CAML: Contextual augmented meta-learning for cold-start recommendation
Neurocomputing(2023)
摘要
The performance of recommendation engines is challenged by the cold-start issues. Classical recommendation techniques have limited capability to address this problem because the underlying machine learning models are data-thirsty. Conversely, the recent success of meta-learning attracted a lot of attention due to few-shot learning capabilities which can be employed to accommodate new tasks even when there are few data samples for training. Most of the meta-learning recommendation models adopt model-agnostic meta-learning to initialize parameters that may lead to stuck into local optima instead of global optima for some users. To leverage the learning process, we propose a Contextually Augmented Meta-Learning recommender system (CAML). The proposed method augments the contextual features into a meta-learning model which considerably improves the tasks adaptation capability. We constructed a Data Augmentation Unit (DAU) that used a hybrid similarity to augment data samples of similar neighbors. The augmented samples are then forwarded to a Meta-Learner (MetaL) to learn user preferences and generate relevant recommendations. Additionally, we highlighted that the proposed method is generalized and can be adapted for various meta-learning-based recommendation models. We validate our proposal on three benchmark datasets for both ranking and rating prediction perspectives. The experimental outcomes prove the significance of our proposal over the state-of-the-art recommendation methods.
更多查看译文
关键词
Data augmentation,Meta-learning,Cold-start,Collaborative filtering,Context-aware recommender systems
AI 理解论文
溯源树
样例
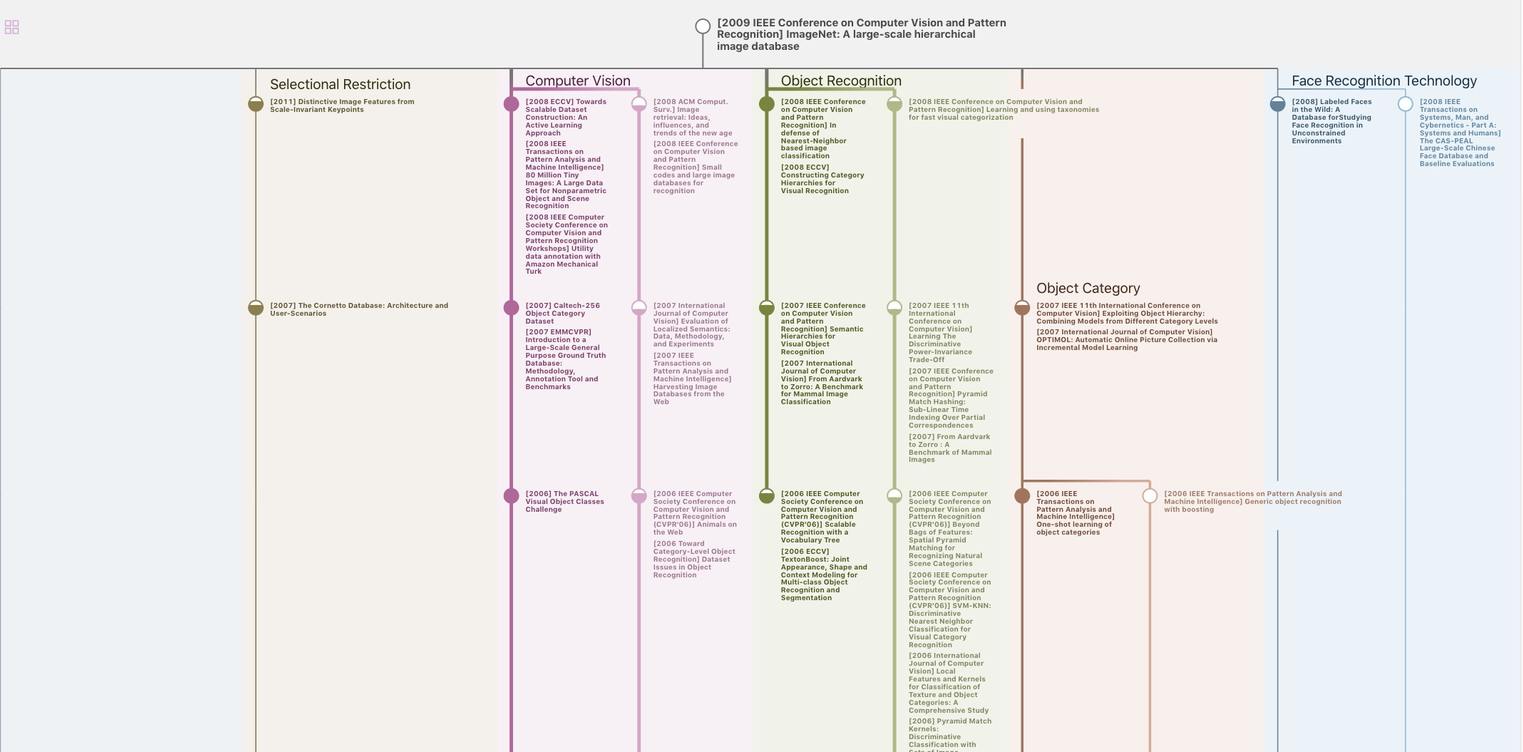
生成溯源树,研究论文发展脉络
Chat Paper
正在生成论文摘要