SACANet: scene-aware class attention network for semantic segmentation of remote sensing images
CoRR(2023)
摘要
Spatial attention mechanism has been widely used in semantic segmentation of remote sensing images given its capability to model long-range dependencies. Many methods adopting spatial attention mechanism aggregate contextual information using direct relationships between pixels within an image, while ignoring the scene awareness of pixels (i.e., being aware of the global context of the scene where the pixels are located and perceiving their relative positions). Given the observation that scene awareness benefits context modeling with spatial correlations of ground objects, we design a scene-aware attention module based on a refined spatial attention mechanism embedding scene awareness. Besides, we present a local-global class attention mechanism to address the problem that general attention mechanism introduces excessive background noises while hardly considering the large intra-class variance in remote sensing images. In this paper, we integrate both scene-aware and class attentions to propose a scene-aware class attention network (SACANet) for semantic segmentation of remote sensing images. Experimental results on three datasets show that SACANet outperforms other state-of-the-art methods and validate its effectiveness. Code is available at https://github.com/xwmaxwma/rssegmentation.
更多查看译文
关键词
Semantic Segmentation,Scene Awareness,Class Attention
AI 理解论文
溯源树
样例
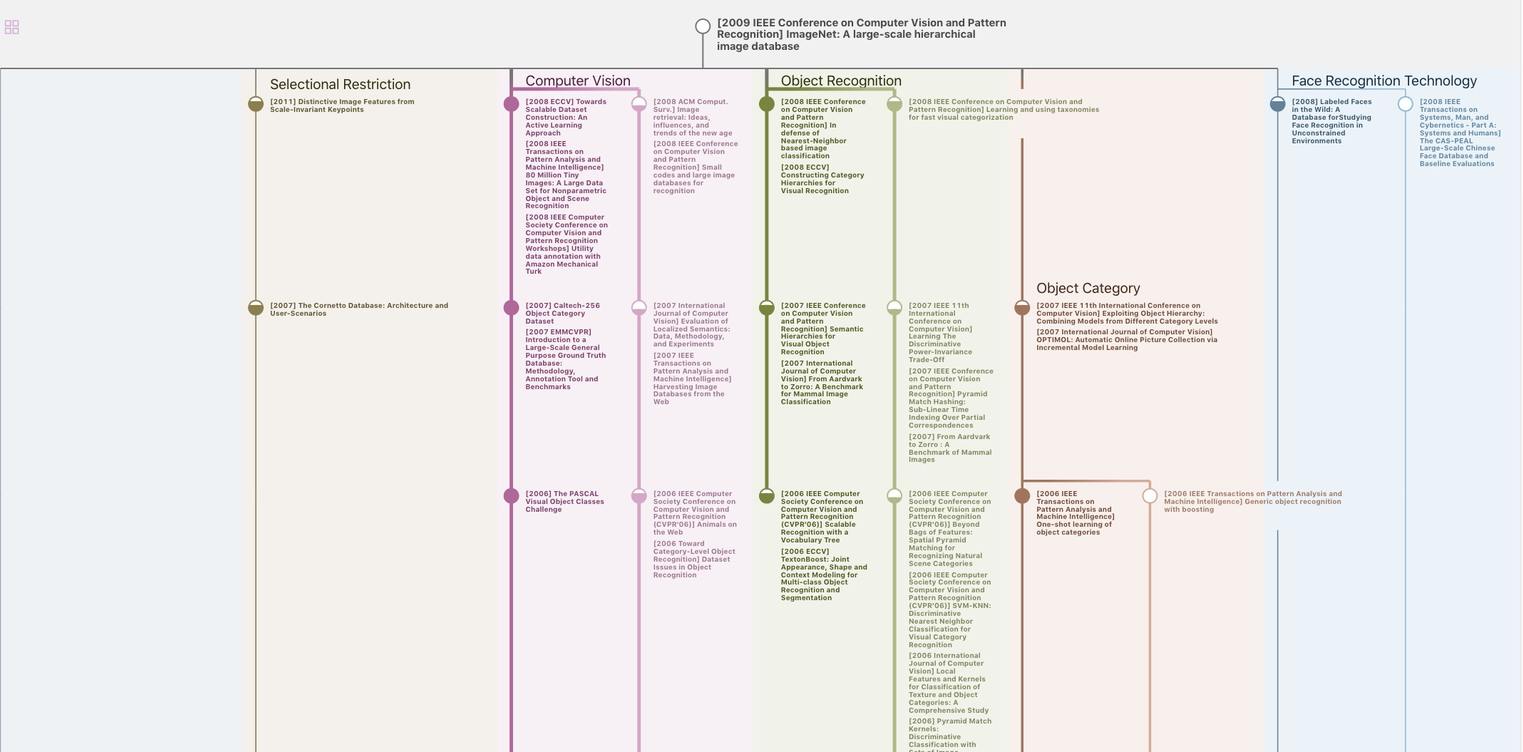
生成溯源树,研究论文发展脉络
Chat Paper
正在生成论文摘要