High-resolution probabilistic load forecasting: A learning ensemble approach
Journal of the Franklin Institute(2023)
摘要
High-resolution probabilistic load forecasting can comprehensively characterize both the uncertainties and the dynamic trends of the future load. Such information is key to the reliable operation of the future power grid with a high penetration of renewables. To this end, various high-resolution probabilistic load forecasting models have been proposed in recent decades. Compared with a single model, it is widely acknowledged that combining different models can further enhance the prediction performance, which is called the model ensemble. However, existing model ensemble approaches for load forecasting are linear combination-based, like mean value ensemble, weighted average ensemble, and quantile regression, and linear combinations may not fully utilize the advantages of different models, seriously limiting the performance of the model ensemble. We propose a learning ensemble approach that adopts the machine learning model to directly learn the optimal nonlinear combination from data. We theoretically demonstrate that the proposed learning ensemble approach can outperform conventional ensemble approaches. Based on the proposed learning ensemble model, we also introduce a Shapley value-based method to evaluate the contributions of each model to the model ensemble. The numerical studies on field load data verify the remarkable performance of our proposed approach.
更多查看译文
关键词
probabilistic load forecasting,ensemble,learning,high-resolution high-resolution
AI 理解论文
溯源树
样例
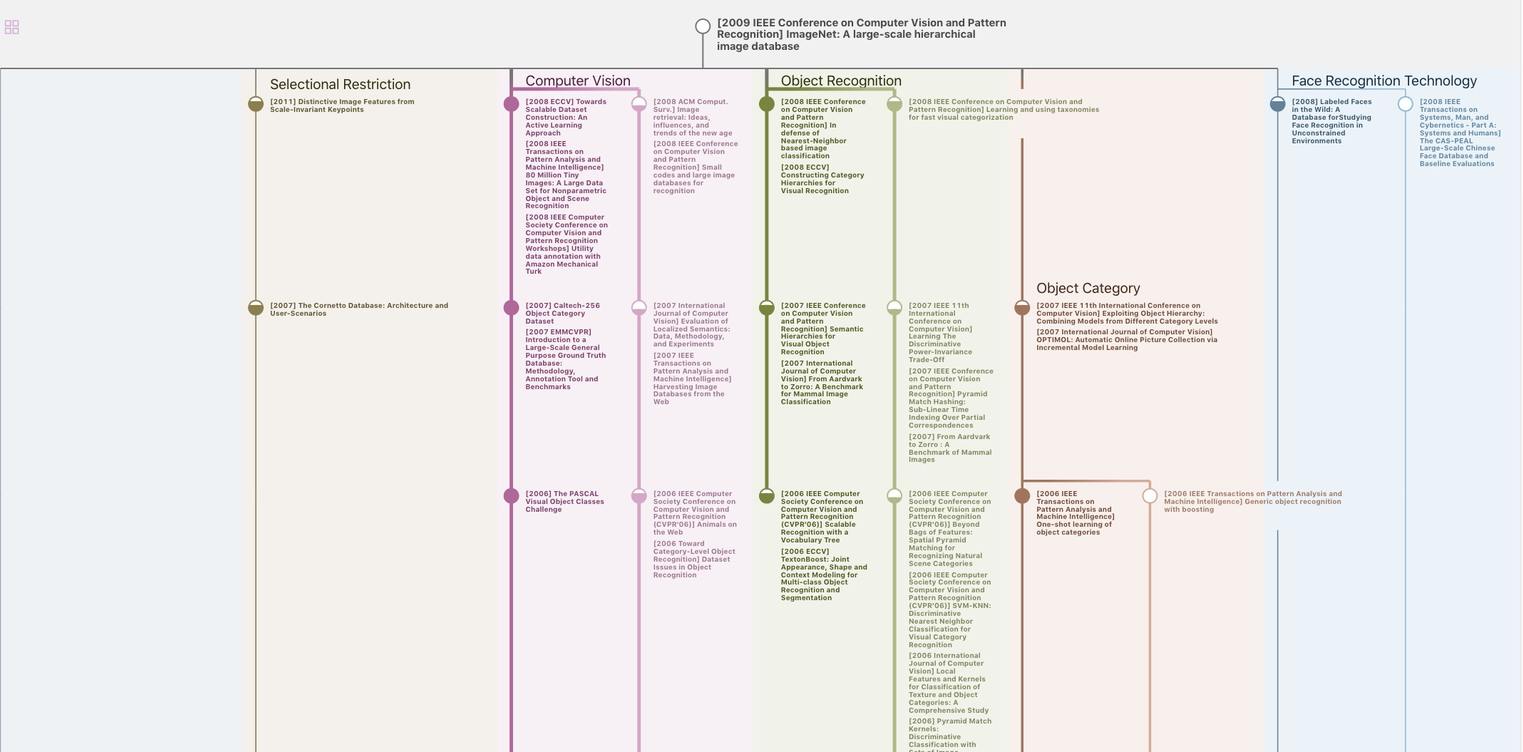
生成溯源树,研究论文发展脉络
Chat Paper
正在生成论文摘要