Effectiveness of three bioinformatics tools in the detection of ASD candidate variants from whole exome sequencing data
medrxiv(2023)
摘要
Background Autism spectrum disorder (ASD) is a heterogenous multifactorial neurodevelopmental condition with a significant genetic susceptibility component. Thus, identifying genetic variations associated with ASD is a complex task. Whole exome sequencing (WES) is an effective approach for detecting extremely rare protein-coding single nucleotide variants (SNVs). However, interpreting the functional and clinical consequences of these variants requires integrating multifaceted genomic information. Methods We compared the effectiveness of three bioinformatics tools in detecting ASD candidate SNVs from WES data of 250 ASD family trios registered in the National Autism Database of Israel. We studied only rare (less than 1% population frequency), proband specific SNVs. The pathogenicity of SNVs, according to the American College of Medical Genetics (ACMG) guidelines, was evaluated by the InterVar and TAPES tools. In addition, likely gene disrupting (LGD) SNVs were detected based on an inhouse bioinformatics tool, designated PsiVariant, that integrates results from seven insilico prediction tools. We compared the effectiveness of these three approaches and their combinations in detecting SNVs in high confidence ASD genes. Results Overall, 605 SNVs in 499 genes distributed in 193 probands were detected by these tools. The overlap between the tools was 64.1%, 17.0%, and 21.6% for InterVar and TAPES, InterVar and PsiVariant, and TAPES and PsiVariant, respectively. The intersection between InterVar and PsiVariant (I and P) was the most effective approach in detecting ASD genes (OR = 5.38, 95% C.I. = 3.25 to 8.53). This combination detected 102 SNVs in 99 genes among 80 probands (approximate 36% diagnostic yield). Conclusions Our results suggest that integration of different variant interpretation approaches in detecting ASD candidate SNVs from WES data is superior to each approach alone. Inclusion of additional criteria could further improve the detection of ASD candidate variants.
### Competing Interest Statement
The authors have declared no competing interest.
### Funding Statement
This study was supported by a grant from the Israel Science Foundation (1092/21).
### Author Declarations
I confirm all relevant ethical guidelines have been followed, and any necessary IRB and/or ethics committee approvals have been obtained.
Yes
The details of the IRB/oversight body that provided approval or exemption for the research described are given below:
The study was conducted according to the guidelines of the Declaration of Helsinki and approved by the Ethics Committee of Soroka University Medical Center (SOR-076-15; 17 April 2016).
I confirm that all necessary patient/participant consent has been obtained and the appropriate institutional forms have been archived, and that any patient/participant/sample identifiers included were not known to anyone (e.g., hospital staff, patients or participants themselves) outside the research group so cannot be used to identify individuals.
Yes
I understand that all clinical trials and any other prospective interventional studies must be registered with an ICMJE-approved registry, such as ClinicalTrials.gov. I confirm that any such study reported in the manuscript has been registered and the trial registration ID is provided (note: if posting a prospective study registered retrospectively, please provide a statement in the trial ID field explaining why the study was not registered in advance).
Yes
I have followed all appropriate research reporting guidelines, such as any relevant EQUATOR Network research reporting checklist(s) and other pertinent material, if applicable.
Yes
WES data were generated as part of the ASC and are available in dbGaP with study accession: phs000298.v4.p3. The generated results and codes are available in a GitHub public repository: https://github.com/AppWick-hub/Psi-Variant or available upon reasonable request to the corresponding author Prof. Idan Menashe (idanmen@bgu.ac.il).
更多查看译文
AI 理解论文
溯源树
样例
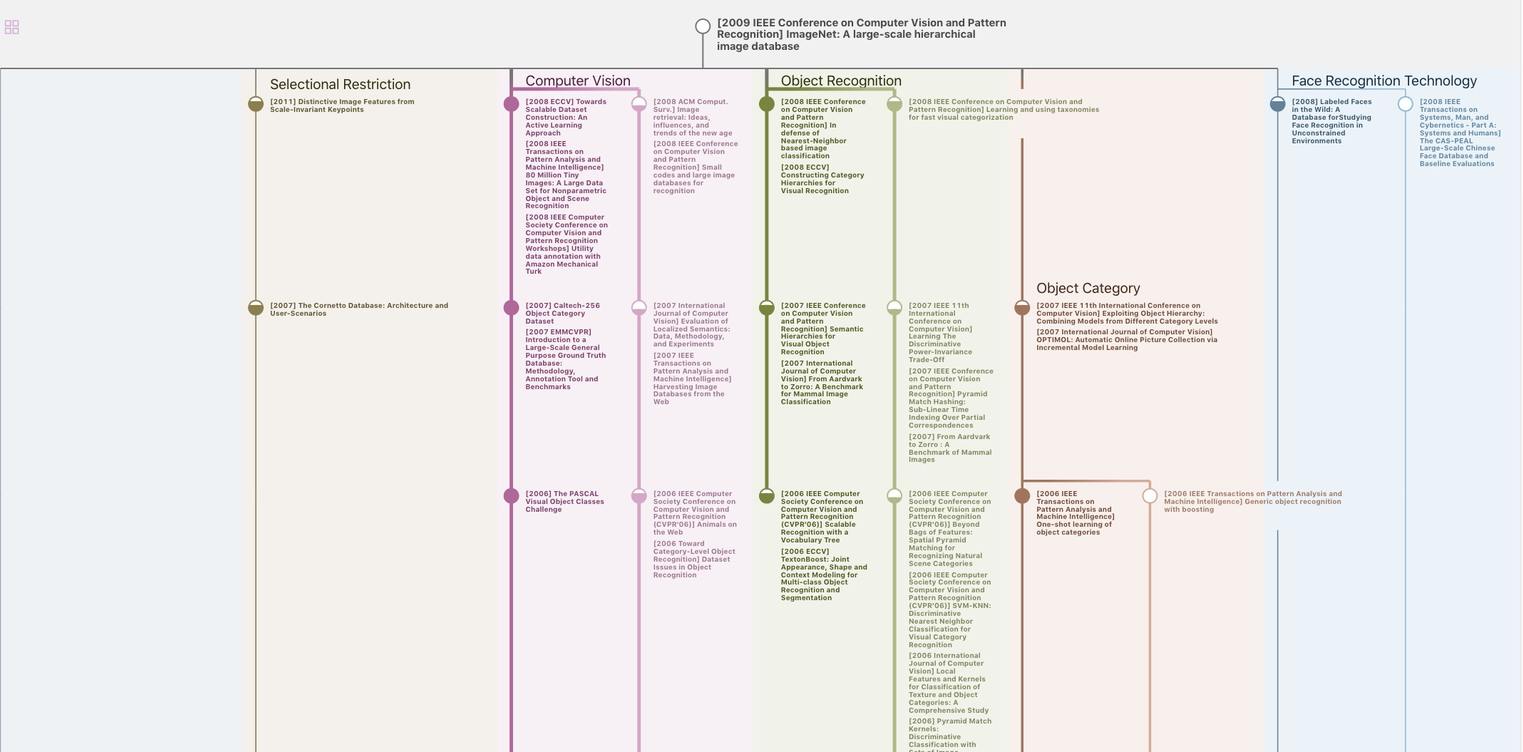
生成溯源树,研究论文发展脉络
Chat Paper
正在生成论文摘要