A novel ensemble CNN model for COVID-19 classification in computerized tomography scans
Results in Control and Optimization(2023)
摘要
COVID-19 is a rapidly spread infectious disease caused by a severe acute respiratory syndrome that can lead to death in just a few days. Thus, early disease detection can provide more time for successful treatment or action, even though an efficient treatment is unknown so far. In this context, this work proposes and investigates four ensemble CNNs using transfer learning and compares them with state-of-art CNN architectures. To select which models to use we tested 11 state-of-art CNN architectures: DenseNet121, DenseNet169, DenseNet201, VGG16, VGG19, Xception, ResNet50, ResNet50v2, InceptionV3, MobileNet, and MobileNetv2. We used a public dataset comprised of 2477 computerized tomography images divided into two classes: patients diagnosed with COVID-19 and patients with a negative diagnosis. Then three architectures were selected: DenseNet169, VGG16, and Xception. Finally, the ensemble models were tested in all possible combinations. The results showed that the ensemble models tend to present the best results. Moreover, the best ensemble CNN, called EnsenbleDVX, comprising all the three CNNs, provides the best results achieving an average accuracy of 97.7%, an average precision of 97.7%, an average recall of 97.8%, and an F1 average score of 97.7%
更多查看译文
关键词
COVID-19,CT image,Transfer learning,Ensemble,CNN
AI 理解论文
溯源树
样例
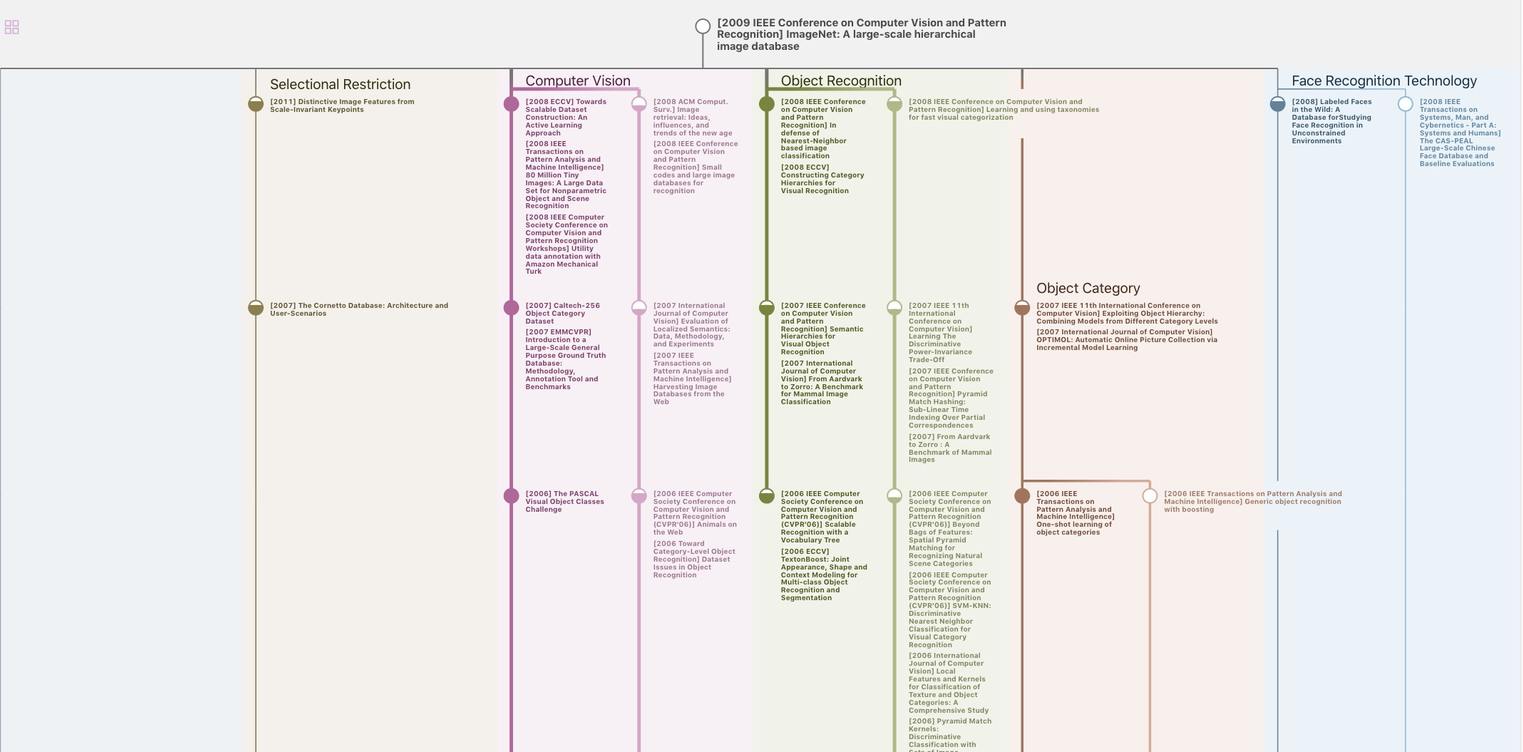
生成溯源树,研究论文发展脉络
Chat Paper
正在生成论文摘要