Cluster Analysis Identified Clinically Relevant Metabolic Syndrome Endophenotypes
medrxiv(2022)
摘要
Aims/hypothesis Metabolic syndrome (MetS) is a collection of cardiovascular risk factors; however, the high prevalence and heterogeneity impede proper and effective clinical management of MetS. In order for precision medicine to work for MetS, we aimed to identify clinically relevant MetS sub-phenotypes.
Methods We conducted cluster analysis on individuals from UK Biobank based on MetS criteria to reveal endophenotypes, identified the corresponding cardiometabolic traits and established the association across 21 clinical outcomes. Genome-wide association studies were conducted to identify associated genotypic traits. We further compared the genotypic traits to reveal endophenotypes-specific genotypic traits. Lastly, potential drug targets were identified for the different endophenotypes.
Results Five MetS subgroups were identified which were Cluster 1 (C1): non-descriptive, Cluster 2 (C2): hypertensive, Cluster 3 (C3): obese, Cluster 4 (C4): lipodystrophy-like, and Cluster 5 (C5): hyperglycaemic. Some MetS clusters had higher CVD risks such as C1 (OR=6·765) and C5 (OR=9·486). Despite being non-descriptive across all cardiometabolic traits, C1 had higher risks for most clinical outcomes. MetS clusters also had different risks to various types of cancers. GWAS of each MetS clusters revealed different genotypic traits. LPCAT2 was associated with all clusters except C2 and expression is specific to immune cells. C1 GWAS revealed novel findings of TRIM63, MYBPC3, MYLPF , and RAPSN . Intriguingly, C1, C3, and C4 were associated with genes highly expressed in brain tissues: CN1H2, TMEM151A, MT3 , and C1QTNF4 . The cluster-specific genotypic traits also revealed potential drug repurposing targets specific to the endophenotypes.
Conclusion/interpretation MetS is highly heterogeneous with endophenotypes that are different in terms of phenotypic and genotypic traits. GWAS of subgroups revealed novel cardiometabolic genotypes which were masked by heterogeneity of MetS.
Evidence before this study We searched PubMed, Science Direct and Scopus from 1st January 2012 to 30th September 2022 for “unsupervised learning” or “clustering” or “endophenotype” or “subclassifications” or “sub-phenotype” and “metabolic syndrome” or “complex diseases”. Google Scholar, UK Biobank published work and approved research were also searched for similar study. This search only revealed published work in other complex diseases such as T2D (which is heavily referenced in our manuscript), Alzheimer’s diseases, psychiatric diseases, and asthma. None of the previously published work applied the combination of unsupervised learning and GWAS for identification of clinically relevant endophenotypes in metabolic syndrome or any complex diseases.
Added value of this study Metabolic syndrome (MetS) is a known cardiovascular disease risk factor, however the constantly changing MetS criteria and high prevalence of MetS impede proper clinical management of individuals with MetS. Through clustering, we identified MetS endophenotypes with semi-distinctive cardiometabolic traits. Some of the MetS endophenotypes correspond with T2D subgroups discovered by other research groups. However, our endophenotypes are more clinically relevant, due to the differing clinical risks across 21 clinical outcomes. We also identified a non-descriptive MetS subgroup with strikingly high cardiovascular risk which likely to be overlooked in clinical settings. Through genome-wide association studies, our endophenotypes also revealed interesting insights into the genetic causes and biological pathways of MetS. We were able to identified genotypic traits that are unique to each MetS endophenotypes and shared genotypic traits which highlights the common pathophysiology underlying MetS. Lastly, we were also able to reveal potential drug targets for drug repurposing, some drug targets are unique to specific endophenotypes.
Implications of all the available evidence Our study attempted to resolve the issue of MetS heterogeneity, by revealing clinically relevant endophenotypes which might respond to different pharmacotherapy. Furthermore, our findings challenge the “one size fits all” step-wise approach in managing complex diseases, emphasizing tailored treatment for different subgroups of patients, a key step towards precision medicine in clinical practice.
![Figure][1]
### Competing Interest Statement
The authors have declared no competing interest.
### Funding Statement
This project is supported by MOST 111-2314-B-001-008 grant from Ministry of Science and Technology of Taiwan.
### Author Declarations
I confirm all relevant ethical guidelines have been followed, and any necessary IRB and/or ethics committee approvals have been obtained.
Yes
I confirm that all necessary patient/participant consent has been obtained and the appropriate institutional forms have been archived, and that any patient/participant/sample identifiers included were not known to anyone (e.g., hospital staff, patients or participants themselves) outside the research group so cannot be used to identify individuals.
Yes
I understand that all clinical trials and any other prospective interventional studies must be registered with an ICMJE-approved registry, such as ClinicalTrials.gov. I confirm that any such study reported in the manuscript has been registered and the trial registration ID is provided (note: if posting a prospective study registered retrospectively, please provide a statement in the trial ID field explaining why the study was not registered in advance).
Yes
I have followed all appropriate research reporting guidelines and uploaded the relevant EQUATOR Network research reporting checklist(s) and other pertinent material as supplementary files, if applicable.
Yes
Analyses in this study was conducted under UKB application ID 46789. The genetic and phenotype datasets are not publicly available but can be accessed via the UK Biobank data access process ( ). GWAS summary statistics are available on GWAS Catalog ( ).
