Individual Fairness in Bayesian Neural Networks
CoRR(2023)
摘要
We study Individual Fairness (IF) for Bayesian neural networks (BNNs). Specifically, we consider the $\epsilon$-$\delta$-individual fairness notion, which requires that, for any pair of input points that are $\epsilon$-similar according to a given similarity metrics, the output of the BNN is within a given tolerance $\delta>0.$ We leverage bounds on statistical sampling over the input space and the relationship between adversarial robustness and individual fairness to derive a framework for the systematic estimation of $\epsilon$-$\delta$-IF, designing Fair-FGSM and Fair-PGD as global,fairness-aware extensions to gradient-based attacks for BNNs. We empirically study IF of a variety of approximately inferred BNNs with different architectures on fairness benchmarks, and compare against deterministic models learnt using frequentist techniques. Interestingly, we find that BNNs trained by means of approximate Bayesian inference consistently tend to be markedly more individually fair than their deterministic counterparts.
更多查看译文
关键词
bayesian neural networks,fairness,neural networks
AI 理解论文
溯源树
样例
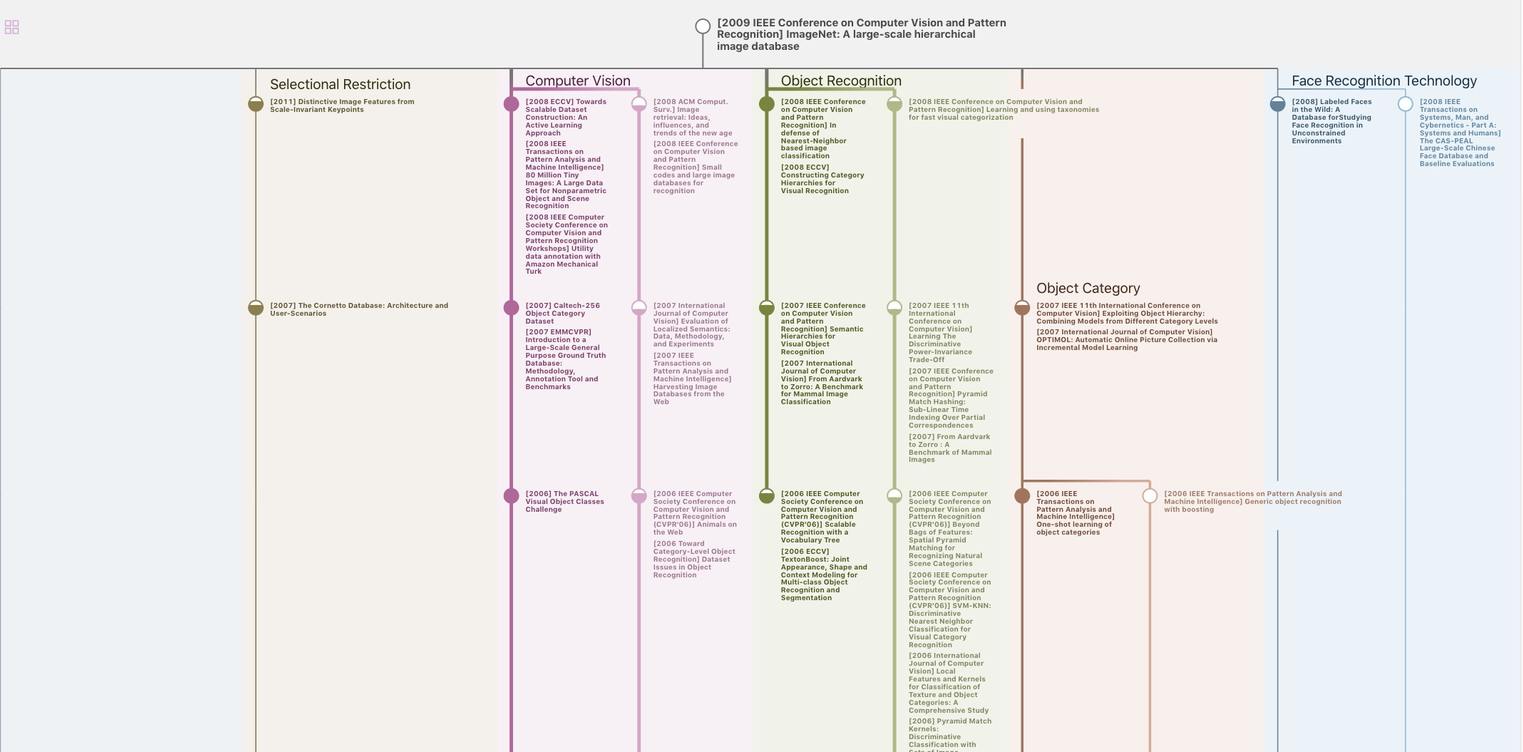
生成溯源树,研究论文发展脉络
Chat Paper
正在生成论文摘要