RoSI: Recovering 3D Shape Interiors from Few Articulation Images
CoRR(2023)
摘要
The dominant majority of 3D models that appear in gaming, VR/AR, and those we use to train geometric deep learning algorithms are incomplete, since they are modeled as surface meshes and missing their interior structures. We present a learning framework to recover the shape interiors (RoSI) of existing 3D models with only their exteriors from multi-view and multi-articulation images. Given a set of RGB images that capture a target 3D object in different articulated poses, possibly from only few views, our method infers the interior planes that are observable in the input images. Our neural architecture is trained in a category-agnostic manner and it consists of a motion-aware multi-view analysis phase including pose, depth, and motion estimations, followed by interior plane detection in images and 3D space, and finally multi-view plane fusion. In addition, our method also predicts part articulations and is able to realize and even extrapolate the captured motions on the target 3D object. We evaluate our method by quantitative and qualitative comparisons to baselines and alternative solutions, as well as testing on untrained object categories and real image inputs to assess its generalization capabilities.
更多查看译文
关键词
3d shape interiors,few articulation images
AI 理解论文
溯源树
样例
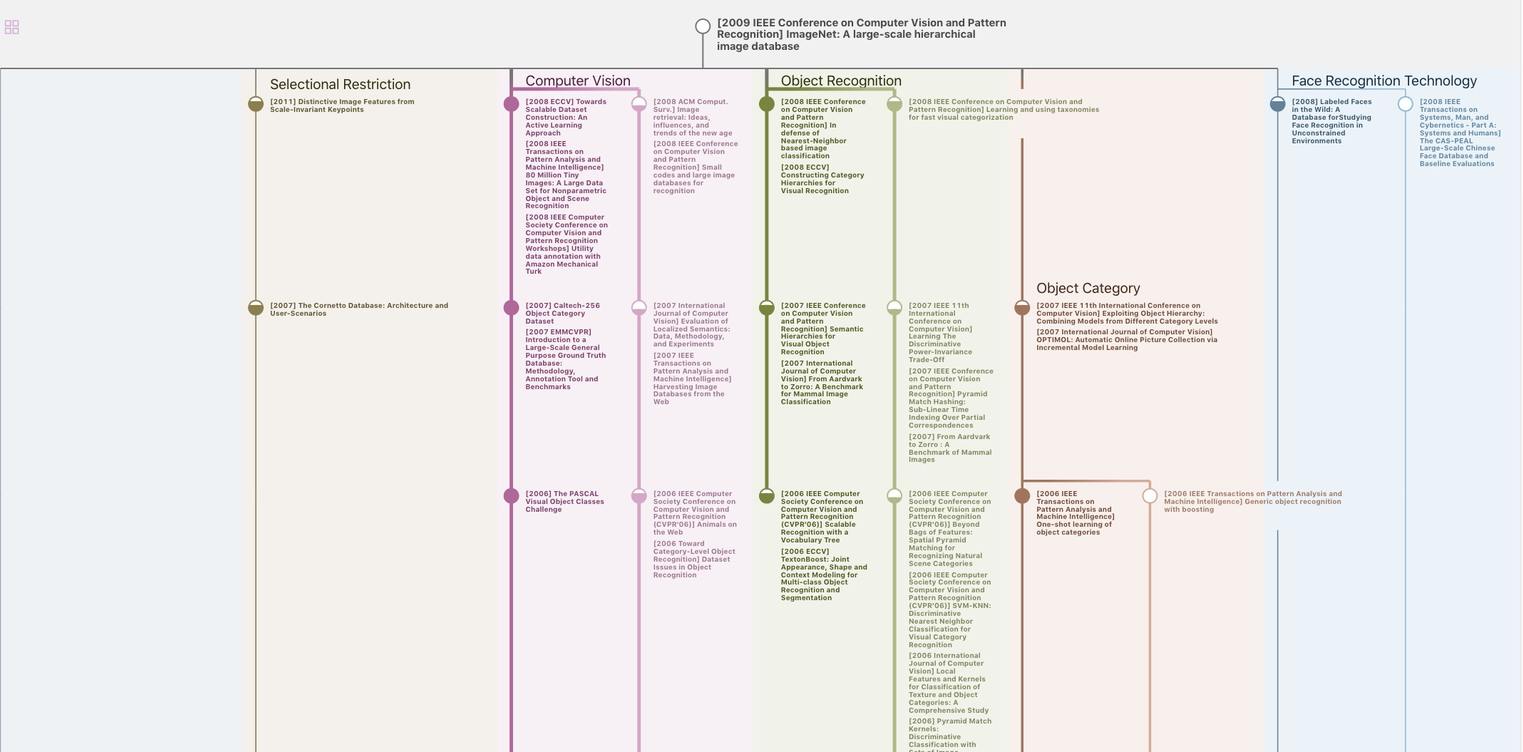
生成溯源树,研究论文发展脉络
Chat Paper
正在生成论文摘要