DiscoGen: Learning to Discover Gene Regulatory Networks
CoRR(2023)
摘要
Accurately inferring Gene Regulatory Networks (GRNs) is a critical and challenging task in biology. GRNs model the activatory and inhibitory interactions between genes and are inherently causal in nature. To accurately identify GRNs, perturbational data is required. However, most GRN discovery methods only operate on observational data. Recent advances in neural network-based causal discovery methods have significantly improved causal discovery, including handling interventional data, improvements in performance and scalability. However, applying state-of-the-art (SOTA) causal discovery methods in biology poses challenges, such as noisy data and a large number of samples. Thus, adapting the causal discovery methods is necessary to handle these challenges. In this paper, we introduce DiscoGen, a neural network-based GRN discovery method that can denoise gene expression measurements and handle interventional data. We demonstrate that our model outperforms SOTA neural network-based causal discovery methods.
更多查看译文
关键词
regulatory networks,gene,discover,learning
AI 理解论文
溯源树
样例
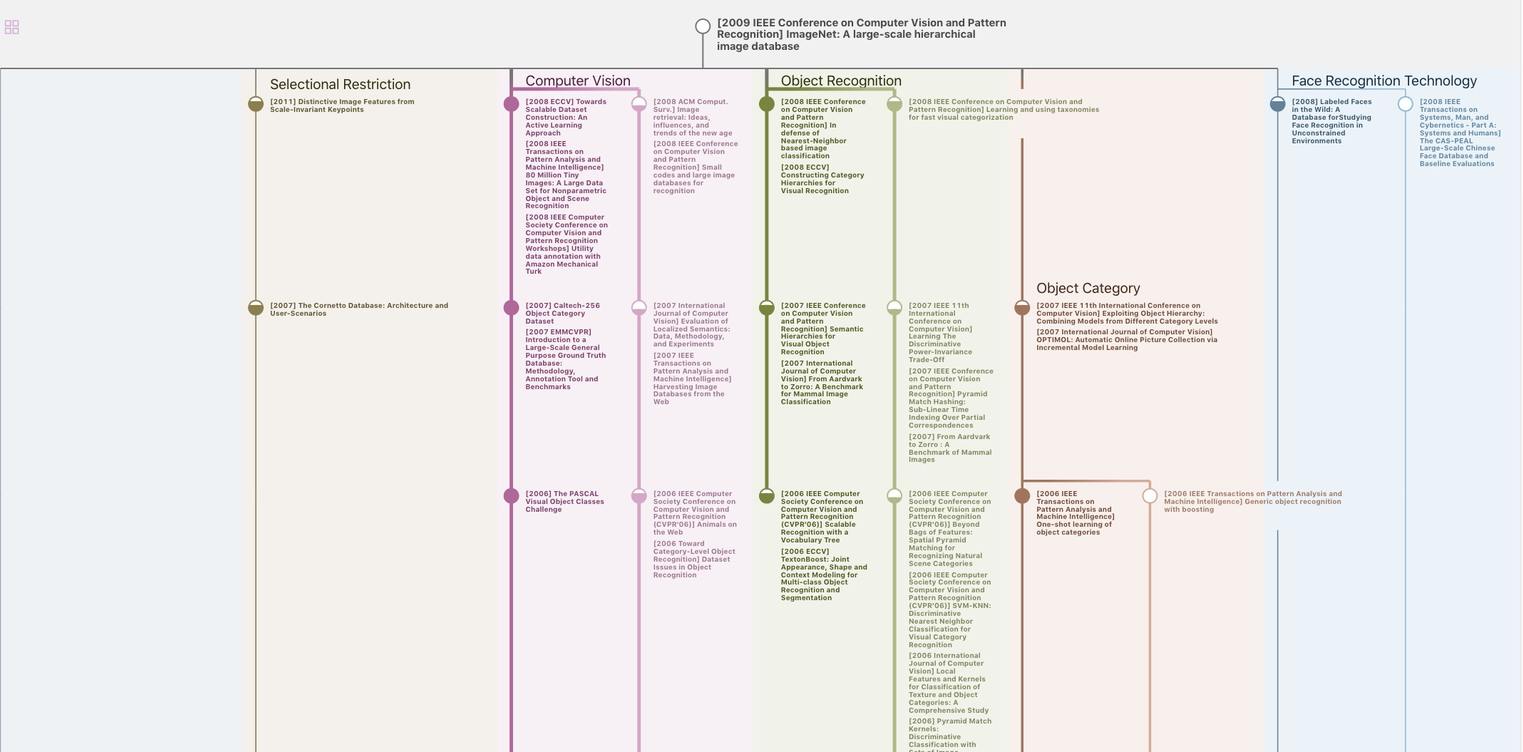
生成溯源树,研究论文发展脉络
Chat Paper
正在生成论文摘要