Sparse Bayesian modeling of hierarchical independent component analysis: Reliable estimation of individual differences in brain networks
Biometrics(2023)
摘要
Independent component analysis (ICA) is one of the leading approaches for studying brain functional networks. There is increasing interest in neuroscience studies to investigate individual differences in brain networks and their association with demographic characteristics and clinical outcomes. In this work, we develop a sparse Bayesian group hierarchical ICA model that offers significant improvements over existing ICA techniques for identifying covariate effects on the brain network. Specifically, we model the population-level ICA source signals for brain networks using a Dirichlet process mixture. To reliably capture individual differences on brain networks, we propose sparse estimation of the covariate effects in the hierarchical ICA model via a horseshoe prior. Through extensive simulation studies, we show that our approach performs considerably better in detecting covariate effects in comparison with the leading group ICA methods. We then perform an ICA decomposition of a between-subject meditation study. Our method is able to identify significant effects related to meditative practice in brain regions that are consistent with previous research into the default mode network, whereas other group ICA approaches find few to no effects.
更多查看译文
关键词
blind source separation,hierarchical independent component analysis,individual network differences,neuroimaging data analysis,reliable estimation of covariate effects on brain networks,sparse Bayesian ICA model
AI 理解论文
溯源树
样例
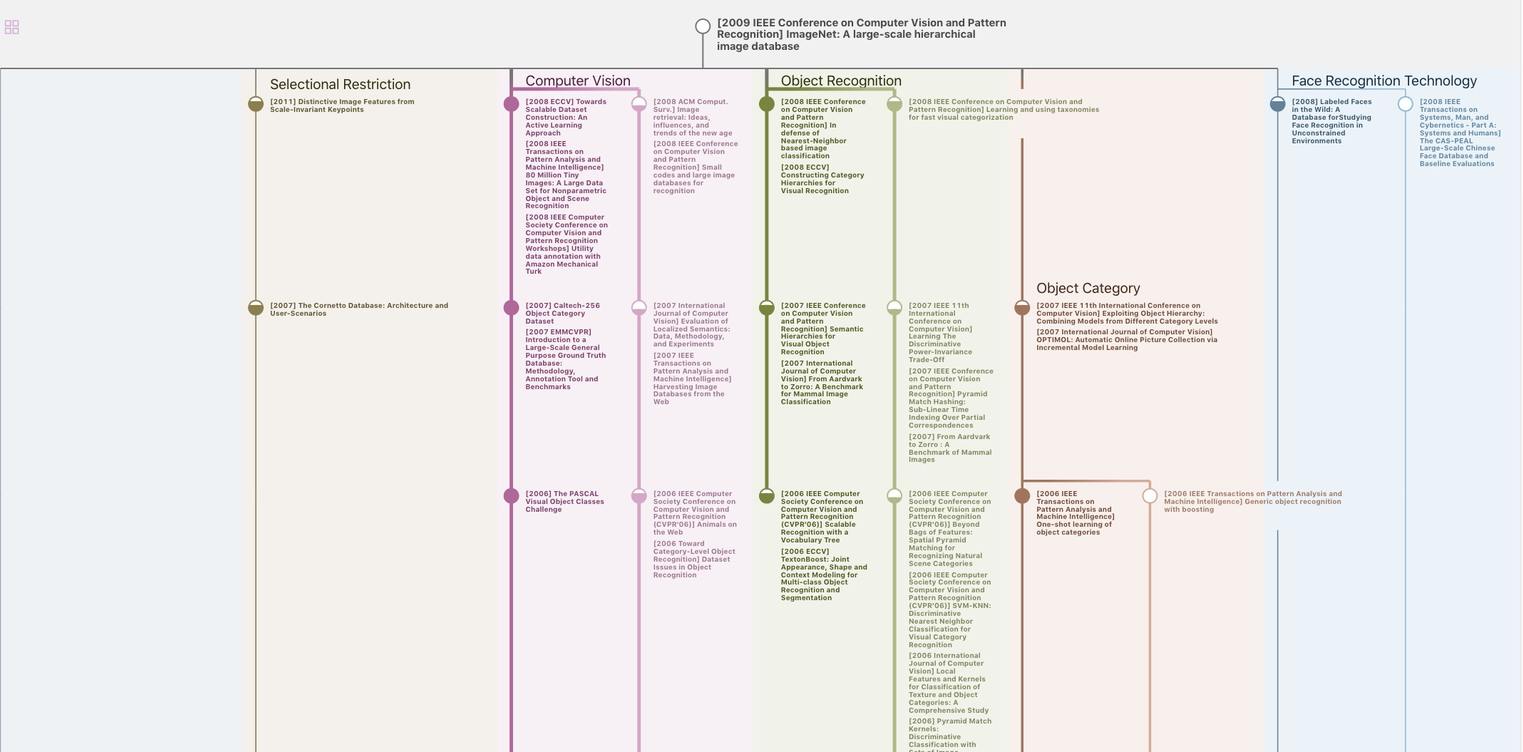
生成溯源树,研究论文发展脉络
Chat Paper
正在生成论文摘要