BCTNet: Bi-Branch Cross-Fusion Transformer for Building Footprint Extraction
IEEE Transactions on Geoscience and Remote Sensing(2023)
摘要
Building footprint extraction in remote sensing remains challenging due to the diverse appearances of buildings and confusing scenarios. Recently, researchers have revealed that both the globality and locality are vitally important in building footprint extraction tasks and proposed to incorporate the local context and global long-range dependency in the segmentation models. However, inadequate integration of the globality and locality still leads to incomplete, fake, or missing extraction results. To alleviate these problems, a novel segmentation method named bi-branch cross-fusion transformer network (BCTNet) is proposed in this study. Two parallel branches of the convolutional encoder branch (CB) and the transformer encoder branch (TB) are designed to extract multiscale feature maps. A concatenation-then-cross-fusion transformer block (CCTB) is put forward to integrate the locality from the CB and globality from the TB in a cross-fusion way at each stage of the encoding process. Then, an adaptive gating module (AGM) is proposed to gate the feature maps from the CCTB to strengthen the important features while suppressing irrelevant interference information. After that, the segmentation results can be obtained through a simple decoding process. Comprehensive experiments on two benchmark datasets demonstrate that the proposed BCTNet can achieve superior performance compared with the current state-of-the-art (SOTA) segmentation methods.
更多查看译文
关键词
Building footprint extraction,cross-fusion,gating mechanism,transformer
AI 理解论文
溯源树
样例
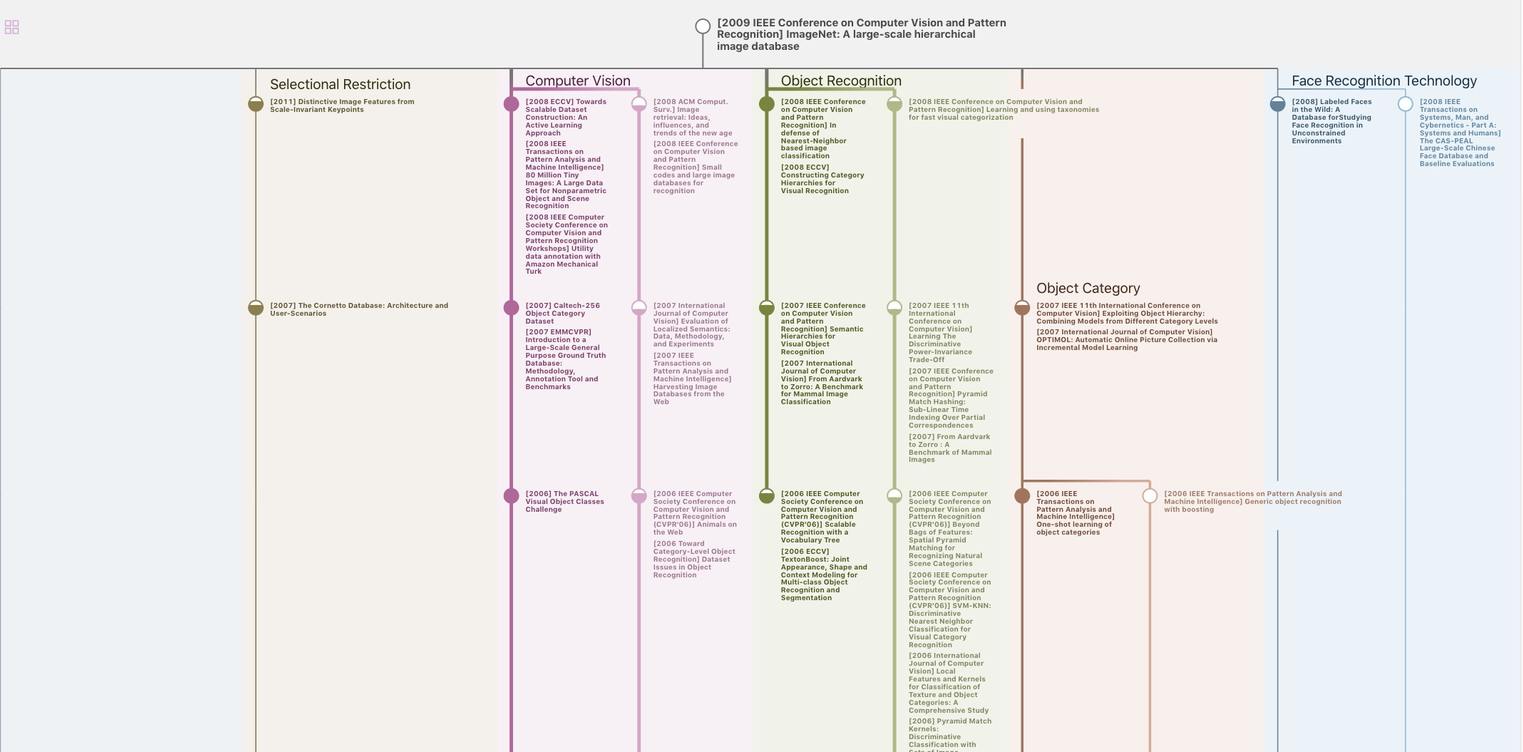
生成溯源树,研究论文发展脉络
Chat Paper
正在生成论文摘要