Learning task-agnostic and interpretable subsequence-based representation of time series and its applications in fMRI analysis
Neural Networks(2023)
摘要
The recent success of sequential learning models, such as deep recurrent neural networks, is largely due to their superior representation-learning capability for learning the informative representation of a targeted time series. The learning of these representations is generally goal-directed, resulting in their task-specific nature, giving rise to excellent performance in completing a single downstream task but hindering between-task generalisation. Meanwhile, with increasingly intricate sequential learning models, learned representation becomes abstract to human knowledge and comprehension. Hence, we propose a unified local predictive model based on the multi-task learning paradigm to learn the task-agnostic and interpretable subsequence-based time series representation, allowing versatile use of learned representations in temporal prediction, smoothing, and classification tasks. The targeted interpretable representation could convey the spectral information of the modelled time series to the level of human comprehension. Through a proof-of-concept evaluation study, we demonstrate the empirical superiority of learned task-agnostic and interpretable representation over task-specific and conventional subsequence-based representation, such as symbolic and recurrent learning-based representation, in solving temporal prediction, smoothing, and classification tasks. These learned task-agnostic representations can also reveal the ground-truth periodicity of the modelled time series. We further propose two applications of our unified local predictive model in functional magnetic resonance imaging (fMRI) analysis to reveal the spectral characterisation of cortical areas at rest and reconstruct more smoothed temporal dynamics of cortical activations in both resting-state and task-evoked fMRI data, giving rise to robust decoding.
更多查看译文
关键词
Multi-task learning paradigm,Subsequence-based time-series analysis,fMRI analysis
AI 理解论文
溯源树
样例
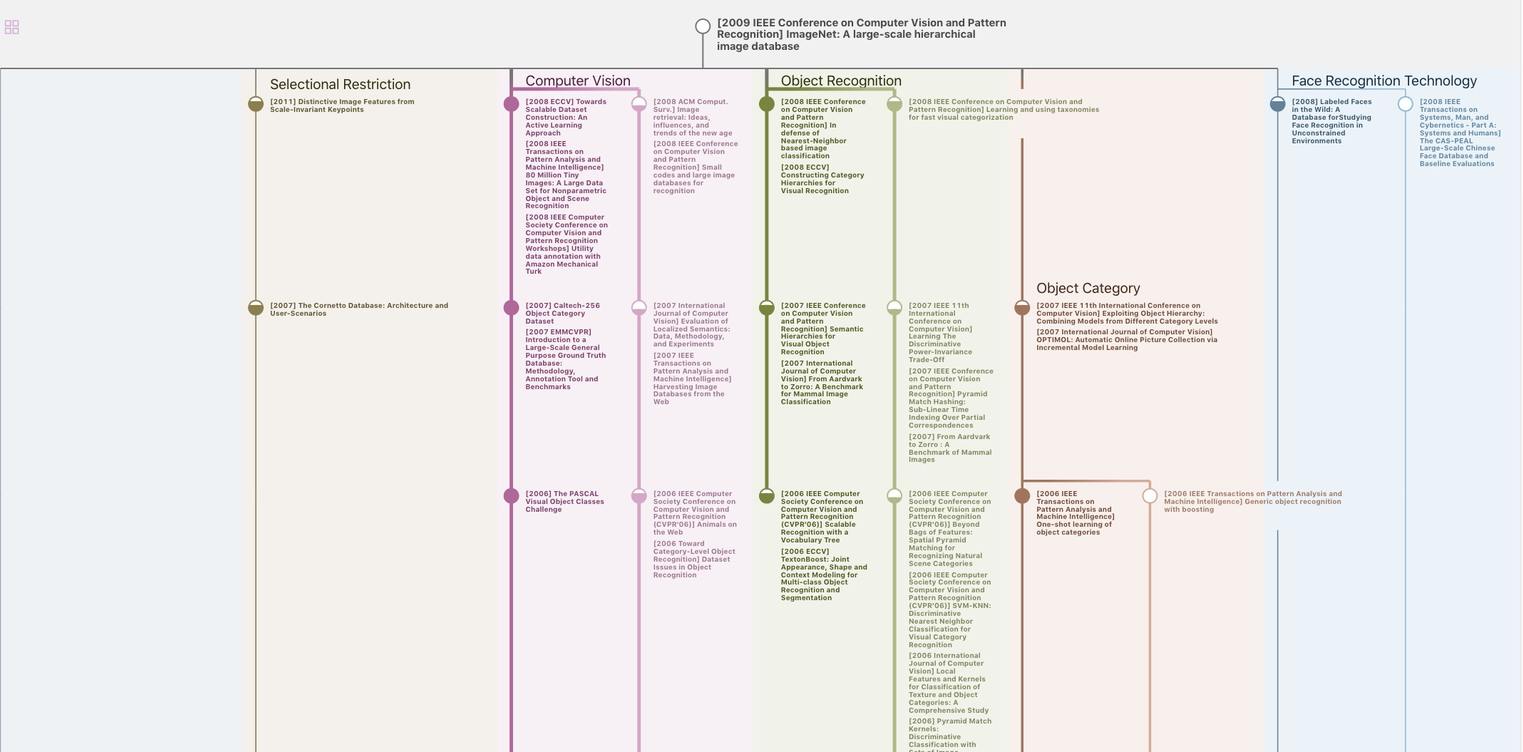
生成溯源树,研究论文发展脉络
Chat Paper
正在生成论文摘要