Scaling MadMiner with a deployment on REANA
arXiv (Cornell University)(2023)
摘要
MadMiner is a Python package that implements a powerful family of multivariate inference techniques that leverage matrix element information and machine learning. This multivariate approach neither requires the reduction of high-dimensional data to summary statistics nor any simplifications to the underlying physics or detector response. In this paper, we address some of the challenges arising from deploying MadMiner in a real-scale HEP analysis with the goal of offering a new tool in HEP that is easily accessible. The proposed approach encapsulates a typical MadMiner pipeline into a parametrized yadage workflow described in YAML files. The general workflow is split into two yadage sub-workflows, one dealing with the physics simulations and the other with the ML inference. After that, the workflow is deployed using REANA, a reproducible research data analysis platform that takes care of flexibility, scalability, reusability, and reproducibility features. To test the performance of our method, we performed scaling experiments for a MadMiner workflow on the National Energy Research Scientific Computer (NERSC) cluster with an HT-Condor back-end. All the stages of the physics sub-workflow had a linear dependency between resources or wall time and the number of events generated. This trend has allowed us to run a typical MadMiner workflow, consisting of 11M events, in 5 hours compared to days in the original study.
更多查看译文
关键词
reana,madminer,scaling
AI 理解论文
溯源树
样例
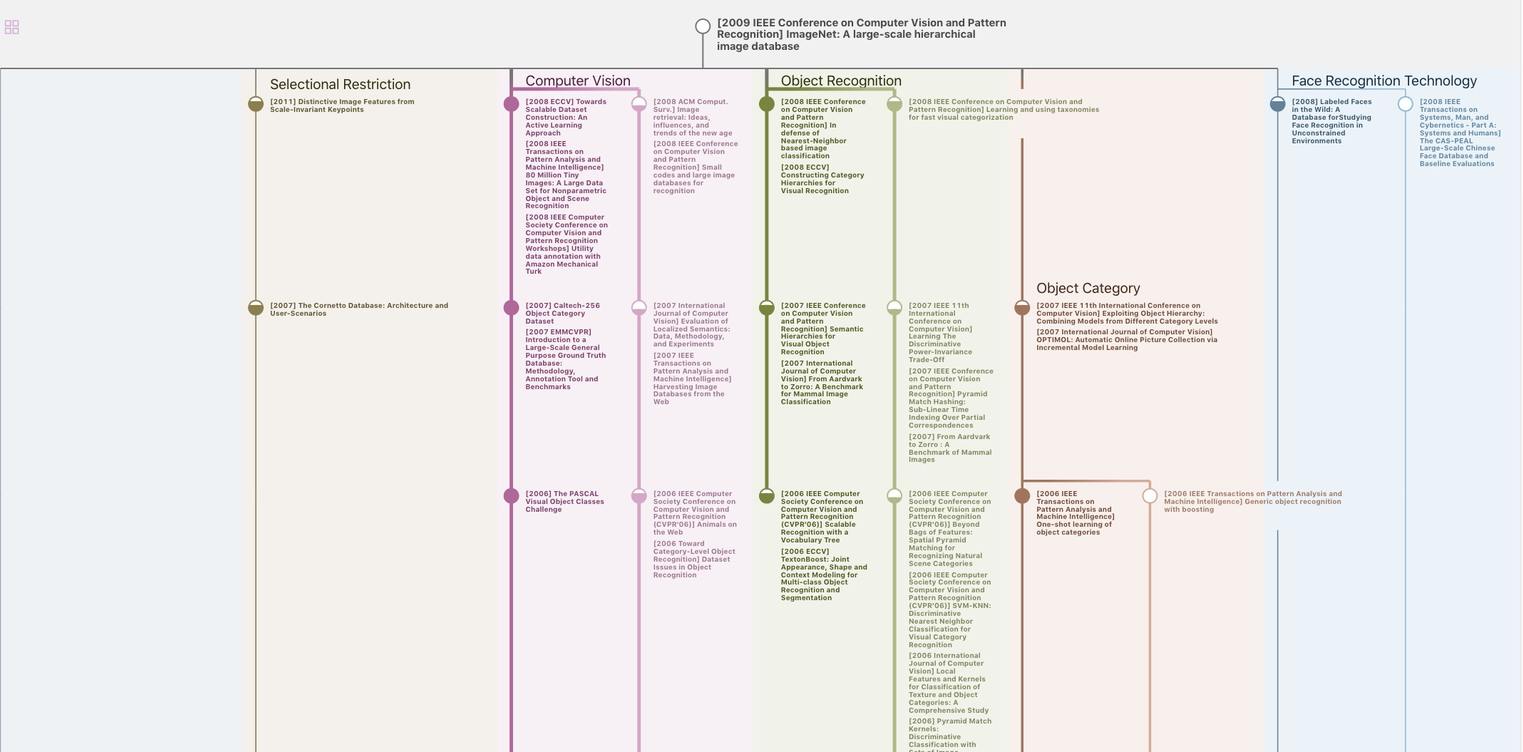
生成溯源树,研究论文发展脉络
Chat Paper
正在生成论文摘要