Wind speed forecasting using multi-site collaborative deep learning for complex terrain application in valleys
RENEWABLE ENERGY(2022)
摘要
Reliable ultra-short-term wind speed forecasts are essential for wind power consumption and scheduling and are an effective way to promote carbon neutrality. Wind farms are usually located in complex terrain with abundant wind resources, where traditional numerical weather forecasting and statistical methods are no longer sufficient to meet the demand. This study aims to address this challenge through a deep learning approach, and proposes a multisite collaborative deep learning (MS-CDL) based method. In the proposed wind speed forecasting model, state-of-the-art spatiotemporal mining algorithms and a framework of multi-task learning are used to mine deep spatiotemporal features in wind speed data using collaborative learning and knowledge sharing among multiple sites related by proximity. One-step-ahead and multi-step-ahead wind speed forecasting were conducted in realistic complex terrain scenarios, and the experimental results show that the proposed model requires only a small amount of computational resources and cost to achieve excellent forecasting results. For the T thorn 1 horizon using data for the four seasons of 2018, MAE for the MS-CDL model was less than it was for the single-site models CNN, LSTM and CNN-LSTM respectively by 16.5%, 11.0% and 7.5%; respective decreases in RMSE were 19.3%, 13.1% and 7.8%. (C)& nbsp;2022 Elsevier Ltd. All rights reserved.
更多查看译文
关键词
Complex terrain, Wind speed forecasting, Multisite collaborative deep learning, Multitask learning
AI 理解论文
溯源树
样例
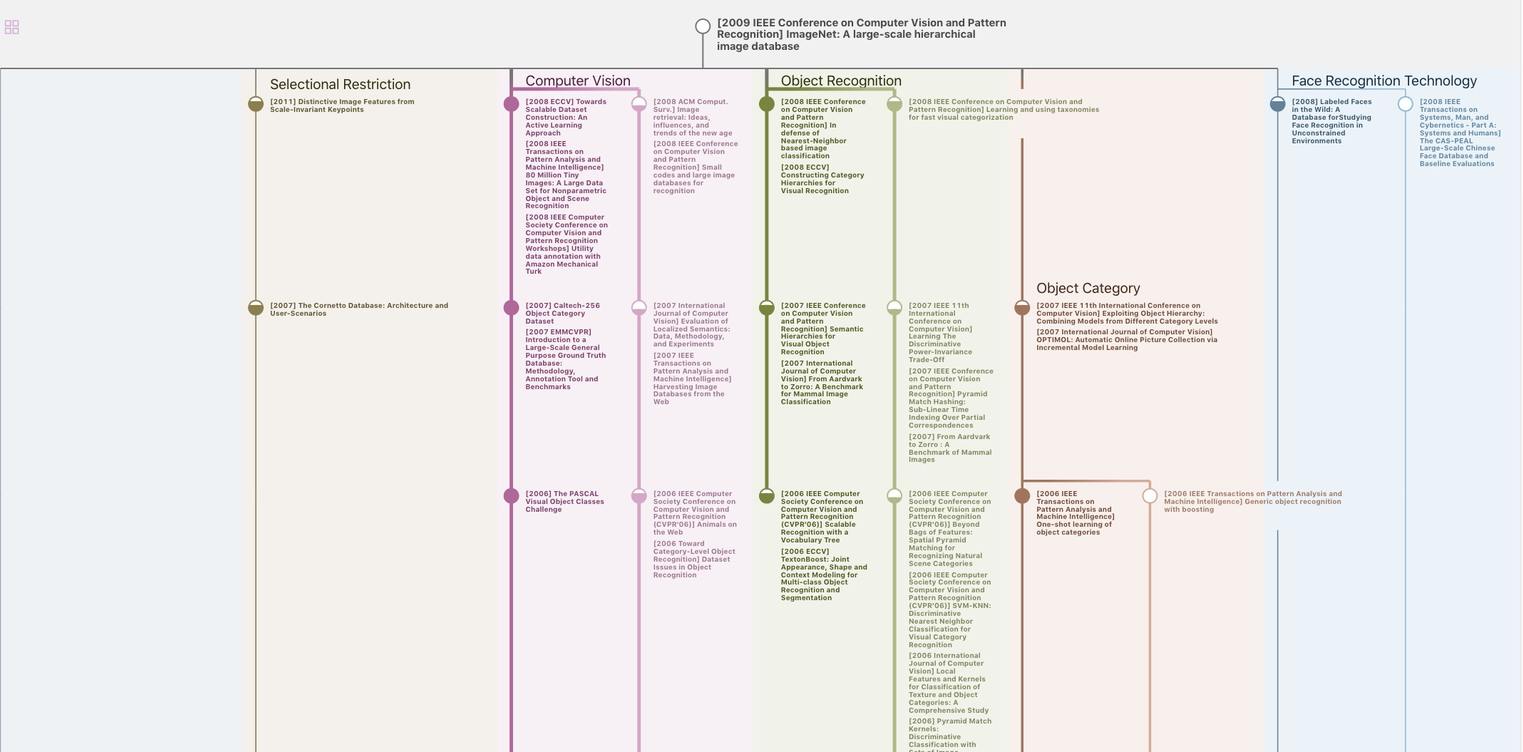
生成溯源树,研究论文发展脉络
Chat Paper
正在生成论文摘要