Utilizing metabolite connectivity and G-CNN to detect gallbladder cancer
2023 1st International Conference on Advanced Innovations in Smart Cities (ICAISC)(2023)
摘要
The hallmark of cancer is metabolic remodelling, which offers a unique insight into the biology of the disease revealing an opportunity for gallbladder cancer identification. By conceptualising the diagnosis of gallbladder cancer as a classification problem, it is possible to assess the capacity of several Machine Learning (ML) models to distinguish between gallbladder cancer patients and healthy individuals. Some of the models that are widely used in metabolomics literature include Support Vector Machines (SVM), Random Forest (RF) classifiers, Artificial Neural Networks (ANN), and Partial Least Squares-Discriminant Analysis (PLS-DA). The use of these models with metabolomic data has been successful. However, whether using them for classification tasks is preferable to using other or more complicated ML models has not been explored. We investigate a novel strategy for classifying people with gallbladder cancer by applying a Graph-Convolutional Neural Network (G-CNN) model to a dataset of patients. The methodical biological model of metabolite interactions and pathways as links is the foundation for this approach. Our findings show that the G-CNN significantly outperforms the most commonly used techniques, in contrast to the RF, whose effectiveness is marginally less but still equivalent to our model. Our findings suggest that using G-CNNs in metabolomics research is promising, and the adoption of non-invasive methods of metabolomic data extraction may help identify gallbladder cancer. Our suggested method achieves an accuracy of 93.6%, a specificity of 97%, and an AUC of 99.2%.
更多查看译文
关键词
Cancer,Diagnosis,Graph-Convolutional Neural Network,Gallbladder,Metabolic
AI 理解论文
溯源树
样例
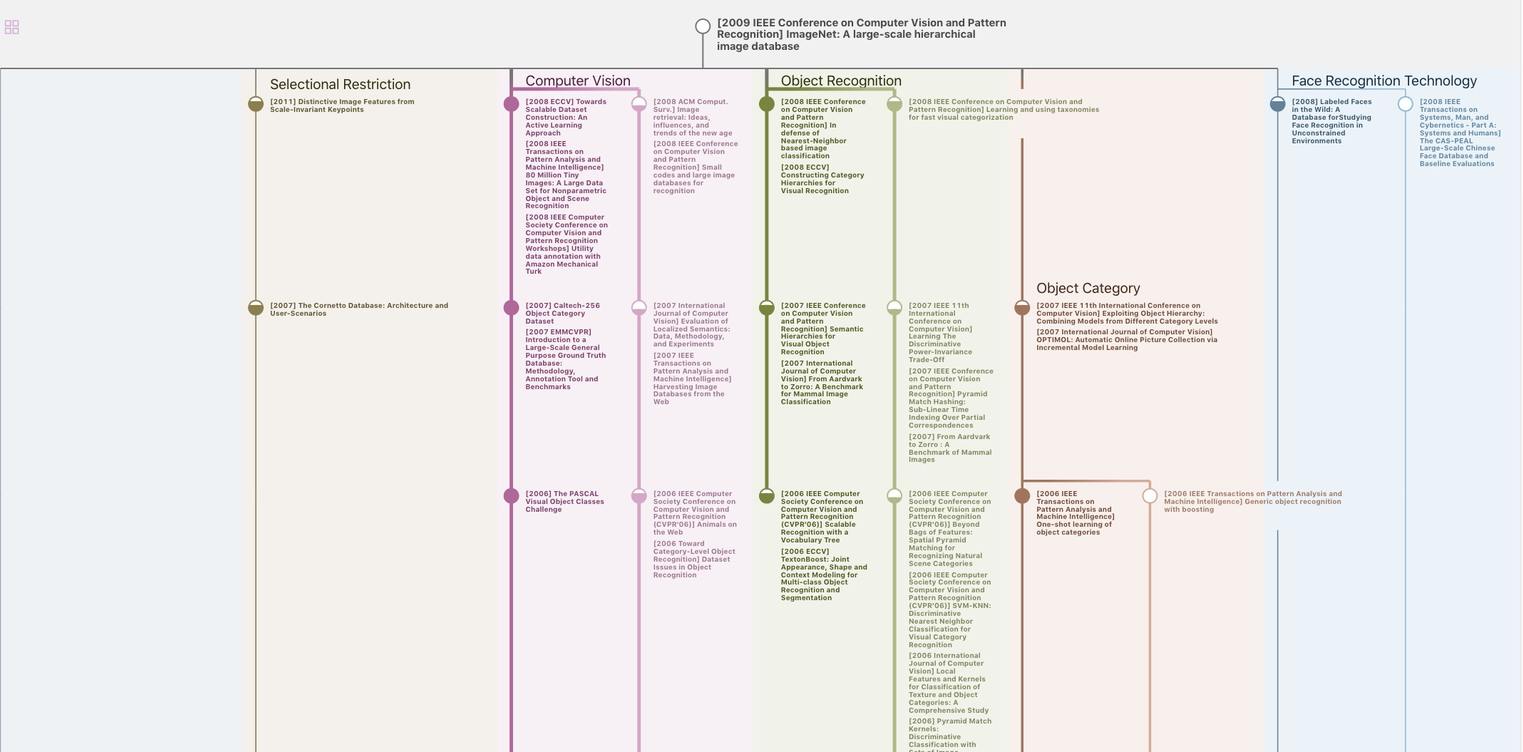
生成溯源树,研究论文发展脉络
Chat Paper
正在生成论文摘要