CSGP: Closed-Loop Safe Grasp Planning via Attention-Based Deep Reinforcement Learning From Demonstrations
IEEE Robotics and Automation Letters(2023)
摘要
Grasping is at the core of many robotic manipulation tasks. Despite the recent progress, closed-loop grasp planning in stacked scenes is still unsatisfactory, in terms of efficiency, stability, and most importantly, safety. In this letter, we present CSGP, a closed-loop safe grasp planning approach via attention-based deep reinforcement learning (DRL) from demonstrations, which is capable of learning safe grasping policies that make surrounding objects less disturbed or damaged during manipulation. In CSGP, a 6-DoF safe grasping policy network with a Next-Best-Region attention module is proposed to intrinsically identify the safe regions in the view, facilitating the learning of safe grasping actions. Moreover, we design a fully automatic pipeline in the simulator to collect safe grasping demonstrations, which are utilized to pre-train the policy with behavior cloning and fine-tune it with DRL. To effectively and stably improve the policy during fine-tuning, a DRL from demonstrations algorithm named Safe-DDPGfD is presented in CSGP with a truncated height-anneal exploration mechanism for safe exploration. Moreover, we provide a benchmark that contains scenes with multiple levels of stack layers for method evaluation. Simulation results demonstrate the state-of-the-art performance of our method, achieving the Overall score of 88% in our benchmark. Also, real-world robot grasping experiments also show the effectiveness of our method.
更多查看译文
关键词
Deep learning in grasping and manipulation,deep learning for visual perception,learning from demonstration
AI 理解论文
溯源树
样例
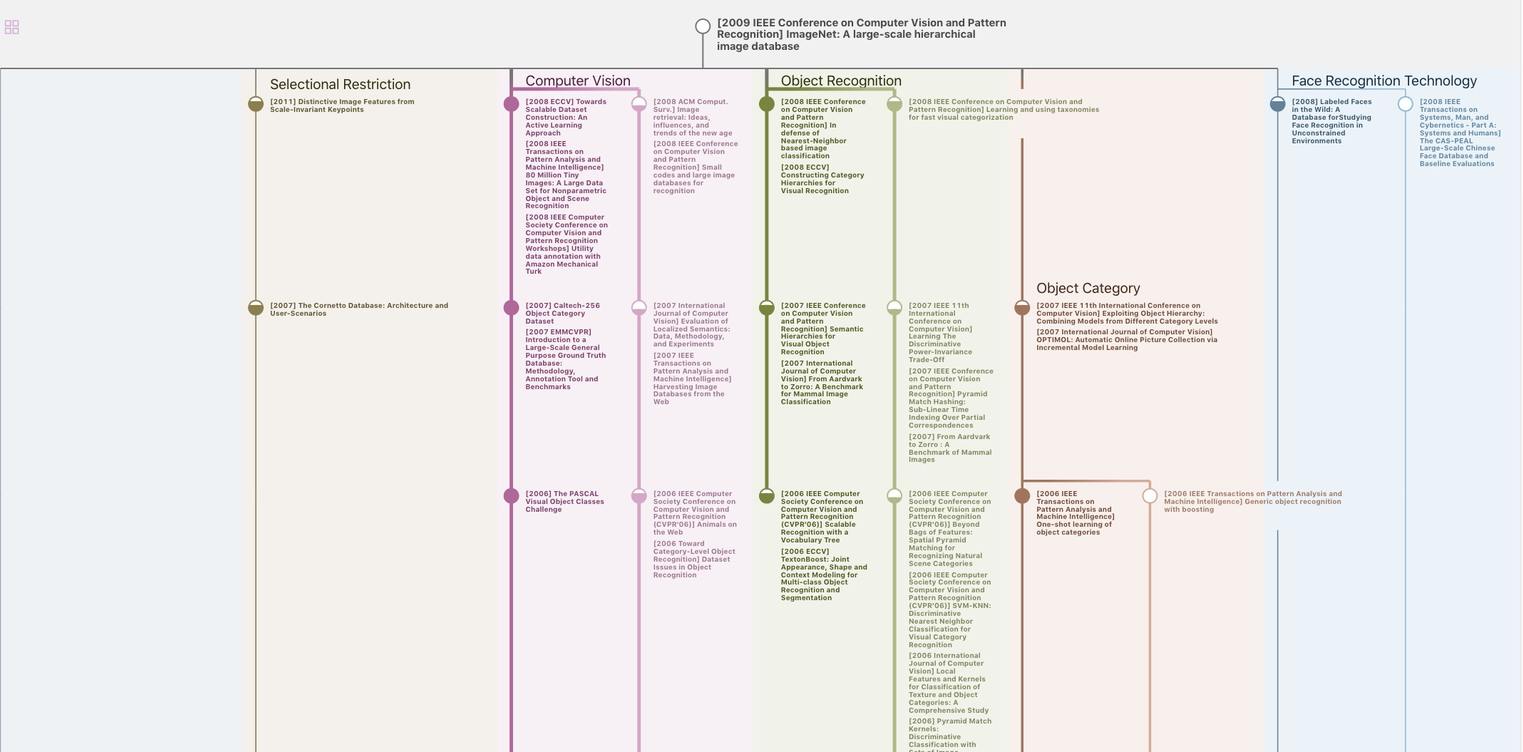
生成溯源树,研究论文发展脉络
Chat Paper
正在生成论文摘要