Classifying FRB Spectrograms Using Nonlinear Dimensionality Reduction Techniques
Monthly Notices of the Royal Astronomical Society(2023)
关键词
astronomical instrumentation,methods,and techniques,methods: data analysis,fast radio bursts
AI 理解论文
溯源树
样例
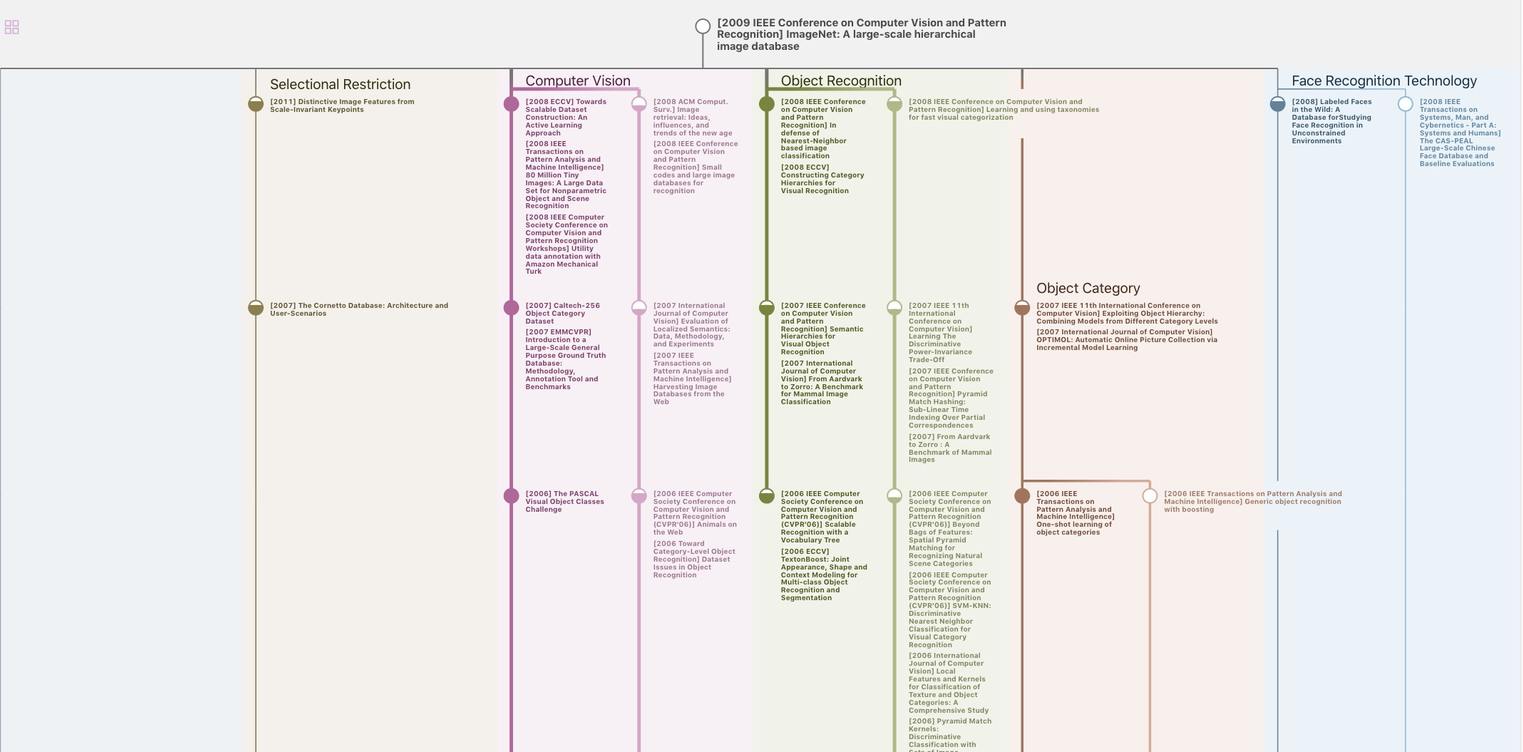
生成溯源树,研究论文发展脉络
Chat Paper
正在生成论文摘要