Net Load Segmented Forecasting Method For Data Center Based on GS-LightGBM Model
2023 IEEE IAS Global Conference on Renewable Energy and Hydrogen Technologies (GlobConHT)(2023)
摘要
The time-space transferability of data center workload determines that its power load has considerable adjustable potential. Making full use of this potential to formulate power load transfer strategy and participating in demand response or electricity market can effectively reduce the operating cost of data center. Accurate load forecasting results of data center are important supports for formulating effective transfer strategy. However, existing data center load forecasting methods still do not fully consider the influencing factors of different types of loads in the data center. And they are inapplicable when behind-the-meter (BTM) distributed photovoltaics (DPVs) are installed in the data center. To solve this, this paper divides the data center net load into two parts and forecasting them respectively: the cooling load (CL) and the remaining net load (RNL), where RNL is the sum of IT load, DPV output and other power loads. According to the characteristics of CL and DPV, we divided CL and RNL into seasons and periods respectively. Under each season or period, feature engineering based on light gradient boosting machine (LightGBM) is created and the LightGBM is also used to establish the forecasting model. The selection of parameters is realized by grid search algorithm (GS). Finally, the validity of the method is verified by the load data provided by National Renewable Energy Laboratory (NREL).
更多查看译文
关键词
data center,net load forecasting,segmented modeling,feature engineering,LightGBM
AI 理解论文
溯源树
样例
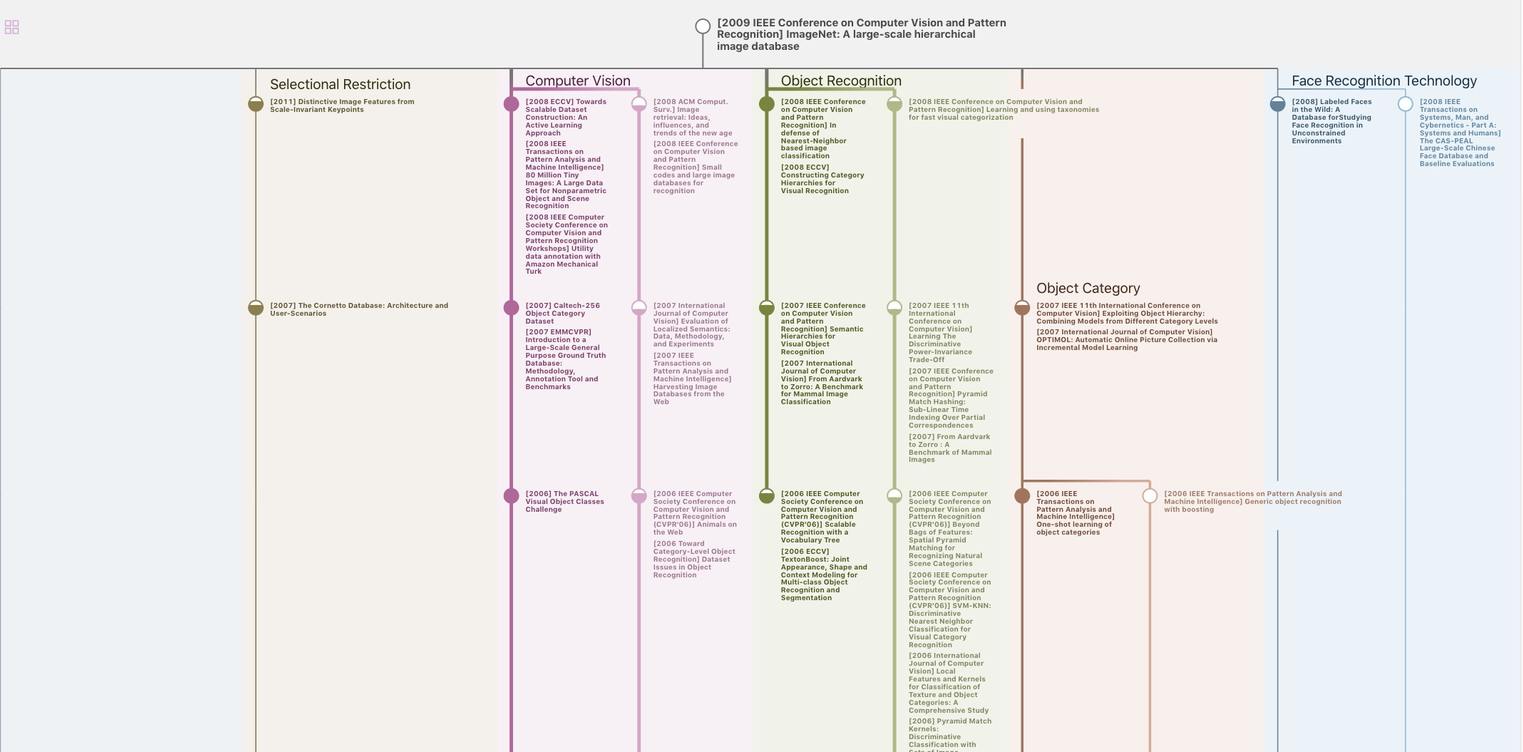
生成溯源树,研究论文发展脉络
Chat Paper
正在生成论文摘要