User Preference and Performance using Tagging and Browsing for Image Labeling
CHI 2023(2023)
摘要
Visual content must be labeled to facilitate navigation and retrieval, or provide ground truth data for supervised machine learning approaches. The efficiency of labeling techniques is crucial to produce numerous qualitative labels, but existing techniques remain sparsely evaluated. We systematically evaluate the efficiency of tagging and browsing tasks in relation to the number of images displayed, interaction modes, and the image visual complexity. Tagging consists in focusing on a single image to assign multiple labels (image-oriented strategy), and browsing in focusing on a single label to assign to multiple images (label-oriented strategy). In a first experiment, we focus on the nudges inducing participants to adopt one of the strategies (n=18). In a second experiment, we evaluate the efficiency of the strategies (n=24). Results suggest an image-oriented strategy (tagging task) leads to shorter annotation times, especially for complex images, and participants tend to adopt it regardless of the conditions they face.
更多查看译文
关键词
Human-Computer Interaction, empirical studies, user performance, image labeling, tagging, browsing, visual complexity, open science
AI 理解论文
溯源树
样例
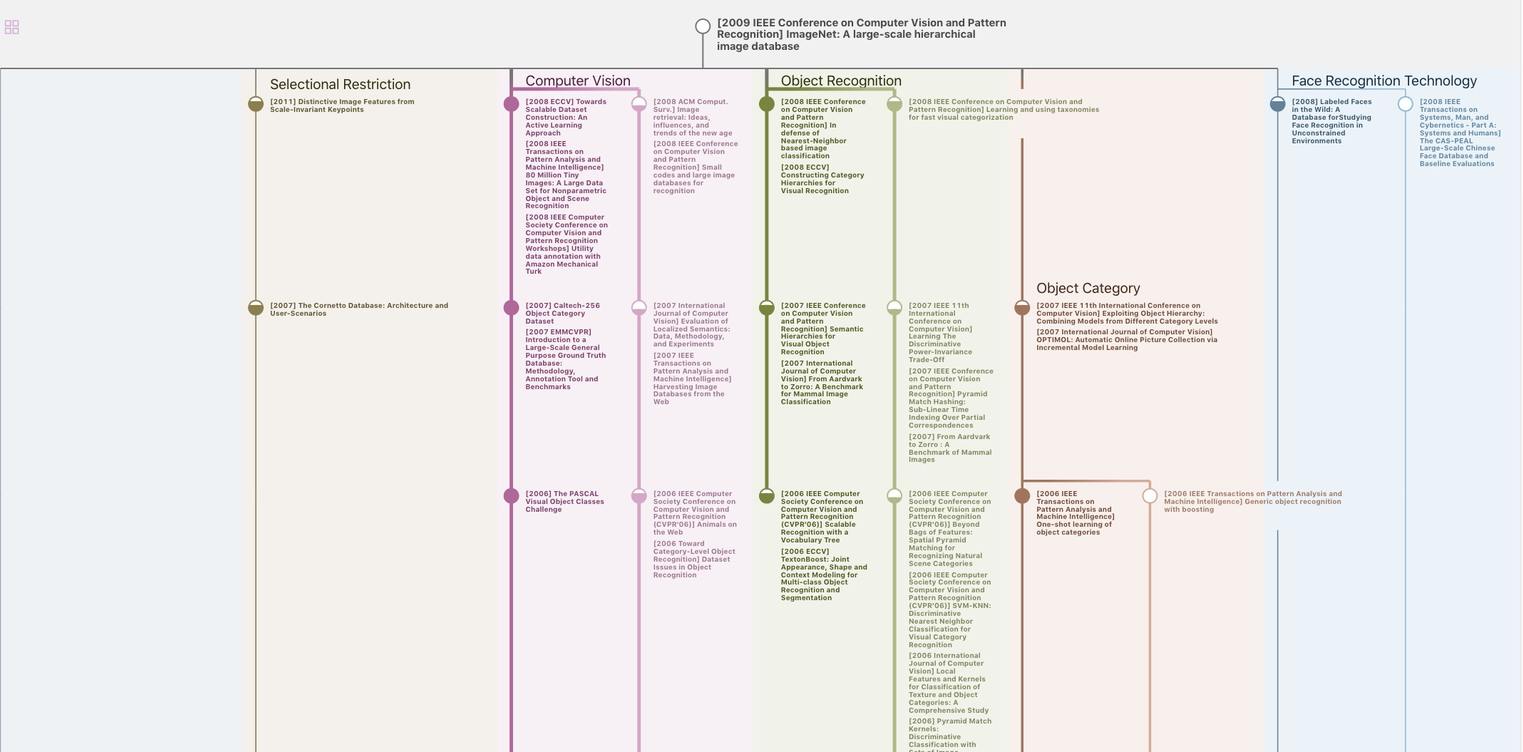
生成溯源树,研究论文发展脉络
Chat Paper
正在生成论文摘要