Embedding expert opinion in a Bayesian network model to predict wheat yield from spring-summer weather
SMART AGRICULTURAL TECHNOLOGY(2023)
摘要
Wheat yield is highly dependent on weather, Therefore, predicting its effect can improve crop management decisions. Various modelling approaches have been used to predict wheat yield including process-based modelling, statistical models, and machine learning. However, these models typically require a large data set for training or fitting. They often also have a limited ability in capturing the effects of small-scale variability, time, and duration of extreme weather events. Here, we develop a Bayesian Network (BN) model by interviewing experts including farmers, embedding their knowledge from years of experience within a quantitative model. These experts identified the period from the beginning of anthesis to the end of grain filling stage as a critical period and maximum temperature, mean temperature and precipitation as key weather variables for inclusion in the BN. To keep the time input from experts manageable, the conditional probability table for the BN was constructed based on their anticipated impact on the mean yield of different weather conditions. The model predicted the yield in the same or neighbouring class (very low, low, medium, high and very high) as the reported yield with low error rate ranging from 9.1 to 15.2% and, when used to estimate the median predicted yield, R2 ranging from 41 to 52%. Interestingly, model successfully predicted the yield in years 1998, 2007, 2012 and 2020 which had the most extreme weather events. Additionally, the more recent data, from 2012 to 2022 was predicted more accurately, especially 2022 season which was not sown yet when eliciting information and recently added to the testing data. Little difference was observed between the predictions made using model parameters based only the opinion of the farm manager from which the test data originated, and the predictions made using the average opinion of a group of 9 experts. The inclusion of causal variables in the model also provided insight into the experts' rationale, allowing unexpected results to be explored. This methodology provides a means to rapidly develop a successful predictive model of wheat yield with limited (or no) data using expert understanding. This model could be tuned and updated with data as it becomes available.
更多查看译文
关键词
Winer wheat,Weather condition,Expert opinion,Bayesian network
AI 理解论文
溯源树
样例
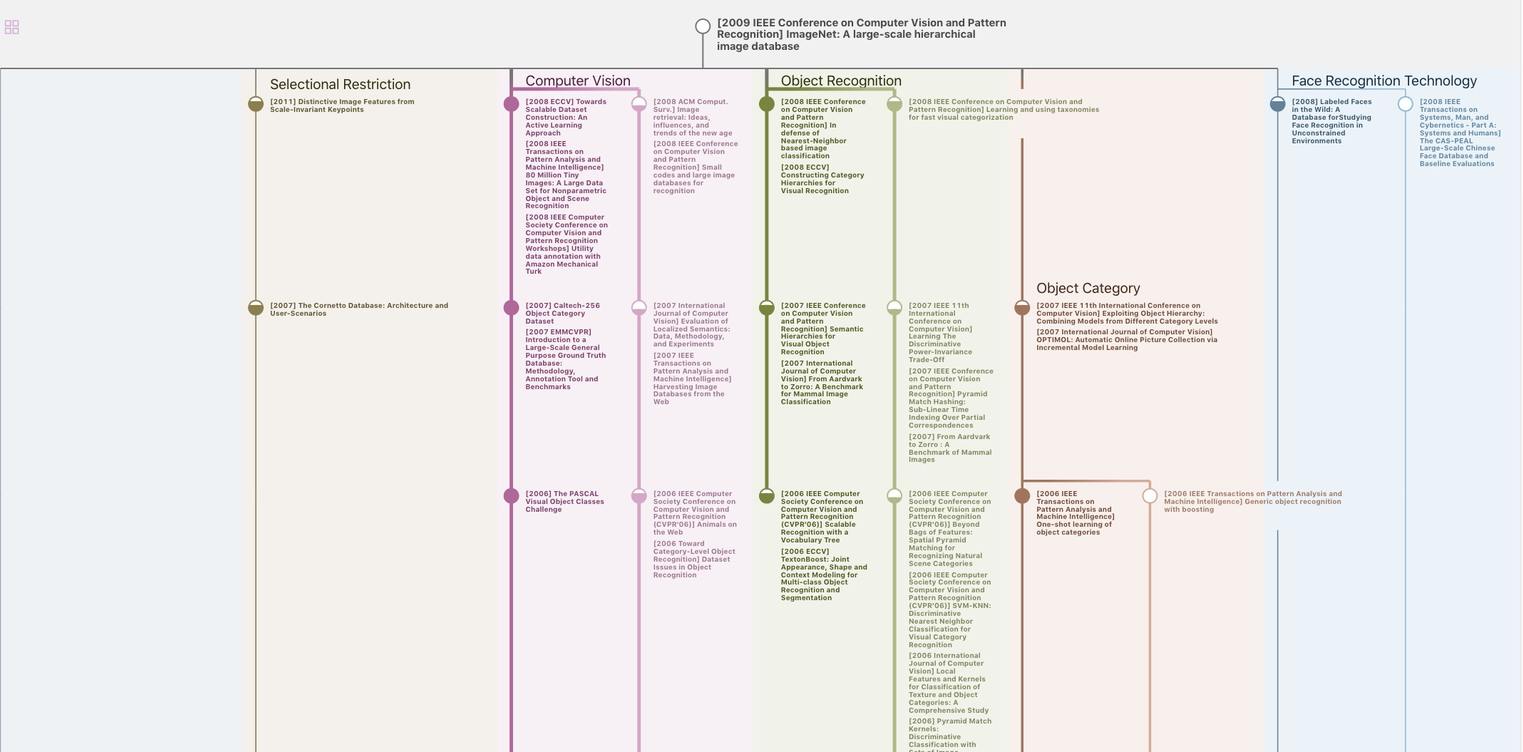
生成溯源树,研究论文发展脉络
Chat Paper
正在生成论文摘要