Lifelong Learning Architecture of Video Surveillance System
Journal of Multimedia Information System(2023)
摘要
The learning capacity of general deep learning models for object detection would not be large enough to represent real-world scene dynamics, and thus such models would be weak to ‘unseen’ data due to environmental changes. To address this issue, online or active learning methods use data samples obtained in new environments, where the new samples collected from false and/or miss detection cases are used to re-train the original model to enhance detection precision. However, it is inevitably degraded over time due to the catastrophic forgetting problem, that is a well-known intrinsic problem of current deep learning technologies. In this study, we propose a cutting-edge end-to-end system architecture to continuously improve the accuracy of the video analytic algorithms such as object detection with less accuracy degradation, by utilizing a hybrid combination of intelligence both the front-end and back-end systems. We use an iterative process where the current model is self-evolving using new incoming data as part of an ongoing adaptation process. We carried out several experiments of person detection in surveillance videos with various challenging environmental changes and showed the high precision and adaptability of our new architecture while it can be practically implemented at a low cost.
更多查看译文
关键词
video surveillance system,surveillance system,learning
AI 理解论文
溯源树
样例
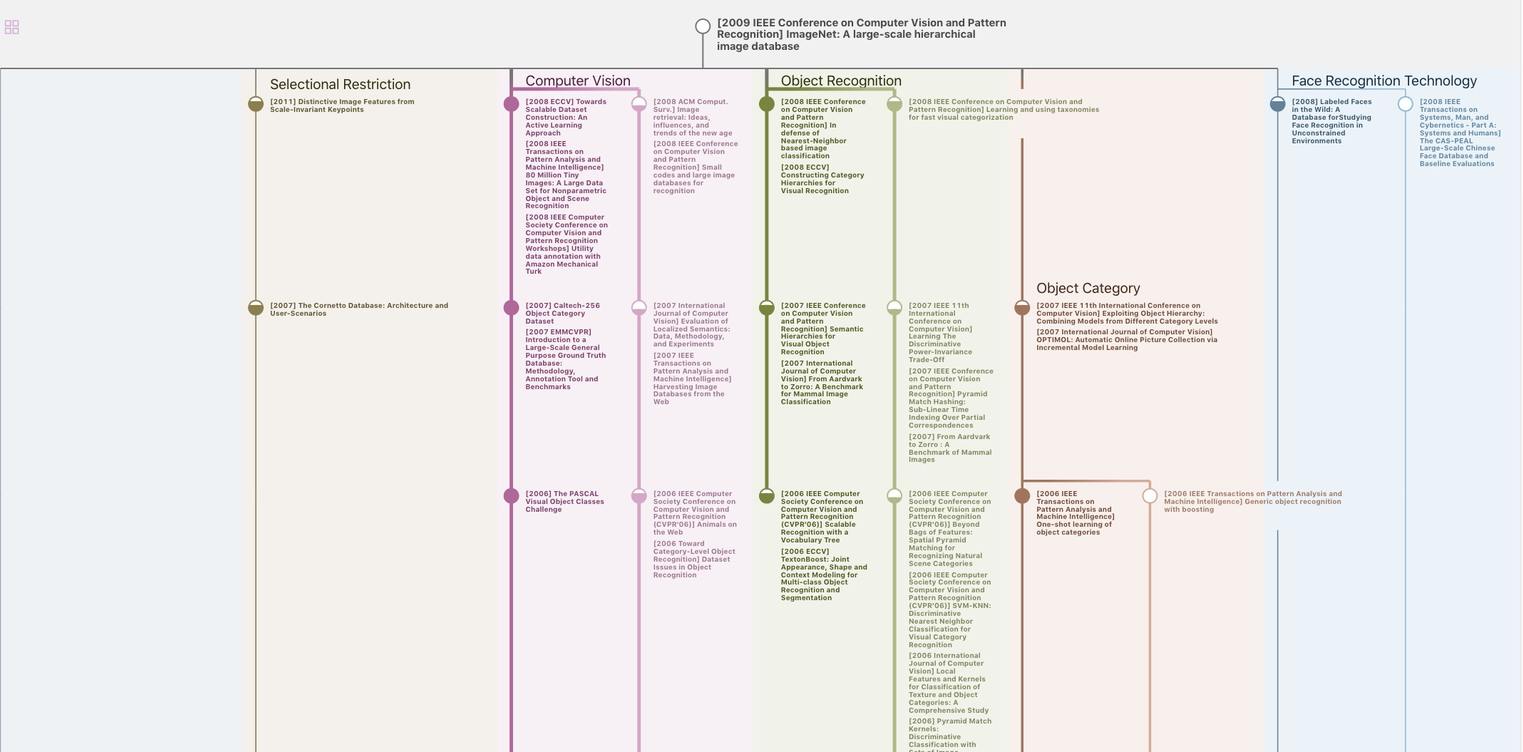
生成溯源树,研究论文发展脉络
Chat Paper
正在生成论文摘要