Attribute recognition for person re-identification using federated learning at all-in-edge
Internet of Things(2023)
摘要
The advancement in person re-identification using attribute recognition is constrained by the increasingly strict data privacy standards since it necessitates the centralization of vast amounts of data containing sensitive personal data in the cloud. Cloud-based person re-identification re-quires the transfer of original video information to the servers, causing increased communication costs because of the need for significant bandwidth, resulting in unpredictable timing. This work presents an all-in-edge architecture for attribute-based person re-identification, which deploys training data in edge nodes that support distributed inference. Edge nodes independently learn but collaborate with specific neighboring nodes by sharing information to minimize communication and computational costs through the utilization of federated learning and transfer learning methods. Furthermore, this paper proposes a federated aggregation strategy-FedTransferLoss to obtain optimal global accuracy by using transfer learning to re-train the low-quality local models. Extensive experiments on two prominent pedestrian datasets-PETA and RAP show that FedTransferLoss achieves higher accuracy, recall and precision values compared to the traditional FedAvg algorithm.
更多查看译文
关键词
Person re-identification, Edge computing, Federated learning, Transfer learning, Attribute recognition
AI 理解论文
溯源树
样例
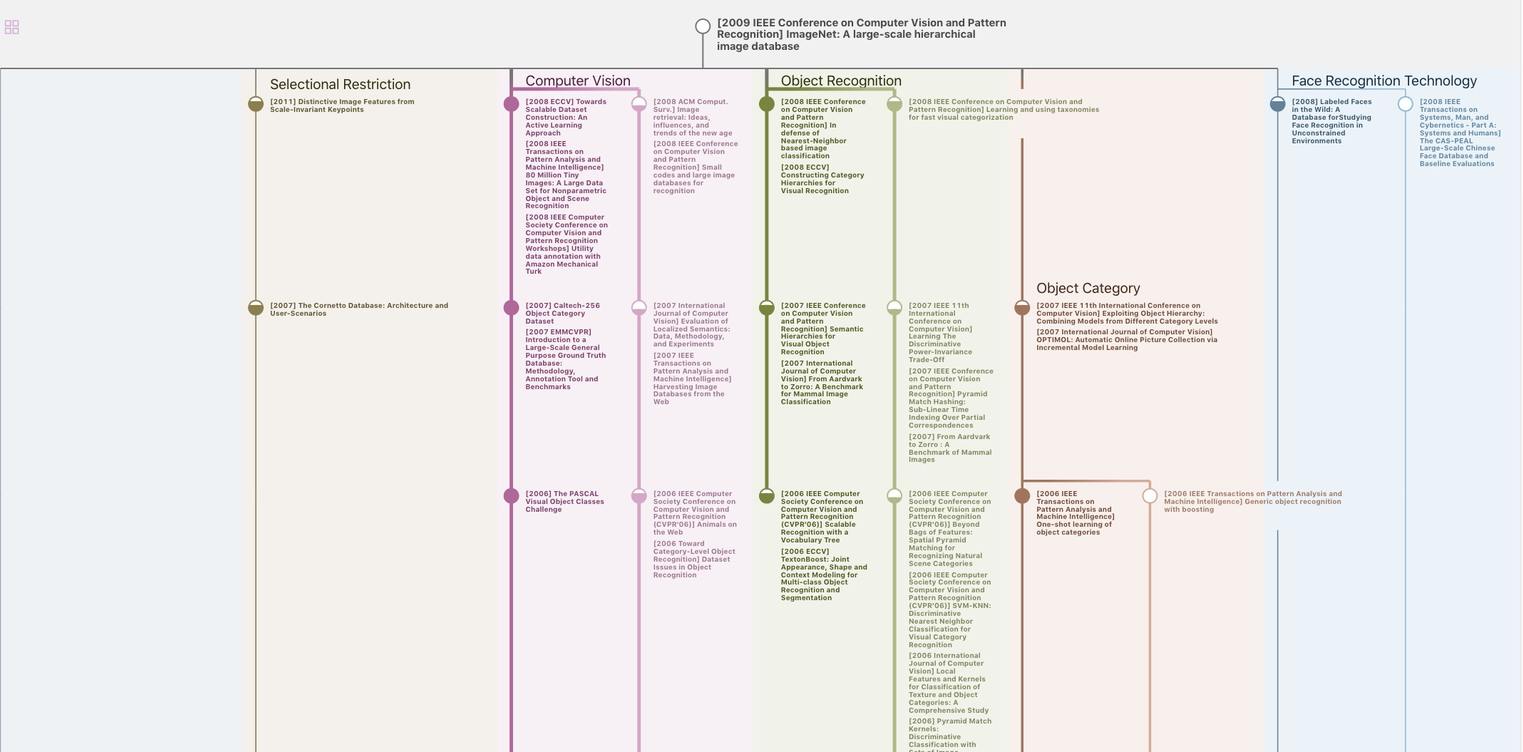
生成溯源树,研究论文发展脉络
Chat Paper
正在生成论文摘要