Mean Estimation Mechanisms under (e, (5)-Local Differential Privacy
Journal of Electronics & Information Technology(2023)
摘要
Compared with the e-Local Differential Privacy (LDP) mechanism, the scheme under (e, (5)-local differential privacy has a smaller error bound and higher data utility. However, the current mean estimation mechanisms under (e, (5)-local differential privacy still have problems such as large estimation error and low data utility. Therefore, for the mean estimation problem, two new mean estimation mechanisms under (e, (5) local differential privacy are proposed: the Interval-based Mechanism for mean estimation (IM) and the Neighbor-based Mechanism for mean estimation (NM). IM divides the perturbed data into three intervals. Then the real data is perturbed to the middle interval with a large probability, and the two sides are perturbed with a small probability. Collector averages directly the perturbed data to get an unbiased estimation. NM perturbs the real data to its near neighborhood with a large probability and perturbs it far away with a small probability. Then the collector applies the expectation maximization algorithm to obtain an estimated mean value with high accuracy. Finally, both IM and NM satisfy the privacy protection requirements are proved through theoretical analysis, and the data utility of IM and NM is superior to existing mechanisms is confirmed by experiments.
更多查看译文
AI 理解论文
溯源树
样例
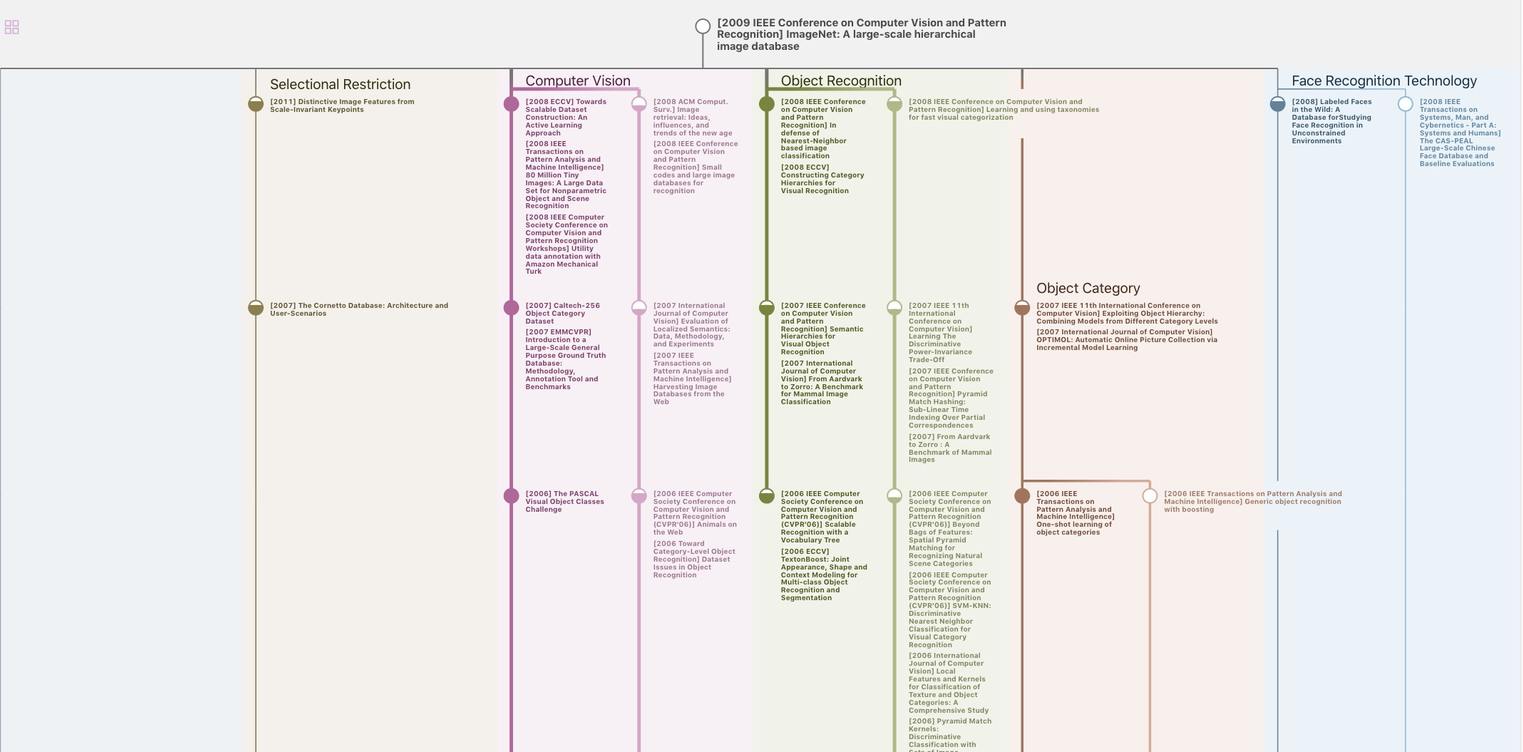
生成溯源树,研究论文发展脉络
Chat Paper
正在生成论文摘要