MFSE: A Meta-Fusion Model for Polypharmacy Side-Effect Prediction with Graph Neural Networks
2022 IEEE 34th International Conference on Tools with Artificial Intelligence (ICTAI)(2022)
摘要
Despite being a very popular approach for treating complex diseases, polypharmacy can lead to increased risk of adverse side effects, many of which are observed after the drugs have been released in the market. Luckily, the significant increase in data availability of observed adverse side-effects has paved the way for machine learning approaches to assist in their prediction. In this work, we first present a novel framework for multi-relational link prediction with graph neural networks. Given a multi-relational graph, we create relation-specific vector representations for each node of the graph. With this approach, we create drug vector representations that are side-effect specific, by integrating external molecular and protein-target information with the drug information that is generated directly from the drug-drug interaction prediction graph. With our new meta-fusion approach, each information type is produced from a distinct G NN - based encoder architecture and then the integration is performed according to the side-effect type being predicted. While state-of-the-art models report maximum AUROC scores of 0.91, our technique reaches a score of 0.95. Also, we show that our fusion approach provides valuable external knowledge particularly to drug nodes in the prediction graph that have a smaller node degree.
更多查看译文
关键词
graph neural networks,polypharmacy side-effect prediction,multi-relational link prediction,data fusion
AI 理解论文
溯源树
样例
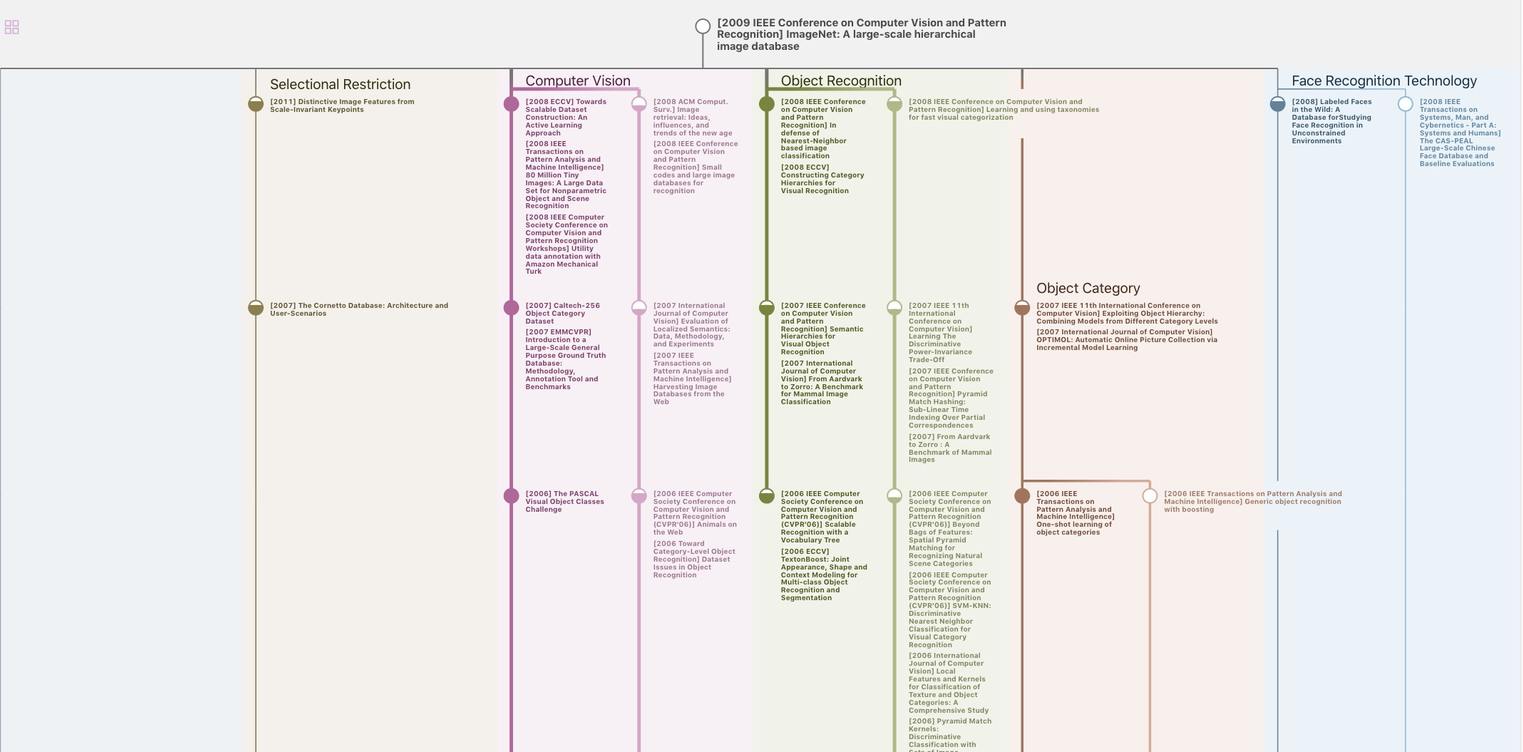
生成溯源树,研究论文发展脉络
Chat Paper
正在生成论文摘要