided re-ranking algorithm for post-processing. Experimental results on two benchmark datasets demonstrate the effectiveness and superiority of our framework, improving the F1 score by 5.7% from 40.5 to 46.3 on CWQ and 5.8% from 62.8 to 68.5 on WebQSP, better or on par with state-of-the-art methods.
更多查看译文
关键词
relation exploration,knowledge base question
AI 理解论文
溯源树
样例
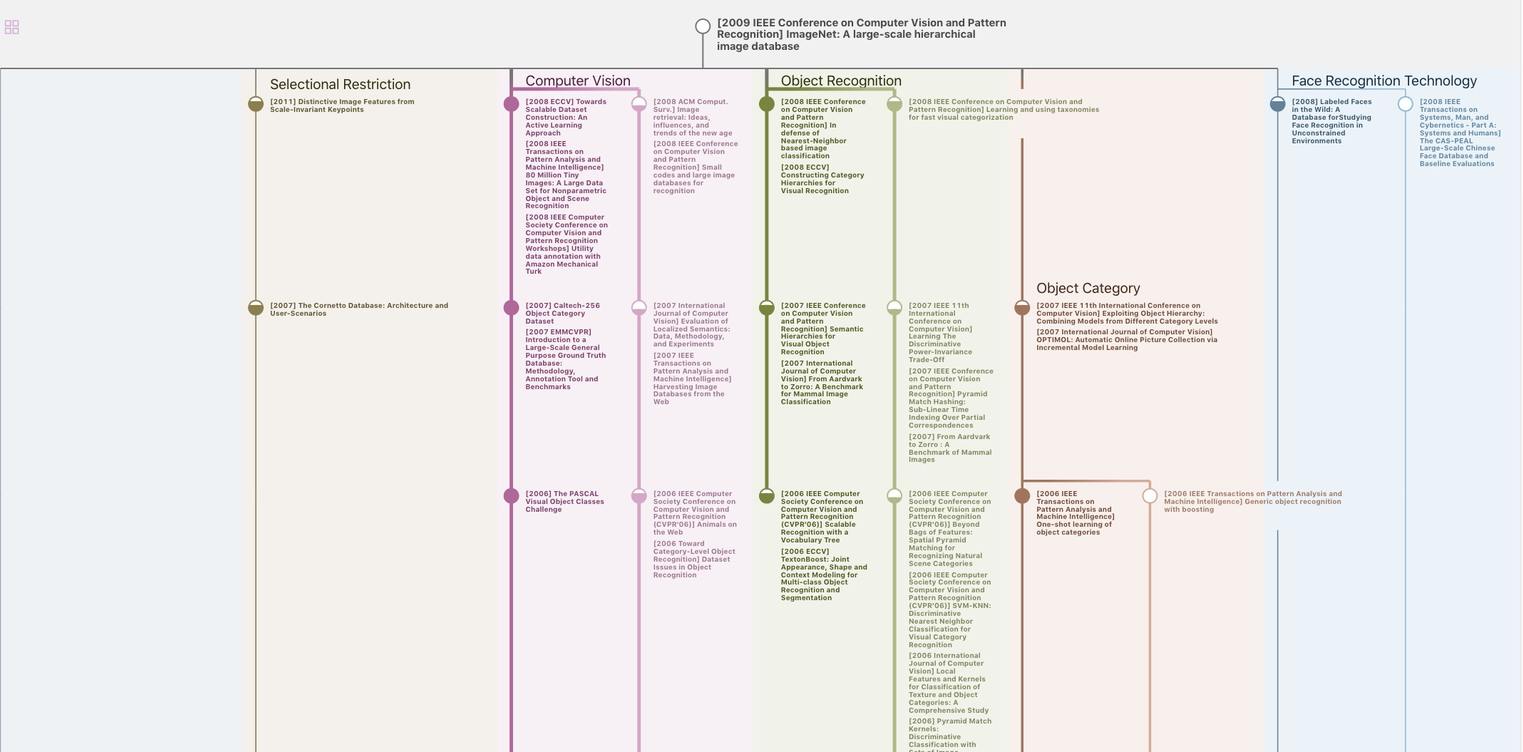
生成溯源树,研究论文发展脉络
Chat Paper
正在生成论文摘要