* AF
: Atrial fibrillation
ALB
: Albumin
ALP
: Alkaline phosphatase
ApoA
: Apolipoprotein A
ApoB
: Apolipoprotein B
AST
: Aspartate aminotransferase
AVI
: Abdominal volume index
BFP
: Body fat percentage
BMI
: Body mass index
BMR
: Basal metabolic rate
CrCl
: Creatine clearance
CRP
: C-reactive protein
CVD
: Cardiovascular diseases
DBP
: Diastolic blood pressure
FEV1
: Forced expiratory volume in 1 second
FVC
: Forced vital capacity
GGT
: Gamma-glutamyl transferase
GWAS
: Genome-wide association study
Hb
: Haemoglobin
HC
: Hip circumference
HDL
: High density lipoprotein
LDL
: Low density lipoprotein
LpA
: Lipoprotein A
MAP
: Mean arterial pressure
MetS
: Metabolic syndrome
OR
: Odds ratio
PEF
: Peak expiratory flow
PP
: Pulse pressure
PR
: Pulse rate
SBP
: Systolic blood pressure
SNP
: Single nucleotide polymorphisms
TB
: Total bilirubin
TC
: Total cholesterol
TG
: Triglyceride
UKB
: UK Biobank
WHR
: Waist to hip ratio
WBC
: White blood count
WBFM
: Whole body fat mass
WI
: Waist index
[1]: pending:yes
更多查看译文
关键词
metabolic,syndrome
AI 理解论文
溯源树
样例
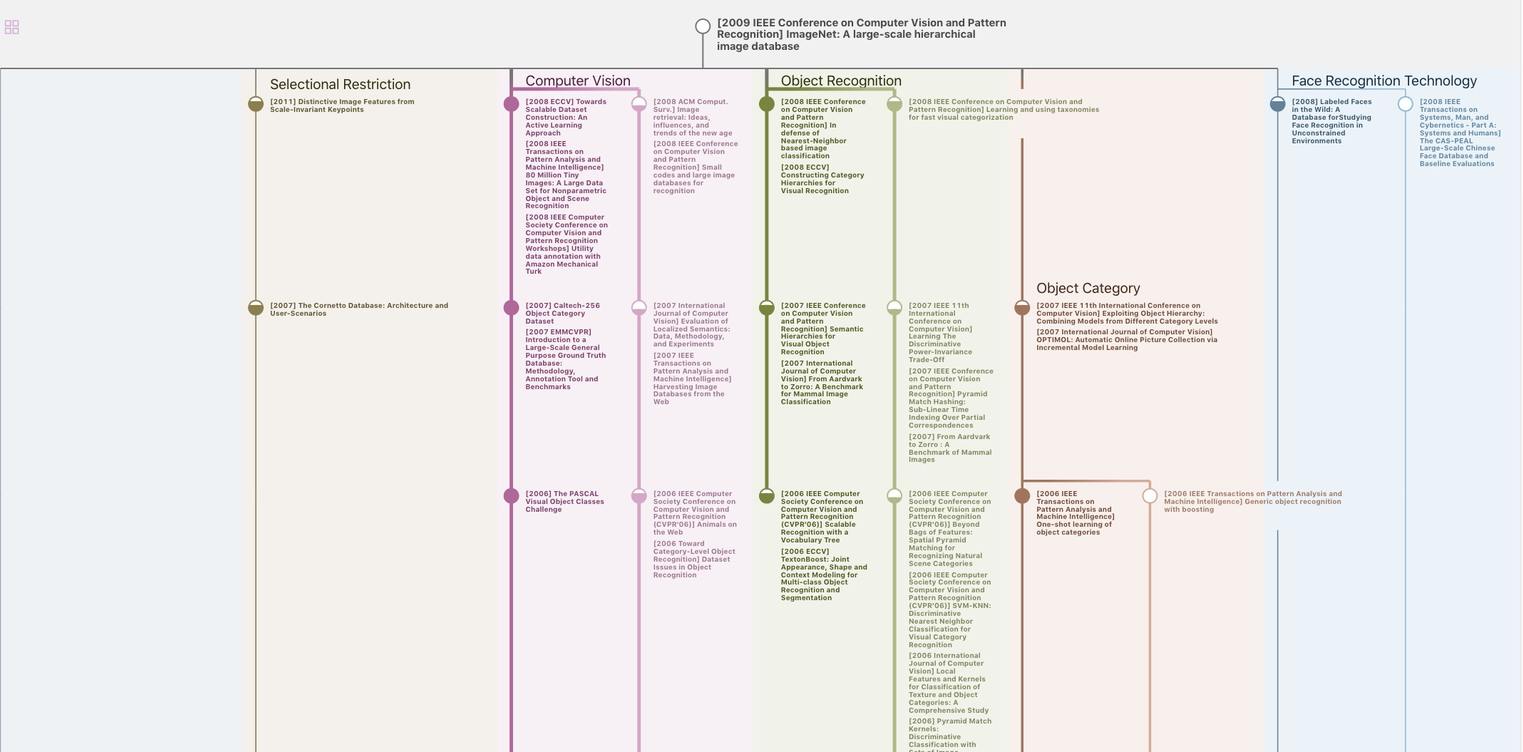
生成溯源树,研究论文发展脉络
Chat Paper
正在生成论文摘要