Knowledge Base Embedding for Sampling-Based Prediction
ACM Transactions on Information Systems(2023)
摘要
Each link prediction task requires different degrees of answer diversity. While a link prediction task may expect up to a couple of answers, another may expect nearly a hundred answers. Given this fact, the performance of a link prediction model can be estimated more accurately if a flexible number of obtained answers are estimated instead of a predefined number of answers. Inspired by this, in this article, we analyze two evaluation criteria for link prediction tasks, respectively ranking-based protocol and sampling-based protocol. Furthermore, we study two classes of models on link prediction task, direct model and latent-variable model respectively, to demonstrate that latent-variable model performs better under the sampling-based protocol. We then propose a latent-variable model where the framework of Conditional Variational AutoEncoder (CVAE) is applied. Experimental study suggests that the proposed model performs comparably to the current state-of-the-art even under the conventional rank-based protocol. Under the sampling-based protocol, the proposed model is shown to outperform various state-of-the-art models.
更多查看译文
关键词
Link prediction,Knowledge Base Embedding,Conditional Variational AutoEncoder
AI 理解论文
溯源树
样例
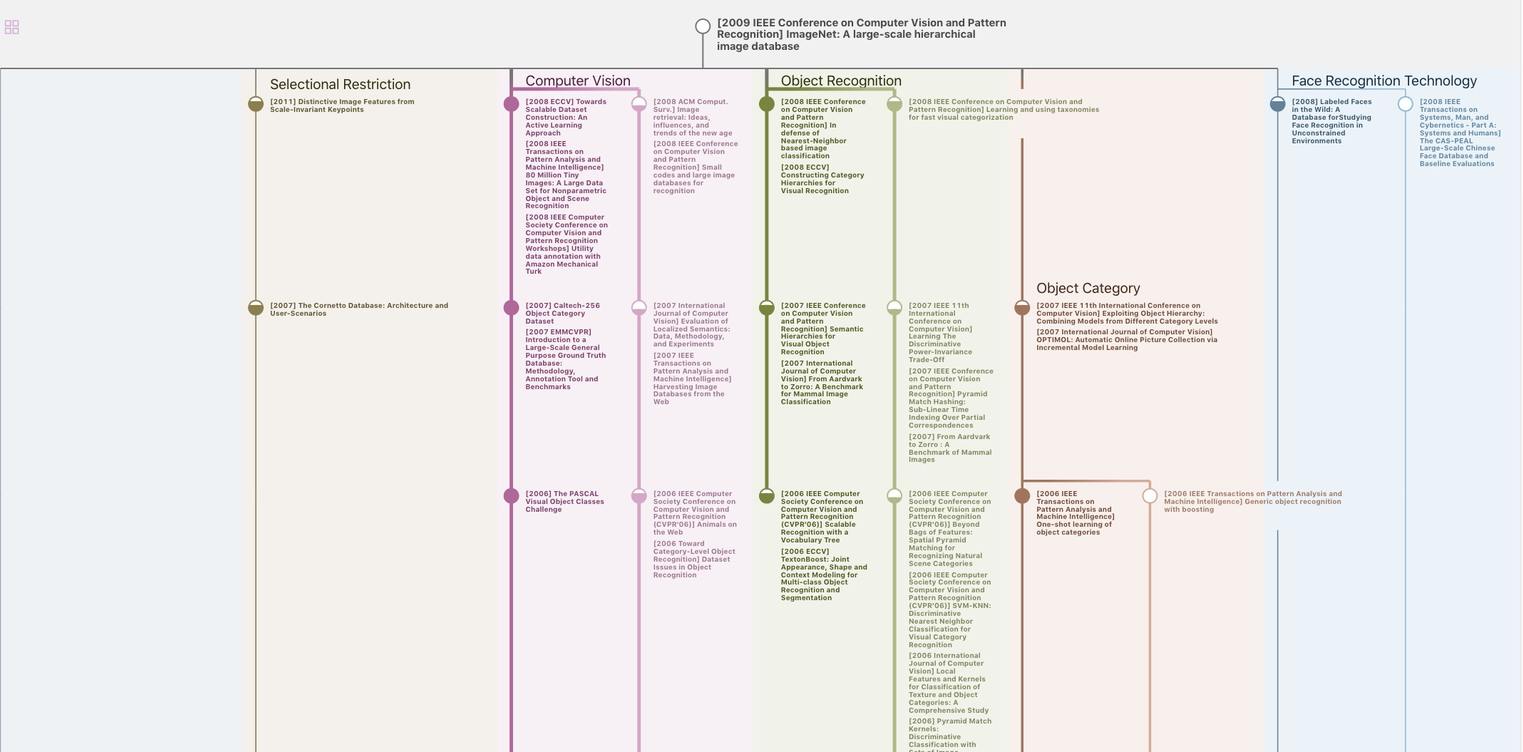
生成溯源树,研究论文发展脉络
Chat Paper
正在生成论文摘要