Learning from Suboptimal Demonstration via Trajectory-Ranked Adversarial Imitation
2022 IEEE 34th International Conference on Tools with Artificial Intelligence (ICTAI)(2022)
摘要
Robots trained by Imitation Learning(IL) are used in many tasks(e.g., autonomous vehicle manipulation). Generative Adversarial Imitation Learning (GAIL) assumes that the demonstration set used for training is of high quality. However, such demonstrations are difficult and expensive to obtain. GAIL-related methods fail to learn effective strategies if non-high quality demonstrations are used because the performance of agents trained by this method is limited by the demonstrator's operations. Our idea is to enable the agent to learn strategy with better performance than the demonstrator from a suboptimal demonstration set, which contains non-high quality demonstrations that are easier to obtain. Inspired by this, we propose the Trajectory-Ranked Adversarial Imitation Learning (TRAIL) method. First, for demonstration set processing, we introduce a ranking process and define the concept of Performance Relative Advantage of suboptimal demonstrations to specify the ranking order. Second, for model training, we reconstruct the objective function of GAIL and use an experience replay buffer, enabling the agent to learn implicit features and ranking information from the ranked suboptimal demonstration set and possess the ability to outperform the demonstrator. Experiments show that in Mujoco's tasks, our method can learn from a suboptimal demonstration set and can achieve better performance than baseline methods.
更多查看译文
关键词
Reinforcement learning,Imitation learning,Suboptimal demonstration,Trajectory-Ranked
AI 理解论文
溯源树
样例
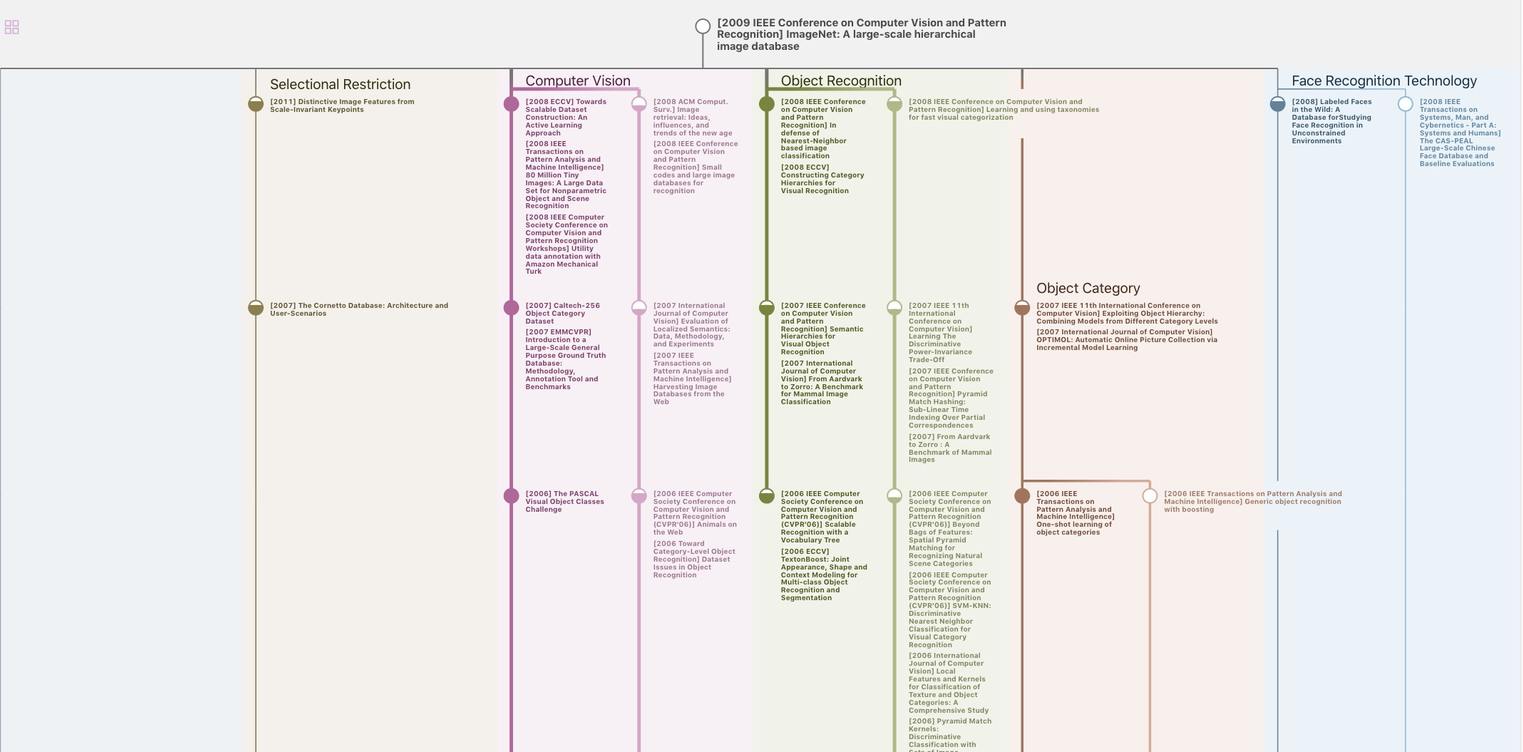
生成溯源树,研究论文发展脉络
Chat Paper
正在生成论文摘要