Fake Reviews Classification using Deep Learning
2023 International Multi-disciplinary Conference in Emerging Research Trends (IMCERT)(2023)
摘要
Customer decisions are heavily influenced by online reviews. Scammers and spammers can now influence consumer behavior by spreading false information in the form of reviews, either by promoting nonexistent goods or by disparaging rival goods. This means that identifying bogus from genuine reviews is more crucial than ever. For text classification, the standard method employs a bag-of-words model to represent text, leading to sparsity and word representations learned from neural networks with poor capacity for handling unknown words. In this work, we offer a method that uses an ensemble of models built using an aggregation methodology to make predictions based on data from three individual models trained using a multi-view learning approach. Our technology is based around a central idea of using bag-of-n-grams in conjunction with parallel convolution neural networks to extract valuable information from review text (CNNs). With the same amount of computing needed to train deep and sophisticated CNNs, we can leverage local context with an n-gram embedding layer that has tiny kernel sizes. In order to better extract feature representations from text, our CNN-based architecture takes n-gram embeddings as input and processes them with concurrent convolutional blocks. In addition to including linguistic aspects of the review text and non-textual information associated with reviewer behavior, our method for identifying fraudulent reviews also considers reviewer activity. We test our method using the openly available Yelp Filtered Dataset, and get F1 scores as high as 92% for recognizing fraudulent reviews.
更多查看译文
关键词
Convolutional networks,Deep learning in NLP,Ensemble models,Fake reviews detection,Information retrieval,Social behavior
AI 理解论文
溯源树
样例
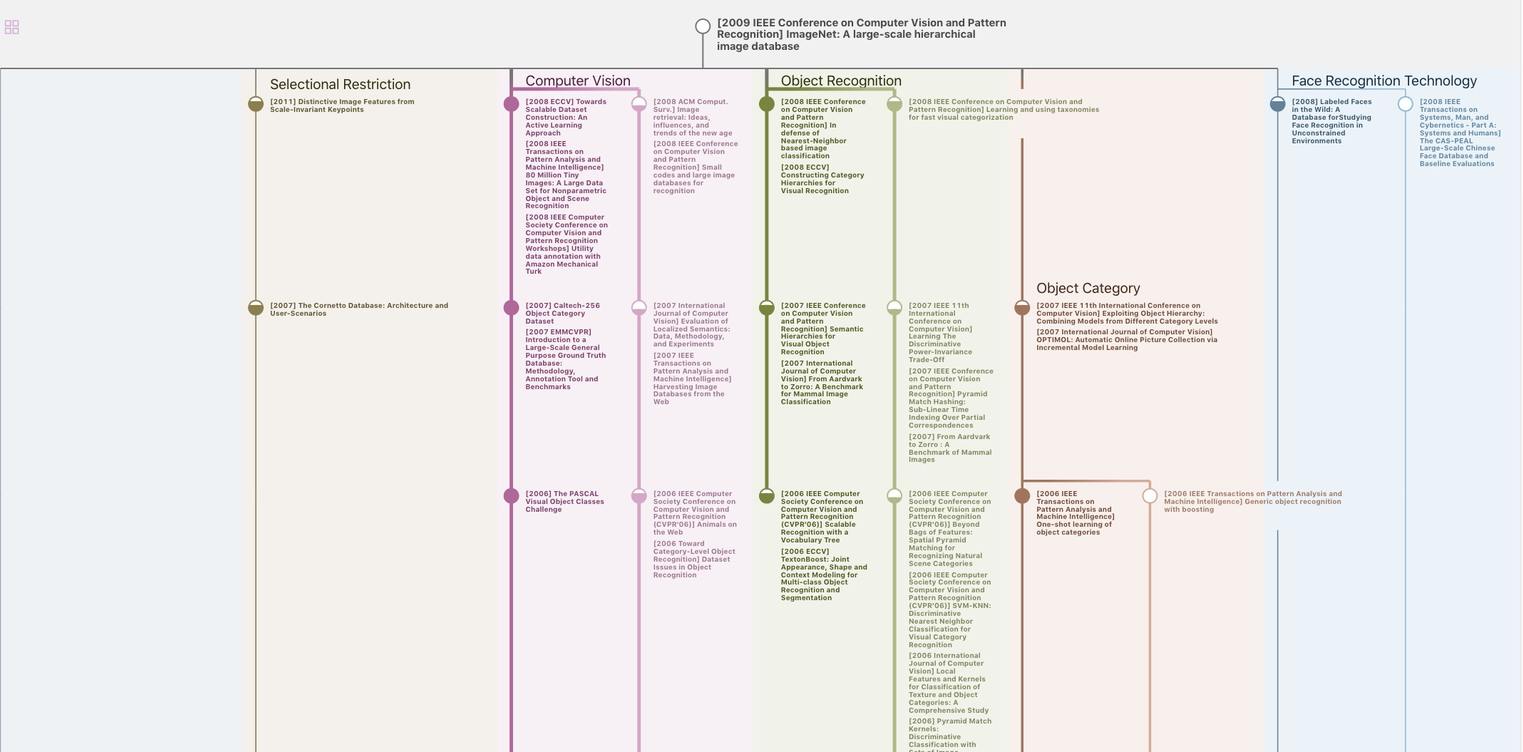
生成溯源树,研究论文发展脉络
Chat Paper
正在生成论文摘要