Shuffle Differential Private Data Aggregation for Random Population
IEEE Transactions on Parallel and Distributed Systems(2023)
摘要
Bridging the advantages of differential privacy in both centralized model (i.e., high accuracy) and local model (i.e., minimum trust), the shuffle privacy model has potential applications in many privacy-sensitive scenarios, such as mobile user data aggregation and federated learning. Since messages from users are anonymized by semi-trusted shufflers (e.g., anonymous channels, edge servers), every user could hide message among other users’ messages and inject only part of noises (a.k.a. privacy amplification). However, existing works assume that the participating user population is known in advance, which is unrealistic for dynamic environments (e.g., mobile computing, vehicular networks). In this work, we study the shuffle privacy model with a random participating population, and give privacy amplification bounds for population size with commonly encountered binomial, Poisson, sub-Gaussian distribution and etc. For further improving accuracy, we formulate and derive optimal dummy sizes for both non-adaptive and adaptive dummies. Finally, to break the error barrier due to the constraint of sending one single message per user, we design a multi-message shuffle private protocol supporting random population. Experiment results show that our approaches reduce more than 60% error when compared to the local model and naive approaches. We hope this work provides tailored solutions of shuffle privacy for dynamic mobile/distributed computing.
更多查看译文
关键词
Data aggregation,data privacy,differential privacy,shuffle privacy,statistical estimation
AI 理解论文
溯源树
样例
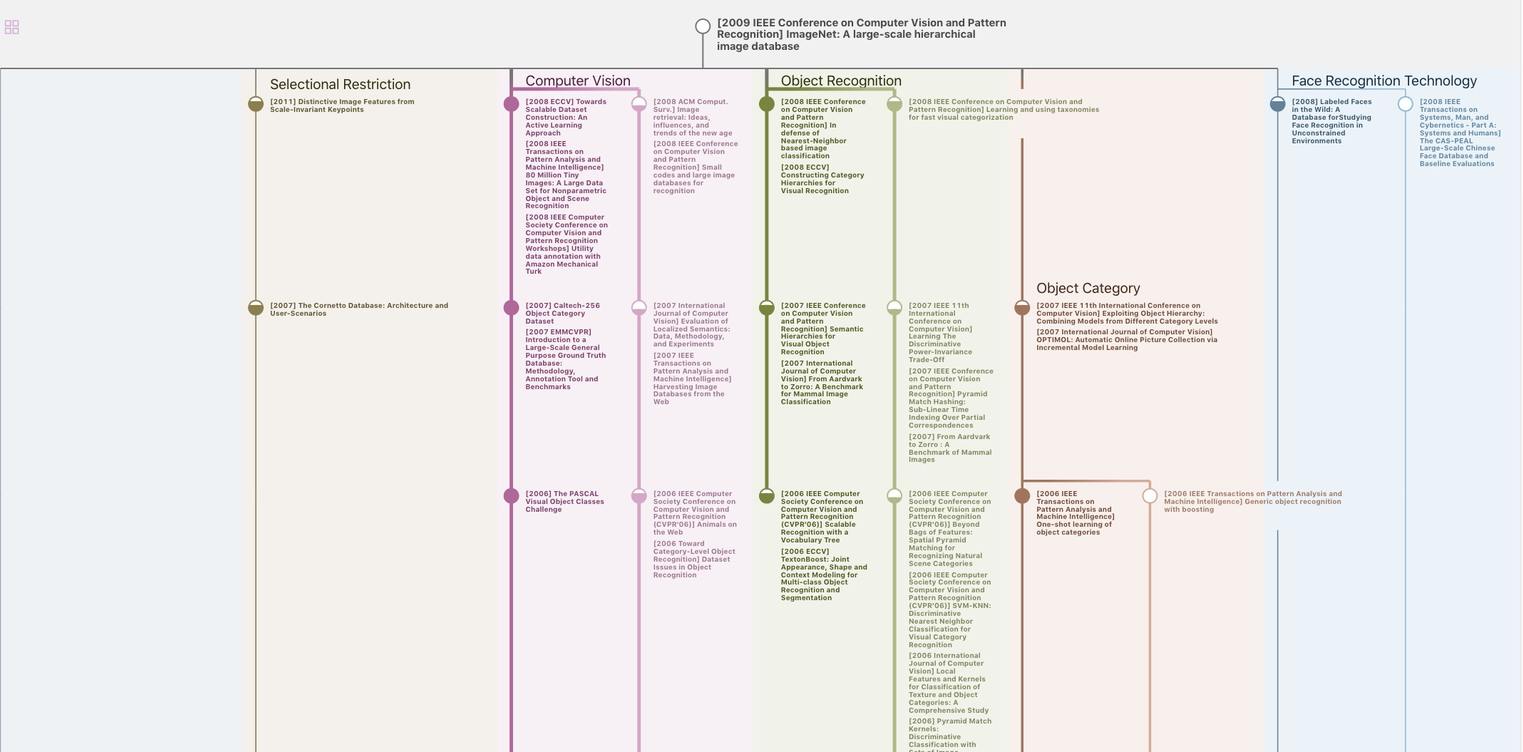
生成溯源树,研究论文发展脉络
Chat Paper
正在生成论文摘要