Tractable Control for Autoregressive Language Generation
CoRR(2023)
Abstract
Despite the success of autoregressive large language models in text generation, it remains a major challenge to generate text that satisfies complex constraints: sampling from the conditional distribution $\Pr(\text{text} | \alpha)$ is intractable for even the simplest lexical constraints $\alpha$. To overcome this challenge, we propose to use tractable probabilistic models to impose lexical constraints in autoregressive text generation, which we refer to as GeLaTo. To demonstrate the effectiveness of this framework, we use distilled hidden Markov models to control autoregressive generation from GPT2. GeLaTo achieves state-of-the-art performance on CommonGen, a challenging benchmark for constrained text generation, beating a wide range of strong baselines by a large margin. Our work not only opens up new avenues for controlling large language models but also motivates the development of more expressive tractable probabilistic models.
MoreTranslated text
Key words
tractable control,language,generation
AI Read Science
Must-Reading Tree
Example
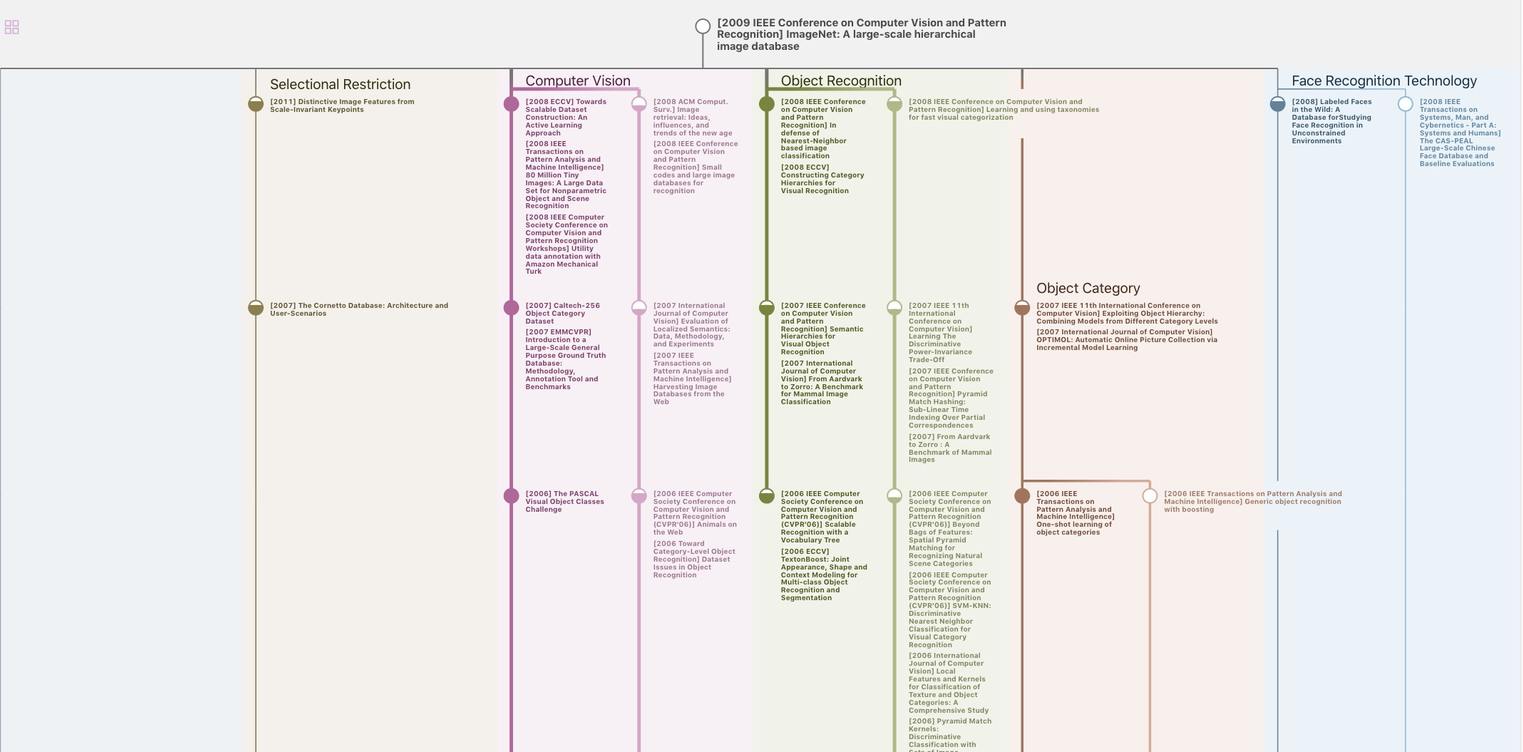
Generate MRT to find the research sequence of this paper
Chat Paper
Summary is being generated by the instructions you defined