Causal deep learning models for studying the Earth system
Geoscientific Model Development(2023)
摘要
Earth is a complex non-linear dynamical system. Despite decades of research and considerable scientific and methodological progress, many processes and relations between Earth system variables remain poorly understood. Current approaches for studying relations in the Earth system rely either on numerical simulations or statistical approaches. However, there are several inherent limitations to existing approaches, including high computational costs, uncertainties in numerical models, strong assumptions about linearity or locality, and the fallacy of correlation and causality. Here, we propose a novel methodology combining deep learning (DL) and principles of causality research in an attempt to overcome these limitations. On the one hand, we employ the recent idea of training and analyzing DL models to gain new scientific insights into relations between input and target variables. On the other hand, we use the fact that a statistical model learns the causal effect of an input variable on a target variable if suitable additional input variables are included. As an illustrative example, we apply the methodology to study soil-moisture–precipitation coupling in ERA5 climate reanalysis data across Europe. We demonstrate that, harnessing the great power and flexibility of DL models, the proposed methodology may yield new scientific insights into complex non-linear and non-local coupling mechanisms in the Earth system.
更多查看译文
关键词
causal deep learning models,deep learning,earth
AI 理解论文
溯源树
样例
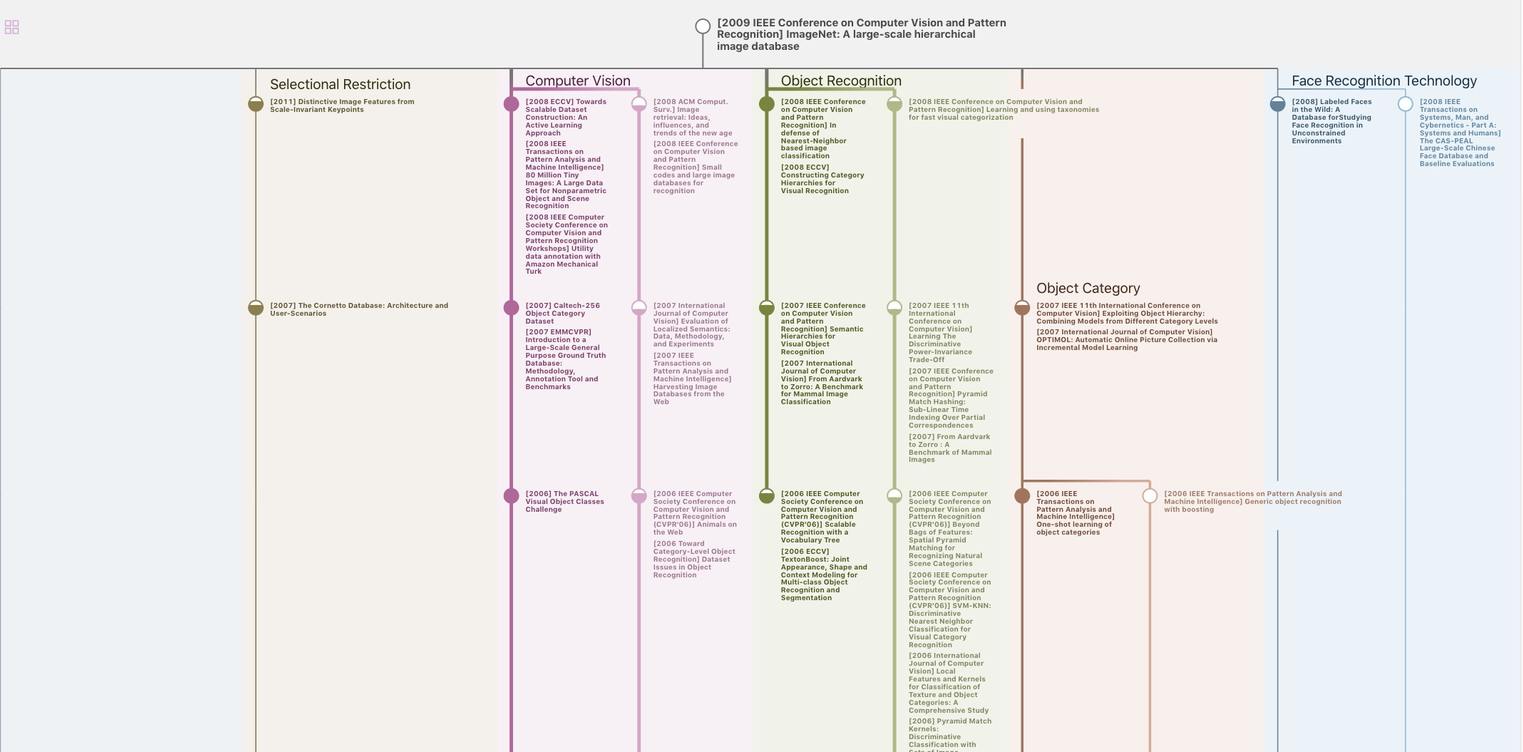
生成溯源树,研究论文发展脉络
Chat Paper
正在生成论文摘要