How to make embeddings suitable for PLDA
Computer Speech & Language(2023)
摘要
Probabilistic linear discriminant analysis (PLDA) is widely implemented in speaker verification tasks. However, PLDA has limitations owing to its assumptions. In this study, we explore how to make deep speaker embeddings suitable for PLDA in complex situations. We analyze PLDA in detail and summarize its three important properties, Gaussianity, simplicity, and domain sensitivity. For the Gaussianity, by comparing the discrimination and Gaussianity of embeddings extracted from different layers of speaker extractors with different numbers of segment-level fully connected (Fc) layers, we demonstrate that embeddings extracted from the first Fc layer of models with two segment-level Fc layers are more suitable for PLDA. Secondly, several common speaker datasets comprise multiple short-duration speech segments extracted from long speech. We find that embeddings of short speech segments extracted from the long speech are less reliable and have complex within-class distributions. By determining the weighted average of embeddings extracted from short-duration speech segments, we simplify the embeddings distribution and make the embeddings suitable for PLDA. Thirdly, PLDA is sensitive to domain mismatches. We propose data adaptation methods that work directly on raw speech to eliminate explicit mismatches, such as the codecs and the environment noise mismatches. We prove that the data adaptation methods achieve performance improvements of PLDA and show strong complementarity with backend adaptation methods. We conduct extensive experiments, using the NIST SRE CTS superset, VoxCeleb, and SRE16 as the training set, and the SRE21 set as the evaluation set mainly. The experimental results show that our methods effectively improve the overall performance of PLDA.
更多查看译文
关键词
Speaker verification, Probabilistic linear discriminant analysis, Deep speaker embedding, Gaussian, Simplicity, Domain sensitivity, Data adaptation
AI 理解论文
溯源树
样例
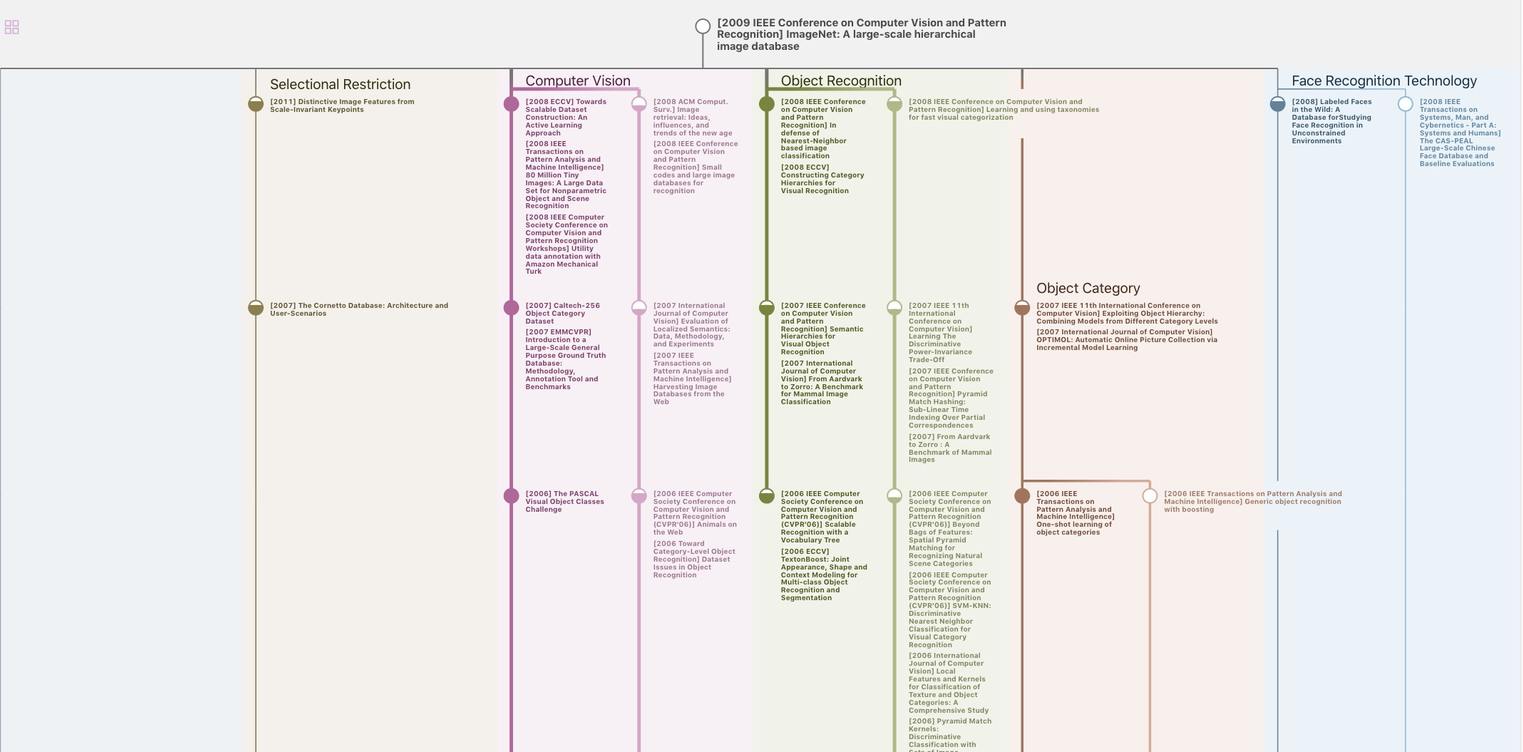
生成溯源树,研究论文发展脉络
Chat Paper
正在生成论文摘要