Optimization of SVM and ANN Based on GAPA and Its Application in Short-Term Load Forecasting
2022 IEEE International Conference on Energy Internet (ICEI)(2022)
摘要
Widespread employment of renewable energy such as wind and solar pushes power grids to move towards comprehensive data and predictive analysis. At present, a large number of researches have been conducted especially on machine learning methods to achieve load forecast. However, premature convergence and redundant iteration are two major defects of existing machine learning-based load forecasting methods, resulting in poor prediction effect and high time consumption. In this paper, a novel combined intelligent optimization algorithm based on genetic algorithm (GA), artificial fish swarm algorithm (AFSA) and particle swarm optimization (PSO) is proposed for optimizing machine learning-based load forecasting models. By replacing GA's mutation process with AFSA operator and PSO operator, the proposed algorithm named GA-AFSA-PSO Algorithm (GAPA) enhances both global search ability and local search ability, leading to its high prediction accuracy and fast convergence speed. To validate its effectiveness, GAPA is applied to the optimization of support vector machine (SVM) and artificial neural network (ANN) to predict one-day ahead load data. Moreover, two different sets of comparative tests are carried out to confirm the advantages of GAPA. The simulation results illustrate that, compared with GA, AFSA, PSO, AFSA-GA and GA-PSO, GAPA brings forth advancement in prediction accuracy, convergence rate and global search ability.
更多查看译文
关键词
load forecasting,intelligent optimization algorithm,GA,AFSA,PSO
AI 理解论文
溯源树
样例
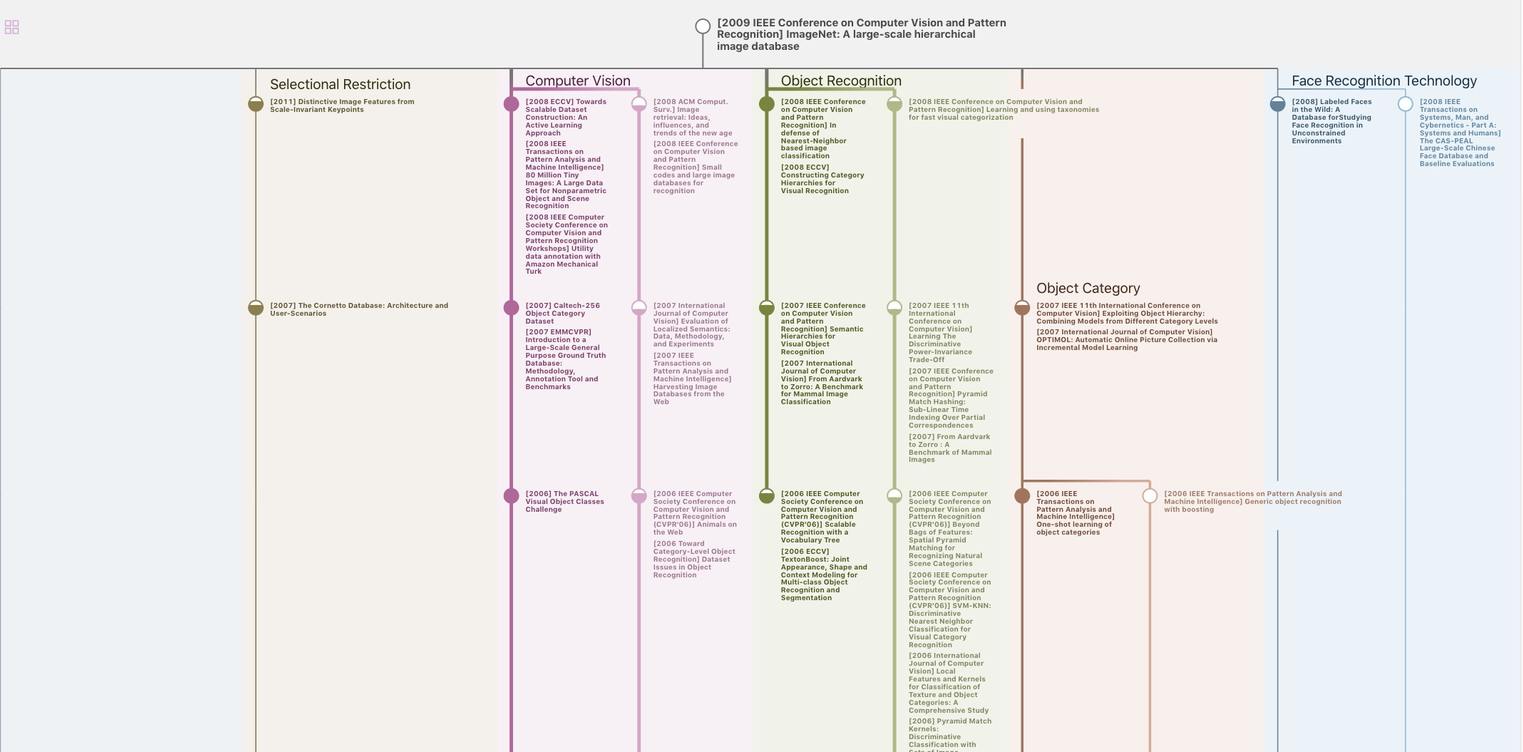
生成溯源树,研究论文发展脉络
Chat Paper
正在生成论文摘要