Predicting opioid use disorder before and after the opioid prescribing peak in the United States: A machine learning tool using electronic healthcare records
HEALTH INFORMATICS JOURNAL(2023)
摘要
Existing predictive models of opioid use disorder (OUD) may change as the rate of opioid prescribing decreases. Using Veterans Administration's EHR data, we developed machine-learning predictive models of new OUD diagnoses and ranked the importance of patient features based on their ability to predict a new OUD diagnosis in 2000-2012 and 2013-2021. Using patient characteristics, the three separate machine learning techniques were comparable in predicting OUD, achieving an accuracy of >80%. Using the random forest classifier, opioid prescription features such as early refills and length of prescription consistently ranked among the top five factors that predict new OUD. Younger age was positively associated with new OUD, and older age inversely associated with new OUD. Age stratification revealed prior substance abuse and alcohol dependency as more impactful in predicting OUD for younger patients. There was no significant difference in the set of factors associated with new OUD in 2000-2012 compared to 2013-2021. Characteristics of opioid prescriptions are the most impactful variables that predict new OUD both before and after the peak in opioid prescribing rates. Predictive models should be tailored to age groups. Further research is warranted to determine if machine learning models perform better when tailored to other patient subgroups.
更多查看译文
关键词
opioid prescribing peak,opioid use disorder,machine learning tool,machine learning
AI 理解论文
溯源树
样例
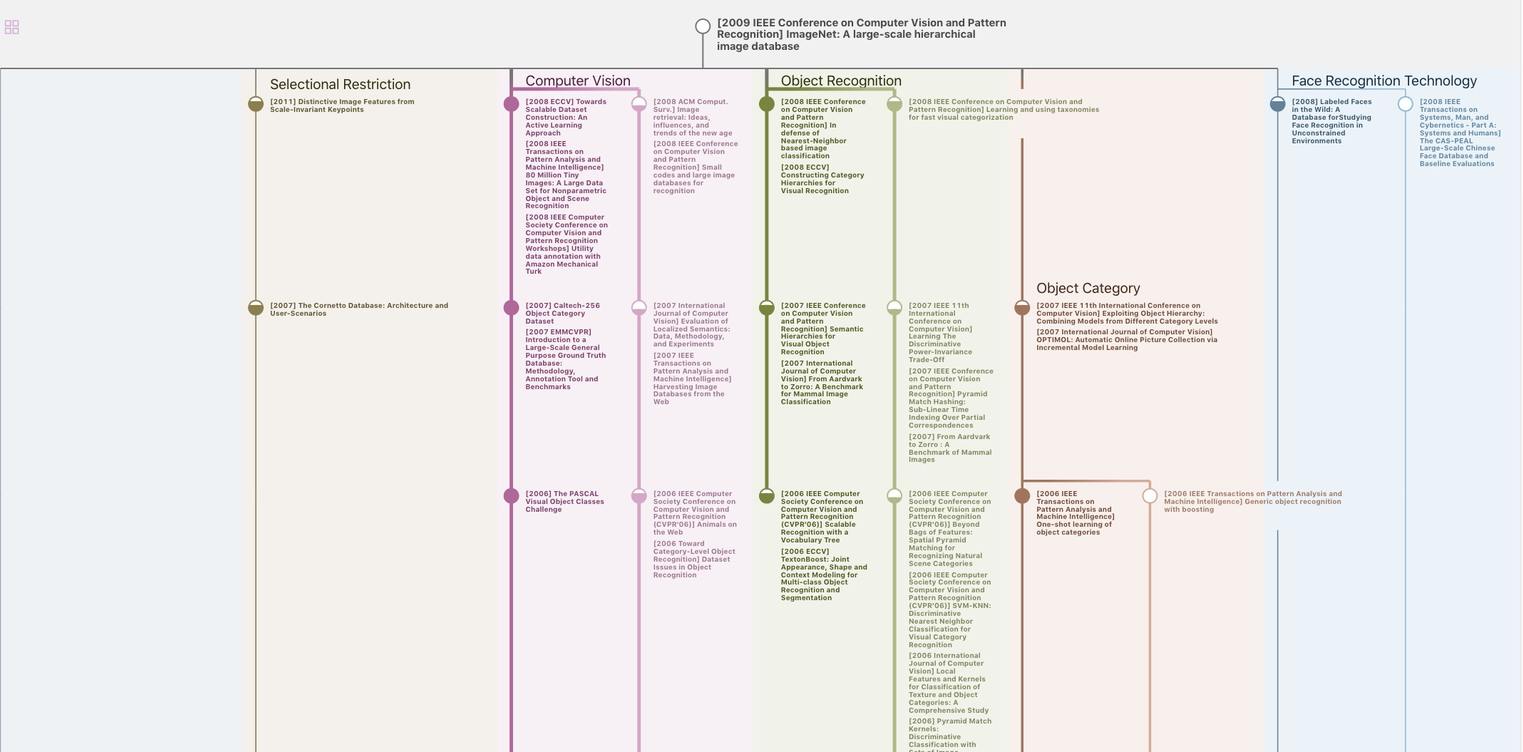
生成溯源树,研究论文发展脉络
Chat Paper
正在生成论文摘要