Robustified Learning for Online Optimization with Memory Costs
CoRR(2023)
摘要
Online optimization with memory costs has many real-world applications, where sequential actions are made without knowing the future input. Nonetheless, the memory cost couples the actions over time, adding substantial challenges. Conventionally, this problem has been approached by various expert-designed online algorithms with the goal of achieving bounded worst-case competitive ratios, but the resulting average performance is often unsatisfactory. On the other hand, emerging machine learning (ML) based optimizers can improve the average performance, but suffer from the lack of worst-case performance robustness. In this paper, we propose a novel expert-robustified learning (ERL) approach, achieving both good average performance and robustness. More concretely, for robustness, ERL introduces a novel projection operator that robustifies ML actions by utilizing an expert online algorithm; for average performance, ERL trains the ML optimizer based on a recurrent architecture by explicitly considering downstream expert robustification. We prove that, for any λ ≥ 1, ERL can achieve λ-competitive against the expert algorithm and λ•C-competitive against the optimal offline algorithm (where C is the expert’s competitive ratio). Additionally, we extend our analysis to a novel setting of multi-step memory costs. Finally, our analysis is supported by empirical experiments for an energy scheduling application.
更多查看译文
关键词
bounded worst-case competitive ratios,downstream expert robustification,energy scheduling application,ERL,expert-designed online algorithms,expert-robustified learning approach,machine learning based optimizers,ML optimizer,multistep memory costs,online optimization,optimal offline algorithm,sequential actions
AI 理解论文
溯源树
样例
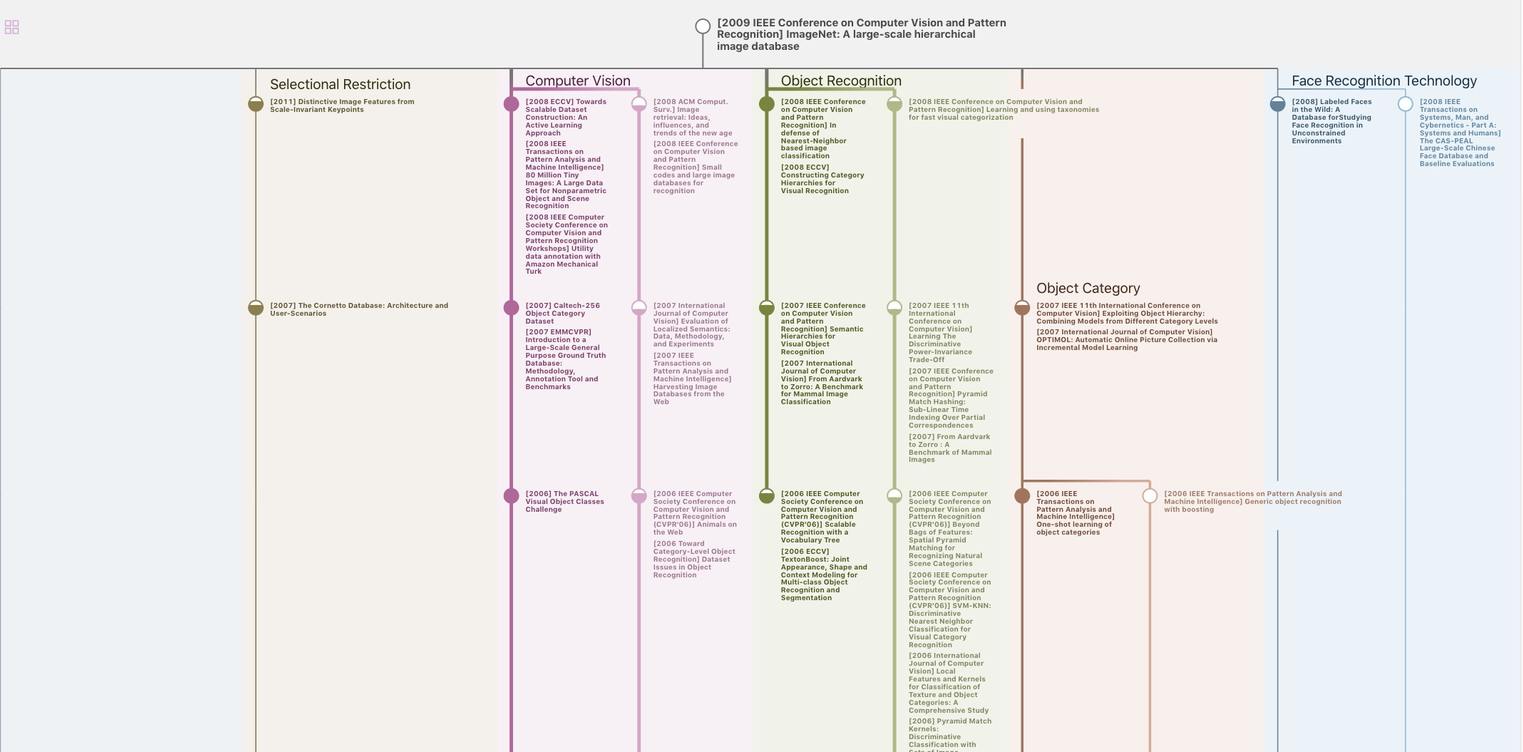
生成溯源树,研究论文发展脉络
Chat Paper
正在生成论文摘要